Harnessing Artificial Intelligence for a Cutting-Edge Tsunami Early Warning System
Researchers have developed an early warning system for tsunamis that combines state-of-the-art acoustic technology with artificial intelligence. This system measures the acoustic radiation produced by underwater earthquakes, which travels faster than tsunami waves and carries information about the tectonic event. By using hydrophones to record acoustic waves, the system can monitor tectonic activity in real-time. A computational model then triangulates the source of the earthquake, and AI algorithms classify its slip type and magnitude to determine potential tsunami risk. This method allows for faster and more accurate assessments, reducing false alarms and enhancing the reliability of warning systems.
Real-time classification of underwater earthquakes based on acoustic signals enables earlier, more reliable disaster preparation.
Researchers have developed an AI-powered early warning system for tsunamis that uses acoustic technology and hydrophones to measure and classify underwater earthquakes in real-time, allowing for faster and more accurate risk assessments.
Tsunamis are incredibly destructive waves that can destroy coastal infrastructure and cause loss of life. Early warnings for such natural disasters are difficult because the risk of a tsunami is highly dependent on the features of the underwater earthquake that triggers it.
In Physics of Fluids, by AIP Publishing, researchers from the University of California, Los Angeles (UCLA), and Cardiff University in the U.K. developed an early warning system that combines state-of-the-art acoustic technology with artificial intelligence to immediately classify earthquakes and determine potential tsunami risk.
Underwater earthquakes can trigger tsunamis if a large amount of water is displaced, so determining the type of earthquake is critical to assessing the tsunami risk.
“Tectonic events with a strong vertical slip element are more likely to raise or lower the water column compared to horizontal slip elements,” said co-author Bernabe Gomez. “Thus, knowing the slip type at the early stages of the assessment can reduce false alarms and enhance the reliability of the warning systems through independent cross-validation.”

This study investigates four different past earthquake scenarios associated with tsunami events. The red and yellow rectangles represent the projected earthquake dimensions, locations, and orientations retrieved by the proposed inverse model for acoustic radiation. The analyzed earthquakes are: a) Sept. 29, 2009, Mw 8.1, SSW of Matavai, Samoa; b) Dec. 21, 2010, Mw 7.4, Bonin Islands, Japan region; c) March 14, 2012, Mw 6.9, SSE of Kushiro, Japan; and d) Oct. 25, 2013, Mw 7.1, off the east coast of Honshu, Japan. The model delivers two potential fault orientations for each earthquake scenario, which are numerically modeled and compared. Credit: Bernabe Gomez and Usama Kadri
In these cases, time is of the essence, and relying on deep ocean wave buoys to measure water levels often leaves insufficient evacuation time. Instead, the researchers propose measuring the acoustic radiation (sound) produced by the earthquake, which carries information about the tectonic event and travels significantly faster than tsunami waves. Underwater microphones, called hydrophones, record the acoustic waves and monitor tectonic activity in real-time.
“Acoustic radiation travels through the water column much faster than tsunami waves. It carries information about the originating source and its pressure field can be recorded at distant locations, even thousands of kilometers away from the source. The derivation of analytical solutions for the pressure field is a key factor in the real-time analysis,” co-author Usama Kadri said.
The computational model triangulates the source of the earthquake from the hydrophones and AI algorithms classify its slip type and magnitude. It then calculates important properties like effective length and width, uplift speed, and duration, which dictate the size of the tsunami.
The authors tested their model with available hydrophone data and discovered that it almost instantaneously and successfully described the earthquake parameters with low computational demand. They are improving the model by factoring in more information to increase the tsunami characterization’s accuracy.
Their work predicting tsunami risk is part of a larger project to enhance hazard warning systems. The tsunami classification is a back-end aspect of a software that can improve the safety of offshore platforms and ships.
Reference: “Numerical validation of an effective slender fault source solution for past tsunami scenarios” by Bernabe Gomez and Usama Kadri, 27 April 2023, Physics of Fluids.
DOI: 10.1063/5.0144360
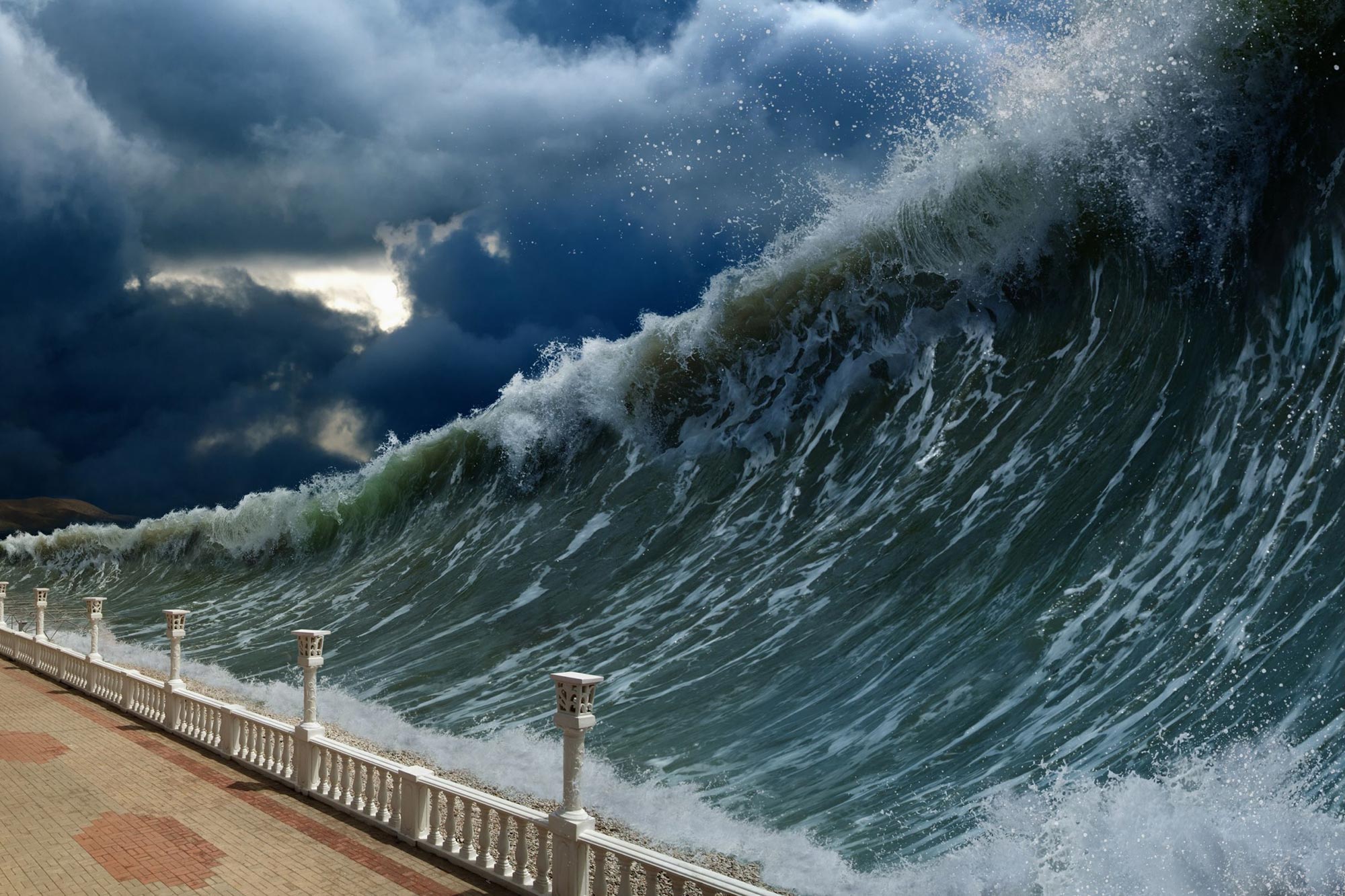
Researchers have developed an early warning system for tsunamis that combines state-of-the-art acoustic technology with artificial intelligence. This system measures the acoustic radiation produced by underwater earthquakes, which travels faster than tsunami waves and carries information about the tectonic event. By using hydrophones to record acoustic waves, the system can monitor tectonic activity in real-time. A computational model then triangulates the source of the earthquake, and AI algorithms classify its slip type and magnitude to determine potential tsunami risk. This method allows for faster and more accurate assessments, reducing false alarms and enhancing the reliability of warning systems.
Real-time classification of underwater earthquakes based on acoustic signals enables earlier, more reliable disaster preparation.
Researchers have developed an AI-powered early warning system for tsunamis that uses acoustic technology and hydrophones to measure and classify underwater earthquakes in real-time, allowing for faster and more accurate risk assessments.
Tsunamis are incredibly destructive waves that can destroy coastal infrastructure and cause loss of life. Early warnings for such natural disasters are difficult because the risk of a tsunami is highly dependent on the features of the underwater earthquake that triggers it.
In Physics of Fluids, by AIP Publishing, researchers from the University of California, Los Angeles (UCLA), and Cardiff University in the U.K. developed an early warning system that combines state-of-the-art acoustic technology with artificial intelligence to immediately classify earthquakes and determine potential tsunami risk.
Underwater earthquakes can trigger tsunamis if a large amount of water is displaced, so determining the type of earthquake is critical to assessing the tsunami risk.
“Tectonic events with a strong vertical slip element are more likely to raise or lower the water column compared to horizontal slip elements,” said co-author Bernabe Gomez. “Thus, knowing the slip type at the early stages of the assessment can reduce false alarms and enhance the reliability of the warning systems through independent cross-validation.”

This study investigates four different past earthquake scenarios associated with tsunami events. The red and yellow rectangles represent the projected earthquake dimensions, locations, and orientations retrieved by the proposed inverse model for acoustic radiation. The analyzed earthquakes are: a) Sept. 29, 2009, Mw 8.1, SSW of Matavai, Samoa; b) Dec. 21, 2010, Mw 7.4, Bonin Islands, Japan region; c) March 14, 2012, Mw 6.9, SSE of Kushiro, Japan; and d) Oct. 25, 2013, Mw 7.1, off the east coast of Honshu, Japan. The model delivers two potential fault orientations for each earthquake scenario, which are numerically modeled and compared. Credit: Bernabe Gomez and Usama Kadri
In these cases, time is of the essence, and relying on deep ocean wave buoys to measure water levels often leaves insufficient evacuation time. Instead, the researchers propose measuring the acoustic radiation (sound) produced by the earthquake, which carries information about the tectonic event and travels significantly faster than tsunami waves. Underwater microphones, called hydrophones, record the acoustic waves and monitor tectonic activity in real-time.
“Acoustic radiation travels through the water column much faster than tsunami waves. It carries information about the originating source and its pressure field can be recorded at distant locations, even thousands of kilometers away from the source. The derivation of analytical solutions for the pressure field is a key factor in the real-time analysis,” co-author Usama Kadri said.
The computational model triangulates the source of the earthquake from the hydrophones and AI algorithms classify its slip type and magnitude. It then calculates important properties like effective length and width, uplift speed, and duration, which dictate the size of the tsunami.
The authors tested their model with available hydrophone data and discovered that it almost instantaneously and successfully described the earthquake parameters with low computational demand. They are improving the model by factoring in more information to increase the tsunami characterization’s accuracy.
Their work predicting tsunami risk is part of a larger project to enhance hazard warning systems. The tsunami classification is a back-end aspect of a software that can improve the safety of offshore platforms and ships.
Reference: “Numerical validation of an effective slender fault source solution for past tsunami scenarios” by Bernabe Gomez and Usama Kadri, 27 April 2023, Physics of Fluids.
DOI: 10.1063/5.0144360