A new model for measuring global water storage
In their recent publication in Nature Water, D-BAUG researchers Junyang Gou and Professor Benedikt Soja introduced a finely resolved model of terrestrial water storage using a novel deep learning approach.
By integrating satellite observations with hydrological models, their method achieves remarkable accuracy even in smaller basins.
This model promises significant benefits across various domains, including hydrology, climate science, sustainable water management, and hazard prediction.
More information:
Junyang Gou et al, Global high-resolution total water storage anomalies from self-supervised data assimilation using deep learning algorithms, Nature Water (2024). DOI: 10.1038/s44221-024-00194-w
Coomentary: Alexander Sun, Learning to downscale satellite gravimetry data through artificial intelligence, Nature Water (2024). DOI: 10.1038/s44221-024-00199-5
Citation:
A new model for measuring global water storage (2024, February 19)
retrieved 19 February 2024
from https://phys.org/news/2024-02-global-storage.html
This document is subject to copyright. Apart from any fair dealing for the purpose of private study or research, no
part may be reproduced without the written permission. The content is provided for information purposes only.
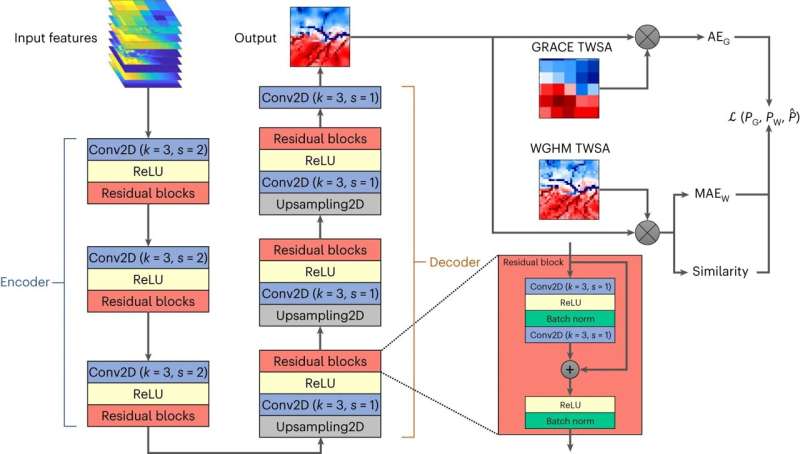
In their recent publication in Nature Water, D-BAUG researchers Junyang Gou and Professor Benedikt Soja introduced a finely resolved model of terrestrial water storage using a novel deep learning approach.
By integrating satellite observations with hydrological models, their method achieves remarkable accuracy even in smaller basins.
This model promises significant benefits across various domains, including hydrology, climate science, sustainable water management, and hazard prediction.
More information:
Junyang Gou et al, Global high-resolution total water storage anomalies from self-supervised data assimilation using deep learning algorithms, Nature Water (2024). DOI: 10.1038/s44221-024-00194-w
Coomentary: Alexander Sun, Learning to downscale satellite gravimetry data through artificial intelligence, Nature Water (2024). DOI: 10.1038/s44221-024-00199-5
Citation:
A new model for measuring global water storage (2024, February 19)
retrieved 19 February 2024
from https://phys.org/news/2024-02-global-storage.html
This document is subject to copyright. Apart from any fair dealing for the purpose of private study or research, no
part may be reproduced without the written permission. The content is provided for information purposes only.