Scientists propose machine learning approach to solve the world’s food insecurity issue
Researchers of a recent paper published by Nature Food suggest a method which they claim will allow decision-makers to make more timely and informed decisions on policies and programs oriented towards the fight against hunger.
In 2021, 193 million people across 53 countries were acutely food insecure. This number has been steadily increasing during the last few years also as a consequence of the COVID-19 pandemic. To address this global issue, monitoring the situation and its evolution is key.
Governments and humanitarian organizations perform food security assessments on a regular basis through face-to-face and remote mobile phone surveys. However, these approaches have high costs in both monetary and human resources, and hence primary data on the food security situation is not always available for all affected areas. Yet this information is key for governments and humanitarian organizations.
To tackle this issue, researchers of the Nature Food paper propose a machine learning approach to predict the number of people with insufficient food consumption when up-to-date direct measurements are not available. “We also propose a method to identify which variables are driving the changes observed in predicted trends, which is key to making predictions serviceable to decision-makers,” says Assistant Professor Elisa Omodei (Department of Network and Data Science, CEU, Vienna).
The proposed method uses a machine learning algorithm to estimate the current food insecurity situation in a given area from data on the key drivers of food insecurity: conflict, weather extremes and economic shocks. The results show that the proposed methodology can explain up to 81% of the variation in insufficient food consumption.
Researchers claim their approach opens the door to food security near-real time nowcasting on a global scale, allowing decision-makers to make more timely and informed decisions on policies and programs oriented towards the fight against hunger, in the effort to try to achieve SDG 2 of the 2030 Agenda for Sustainable Development.
Hunger is increasing worldwide, but women bear the brunt of food insecurity
Giulia Martini et al, Machine learning can guide food security efforts when primary data are not available, Nature Food (2022). DOI: 10.1038/s43016-022-00587-8
Provided by
Central European University
Citation:
Scientists propose machine learning approach to solve the world’s food insecurity issue (2022, September 19)
retrieved 19 September 2022
from https://phys.org/news/2022-09-scientists-machine-approach-world-food.html
This document is subject to copyright. Apart from any fair dealing for the purpose of private study or research, no
part may be reproduced without the written permission. The content is provided for information purposes only.
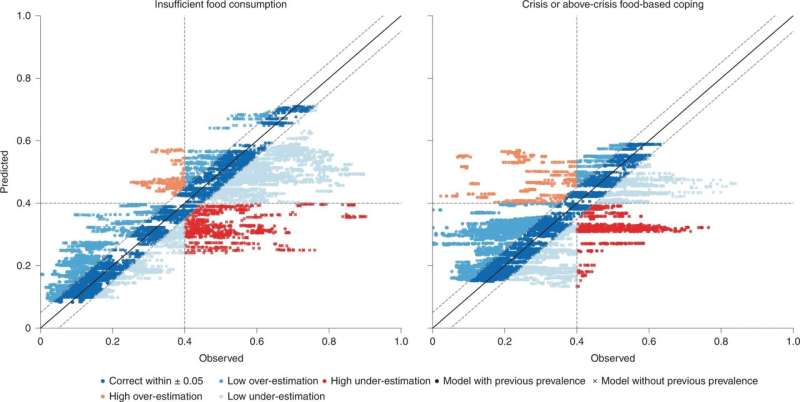
Researchers of a recent paper published by Nature Food suggest a method which they claim will allow decision-makers to make more timely and informed decisions on policies and programs oriented towards the fight against hunger.
In 2021, 193 million people across 53 countries were acutely food insecure. This number has been steadily increasing during the last few years also as a consequence of the COVID-19 pandemic. To address this global issue, monitoring the situation and its evolution is key.
Governments and humanitarian organizations perform food security assessments on a regular basis through face-to-face and remote mobile phone surveys. However, these approaches have high costs in both monetary and human resources, and hence primary data on the food security situation is not always available for all affected areas. Yet this information is key for governments and humanitarian organizations.
To tackle this issue, researchers of the Nature Food paper propose a machine learning approach to predict the number of people with insufficient food consumption when up-to-date direct measurements are not available. “We also propose a method to identify which variables are driving the changes observed in predicted trends, which is key to making predictions serviceable to decision-makers,” says Assistant Professor Elisa Omodei (Department of Network and Data Science, CEU, Vienna).
The proposed method uses a machine learning algorithm to estimate the current food insecurity situation in a given area from data on the key drivers of food insecurity: conflict, weather extremes and economic shocks. The results show that the proposed methodology can explain up to 81% of the variation in insufficient food consumption.
Researchers claim their approach opens the door to food security near-real time nowcasting on a global scale, allowing decision-makers to make more timely and informed decisions on policies and programs oriented towards the fight against hunger, in the effort to try to achieve SDG 2 of the 2030 Agenda for Sustainable Development.
Hunger is increasing worldwide, but women bear the brunt of food insecurity
Giulia Martini et al, Machine learning can guide food security efforts when primary data are not available, Nature Food (2022). DOI: 10.1038/s43016-022-00587-8
Provided by
Central European University
Citation:
Scientists propose machine learning approach to solve the world’s food insecurity issue (2022, September 19)
retrieved 19 September 2022
from https://phys.org/news/2022-09-scientists-machine-approach-world-food.html
This document is subject to copyright. Apart from any fair dealing for the purpose of private study or research, no
part may be reproduced without the written permission. The content is provided for information purposes only.