Improved estimates of carbon sinks and sources of northern ecosystems
A new study shows that a calculation method used by international measurement networks creates a systematic bias in carbon balance estimates for northern ecosystems. The study, led by the Finnish Meteorological Institute, shows that a machine learning method can be used to substantially reduce the error.
A key method used to measure carbon balances of forests, agricultural fields and wetlands is the eddy covariance technique. In principle, this method measures carbon dioxide exchange between ecosystems and the atmosphere continuously every half an hour. However, some of the measurements have to be discarded because of unfavorable atmospheric conditions, and also technical failures lead to data gaps. Replacing, or gap-filling, the missing data to obtain full timeseries is an essential step in calculating annual carbon balances.
A new study, which was led by the Finnish Meteorological Institute, investigated for the first time what is the impact of different gap-filling methods on the carbon balance estimates of high-latitude ecosystems. The results were published in Scientific Reports.
The study showed that the most common gap-filling method, also used by international flux measurement networks, causes a systematic error in the annual carbon balance estimates of northern ecosystems. Because of this error, emissions of carbon sources have been overestimated and the strength of carbon sinks underestimated. The bias is substantial, at some sites even of a similar magnitude to the annual carbon balance. The error is caused by a very skewed distribution of solar radiation in northern latitudes.
A machine-learning method produces reliable carbon balance estimates
To correct for the error, the study utilized a machine learning method, which proved to be more accurate than the old standard method and did not cause any systematic errors. In addition to a new, unbiased method, the old method was modified to better account for the environmental conditions prevailing in northern latitudes.
Boreal and tundra biomes cover an area of more than 20 million square kilometers. Therefore, the systematic error found in the study can have significant impacts on the carbon balance estimates of northern areas, if these are based on eddy covariance measurements. When the improved gap-filling methods are taken widely into use, estimates of carbon balances of northern ecosystems can be calculated more accurately. In the future, eddy covariance data can also be utilized to increase the reliability of carbon balance estimates produced in national greenhouse gas inventories.
More information:
Henriikka Vekuri et al, A widely-used eddy covariance gap-filling method creates systematic bias in carbon balance estimates, Scientific Reports (2023). DOI: 10.1038/s41598-023-28827-2
Provided by
Finnish Meteorological Institute
Citation:
Improved estimates of carbon sinks and sources of northern ecosystems (2023, February 3)
retrieved 3 February 2023
from https://phys.org/news/2023-02-carbon-sources-northern-ecosystems.html
This document is subject to copyright. Apart from any fair dealing for the purpose of private study or research, no
part may be reproduced without the written permission. The content is provided for information purposes only.
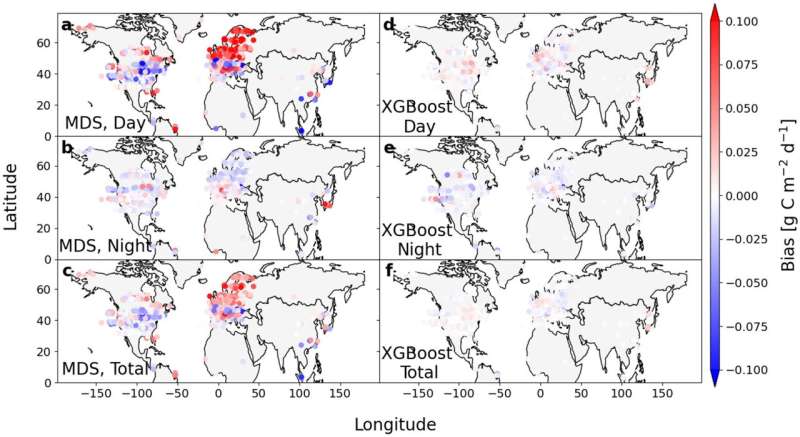
A new study shows that a calculation method used by international measurement networks creates a systematic bias in carbon balance estimates for northern ecosystems. The study, led by the Finnish Meteorological Institute, shows that a machine learning method can be used to substantially reduce the error.
A key method used to measure carbon balances of forests, agricultural fields and wetlands is the eddy covariance technique. In principle, this method measures carbon dioxide exchange between ecosystems and the atmosphere continuously every half an hour. However, some of the measurements have to be discarded because of unfavorable atmospheric conditions, and also technical failures lead to data gaps. Replacing, or gap-filling, the missing data to obtain full timeseries is an essential step in calculating annual carbon balances.
A new study, which was led by the Finnish Meteorological Institute, investigated for the first time what is the impact of different gap-filling methods on the carbon balance estimates of high-latitude ecosystems. The results were published in Scientific Reports.
The study showed that the most common gap-filling method, also used by international flux measurement networks, causes a systematic error in the annual carbon balance estimates of northern ecosystems. Because of this error, emissions of carbon sources have been overestimated and the strength of carbon sinks underestimated. The bias is substantial, at some sites even of a similar magnitude to the annual carbon balance. The error is caused by a very skewed distribution of solar radiation in northern latitudes.
A machine-learning method produces reliable carbon balance estimates
To correct for the error, the study utilized a machine learning method, which proved to be more accurate than the old standard method and did not cause any systematic errors. In addition to a new, unbiased method, the old method was modified to better account for the environmental conditions prevailing in northern latitudes.
Boreal and tundra biomes cover an area of more than 20 million square kilometers. Therefore, the systematic error found in the study can have significant impacts on the carbon balance estimates of northern areas, if these are based on eddy covariance measurements. When the improved gap-filling methods are taken widely into use, estimates of carbon balances of northern ecosystems can be calculated more accurately. In the future, eddy covariance data can also be utilized to increase the reliability of carbon balance estimates produced in national greenhouse gas inventories.
More information:
Henriikka Vekuri et al, A widely-used eddy covariance gap-filling method creates systematic bias in carbon balance estimates, Scientific Reports (2023). DOI: 10.1038/s41598-023-28827-2
Provided by
Finnish Meteorological Institute
Citation:
Improved estimates of carbon sinks and sources of northern ecosystems (2023, February 3)
retrieved 3 February 2023
from https://phys.org/news/2023-02-carbon-sources-northern-ecosystems.html
This document is subject to copyright. Apart from any fair dealing for the purpose of private study or research, no
part may be reproduced without the written permission. The content is provided for information purposes only.