Algorithms are everywhere | MIT Technology Review
“The present is not a prison sentence, but merely our current snapshot,” they write. “We don’t have to use unethical or opaque algorithmic decision systems, even in contexts where their use may be technically feasible. Ads based on mass surveillance are not necessary elements of our society. We don’t need to build systems that learn the stratifications of the past and present and reinforce them in the future. Privacy is not dead because of technology; it’s not true that the only way to support journalism or book writing or any craft that matters to you is spying on you to service ads. There are alternatives.”
A pressing need for regulation
If Wiggins and Jones’s goal was to reveal the intellectual tradition that underlies today’s algorithmic systems, including “the persistent role of data in rearranging power,” Josh Simons is more interested in how algorithmic power is exercised in a democracy and, more specifically, how we might go about regulating the corporations and institutions that wield it.
PRINCETON UNIVERSITY PRESS
Currently a research fellow in political theory at Harvard, Simons has a unique background. Not only did he work for four years at Facebook, where he was a founding member of what became the Responsible AI team, but he previously served as a policy advisor for the Labour Party in the UK Parliament.
In Algorithms for the People: Democracy in the Age of AI, Simons builds on the seminal work of authors like Cathy O’Neil, Safiya Noble, and Shoshana Zuboff to argue that algorithmic prediction is inherently political. “My aim is to explore how to make democracy work in the coming age of machine learning,” he writes. “Our future will be determined not by the nature of machine learning itself—machine learning models simply do what we tell them to do—but by our commitment to regulation that ensures that machine learning strengthens the foundations of democracy.”
Much of the first half of the book is dedicated to revealing all the ways we continue to misunderstand the nature of machine learning, and how its use can profoundly undermine democracy. And what if a “thriving democracy”—a term Simons uses throughout the book but never defines—isn’t always compatible with algorithmic governance? Well, it’s a question he never really addresses.
Whether these are blind spots or Simons simply believes that algorithmic prediction is, and will remain, an inevitable part of our lives, the lack of clarity doesn’t do the book any favors. While he’s on much firmer ground when explaining how machine learning works and deconstructing the systems behind Google’s PageRank and Facebook’s Feed, there remain omissions that don’t inspire confidence. For instance, it takes an uncomfortably long time for Simons to even acknowledge one of the key motivations behind the design of the PageRank and Feed algorithms: profit. Not something to overlook if you want to develop an effective regulatory framework.
“The ultimate, hidden truth of the world is that it is something that we make, and could just as easily make differently.”
Much of what’s discussed in the latter half of the book will be familiar to anyone following the news around platform and internet regulation (hint: that we should be treating providers more like public utilities). And while Simons has some creative and intelligent ideas, I suspect even the most ardent policy wonks will come away feeling a bit demoralized given the current state of politics in the United States.
In the end, the most hopeful message these books offer is embedded in the nature of algorithms themselves. In Filterworld, Chayka includes a quote from the late, great anthropologist David Graeber: “The ultimate, hidden truth of the world is that it is something that we make, and could just as easily make differently.” It’s a sentiment echoed in all three books—maybe minus the “easily” bit.
Algorithms may entrench our biases, homogenize and flatten culture, and exploit and suppress the vulnerable and marginalized. But these aren’t completely inscrutable systems or inevitable outcomes. They can do the opposite, too. Look closely at any machine-learning algorithm and you’ll inevitably find people—people making choices about which data to gather and how to weigh it, choices about design and target variables. And, yes, even choices about whether to use them at all. As long as algorithms are something humans make, we can also choose to make them differently.
Bryan Gardiner is a writer based in Oakland, California.
“The present is not a prison sentence, but merely our current snapshot,” they write. “We don’t have to use unethical or opaque algorithmic decision systems, even in contexts where their use may be technically feasible. Ads based on mass surveillance are not necessary elements of our society. We don’t need to build systems that learn the stratifications of the past and present and reinforce them in the future. Privacy is not dead because of technology; it’s not true that the only way to support journalism or book writing or any craft that matters to you is spying on you to service ads. There are alternatives.”
A pressing need for regulation
If Wiggins and Jones’s goal was to reveal the intellectual tradition that underlies today’s algorithmic systems, including “the persistent role of data in rearranging power,” Josh Simons is more interested in how algorithmic power is exercised in a democracy and, more specifically, how we might go about regulating the corporations and institutions that wield it.
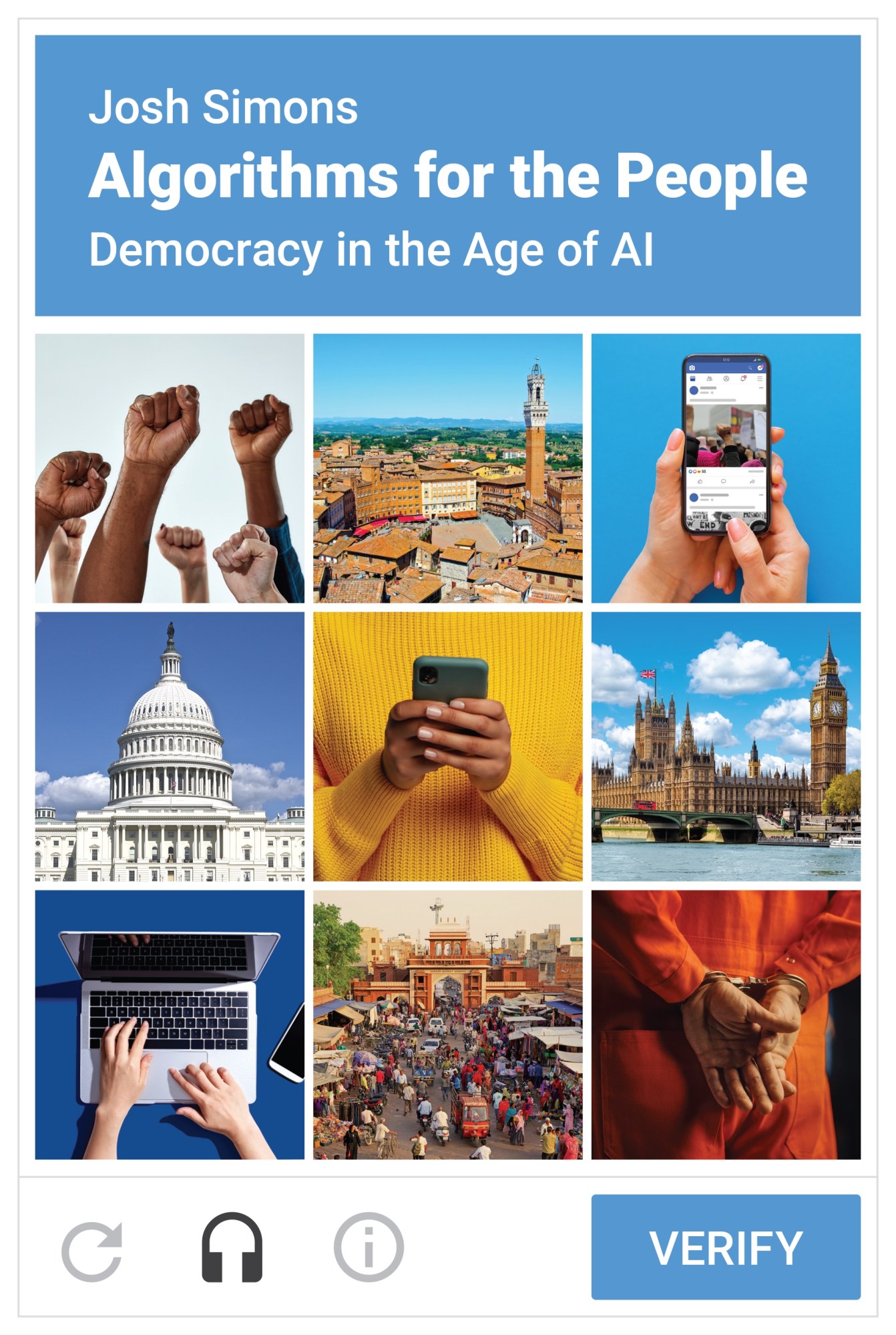
PRINCETON UNIVERSITY PRESS
Currently a research fellow in political theory at Harvard, Simons has a unique background. Not only did he work for four years at Facebook, where he was a founding member of what became the Responsible AI team, but he previously served as a policy advisor for the Labour Party in the UK Parliament.
In Algorithms for the People: Democracy in the Age of AI, Simons builds on the seminal work of authors like Cathy O’Neil, Safiya Noble, and Shoshana Zuboff to argue that algorithmic prediction is inherently political. “My aim is to explore how to make democracy work in the coming age of machine learning,” he writes. “Our future will be determined not by the nature of machine learning itself—machine learning models simply do what we tell them to do—but by our commitment to regulation that ensures that machine learning strengthens the foundations of democracy.”
Much of the first half of the book is dedicated to revealing all the ways we continue to misunderstand the nature of machine learning, and how its use can profoundly undermine democracy. And what if a “thriving democracy”—a term Simons uses throughout the book but never defines—isn’t always compatible with algorithmic governance? Well, it’s a question he never really addresses.
Whether these are blind spots or Simons simply believes that algorithmic prediction is, and will remain, an inevitable part of our lives, the lack of clarity doesn’t do the book any favors. While he’s on much firmer ground when explaining how machine learning works and deconstructing the systems behind Google’s PageRank and Facebook’s Feed, there remain omissions that don’t inspire confidence. For instance, it takes an uncomfortably long time for Simons to even acknowledge one of the key motivations behind the design of the PageRank and Feed algorithms: profit. Not something to overlook if you want to develop an effective regulatory framework.
“The ultimate, hidden truth of the world is that it is something that we make, and could just as easily make differently.”
Much of what’s discussed in the latter half of the book will be familiar to anyone following the news around platform and internet regulation (hint: that we should be treating providers more like public utilities). And while Simons has some creative and intelligent ideas, I suspect even the most ardent policy wonks will come away feeling a bit demoralized given the current state of politics in the United States.
In the end, the most hopeful message these books offer is embedded in the nature of algorithms themselves. In Filterworld, Chayka includes a quote from the late, great anthropologist David Graeber: “The ultimate, hidden truth of the world is that it is something that we make, and could just as easily make differently.” It’s a sentiment echoed in all three books—maybe minus the “easily” bit.
Algorithms may entrench our biases, homogenize and flatten culture, and exploit and suppress the vulnerable and marginalized. But these aren’t completely inscrutable systems or inevitable outcomes. They can do the opposite, too. Look closely at any machine-learning algorithm and you’ll inevitably find people—people making choices about which data to gather and how to weigh it, choices about design and target variables. And, yes, even choices about whether to use them at all. As long as algorithms are something humans make, we can also choose to make them differently.
Bryan Gardiner is a writer based in Oakland, California.