10 Generative AI Finance Use Cases in 2023
Autonomous finance refers to the use of advanced technologies, such as artificial intelligence (AI) and hyperautomation, to automate financial processes, decision-making, and services. Financial institutions are increasingly adopting autonomous finance due to its potential to enhance efficiency, reduce operational costs, and improve customer experiences.
To boost autonomous finance, the financial services industry can leverage generative AI technologies. In this article, we are explaining top 10 generative AI finance use cases by providing some real life examples.
Generative AI Finance Use Cases
The financial services industry is already on its way to adopt the generative AI models for certain financial tasks.
For instance, Morgan Stanley employs OpenAI-powered chatbots to support financial advisors by utilizing the company’s internal collection of research and data as a knowledge resource. More interestingly, Bloomberg announced its finance fine-tuned generative model BloombergGPT, which is capable of making sentiment analysis, news classification and some other financial tasks, successfully passing the benchmarks.
Figure 1. How BloombergGPT performs across two broad categories of NLP tasks: finance-specific and general-purpose
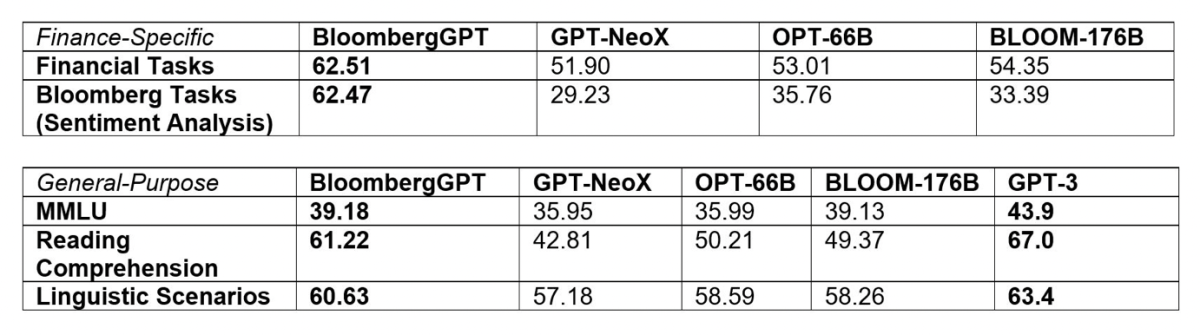
Source: Bloomberg
However, generative AI has a bigger potential to streamline many financial tasks if adopted adequately. Here are the top 10 potential use cases:
1- Conversational finance
Generative AI is a class of AI models that can generate new data by learning patterns from existing data, and generate human-like text based on the input provided. Conversational AI specifically focuses on simulating human-like conversations through AI-powered chatbots or virtual assistants, by using natural language processing (NLP), natural language understanding (NLU) and natural language generation (NLG).
In the context of conversational finance, generative AI models can be used to produce more natural and contextually relevant responses, as they are trained to understand and generate human-like language patterns. As a result, generative AI can significantly enhance the performance and user experience of financial conversational AI systems by providing more accurate, engaging, and nuanced interactions with users.
Conversational finance provides customers with:
- Improved customer support
- Personalized financial advice
- Payment notifications
For more on conversational finance, you can check our article on the use cases of conversational AI in the financial services industry.
Also, for the wide range of use cases of conversational AI for customer service operations, check our conversational AI for customer service article.
2- Document analysis
Generative AI can be used to process, summarize, and extract valuable information from large volumes of financial documents, such as annual reports, financial statements, and earnings calls, facilitating more efficient analysis and decision-making.
3- Financial analysis and forecasting
By learning from historical financial data, generative AI models can capture complex patterns and relationships in the data, enabling them to make predictive analytics about future trends, asset prices, and economic indicators.
Generative AI models, when fine-tuned properly, can generate various scenarios by simulating market conditions, macroeconomic factors, and other variables, providing valuable insights into potential risks and opportunities.
4- Financial question answering
By leveraging its understanding of human language patterns and its ability to generate coherent, contextually relevant responses, generative AI can provide accurate and detailed answers to financial questions posed by users.
These models can be trained on large datasets of financial knowledge to respond to a wide range of financial queries with appropriate information, including topics like:
- Accounting principles
- Financial ratios
- Stock analysis
- Regulatory compliance
For example, BloombergGPT can accurately respond to some finance related questions compared to other generative models.
Figure 2. The ability of BloombergGPT, GPT-NeoX, and FLAN-T5-XXL to re- call the names of CEOs of companies
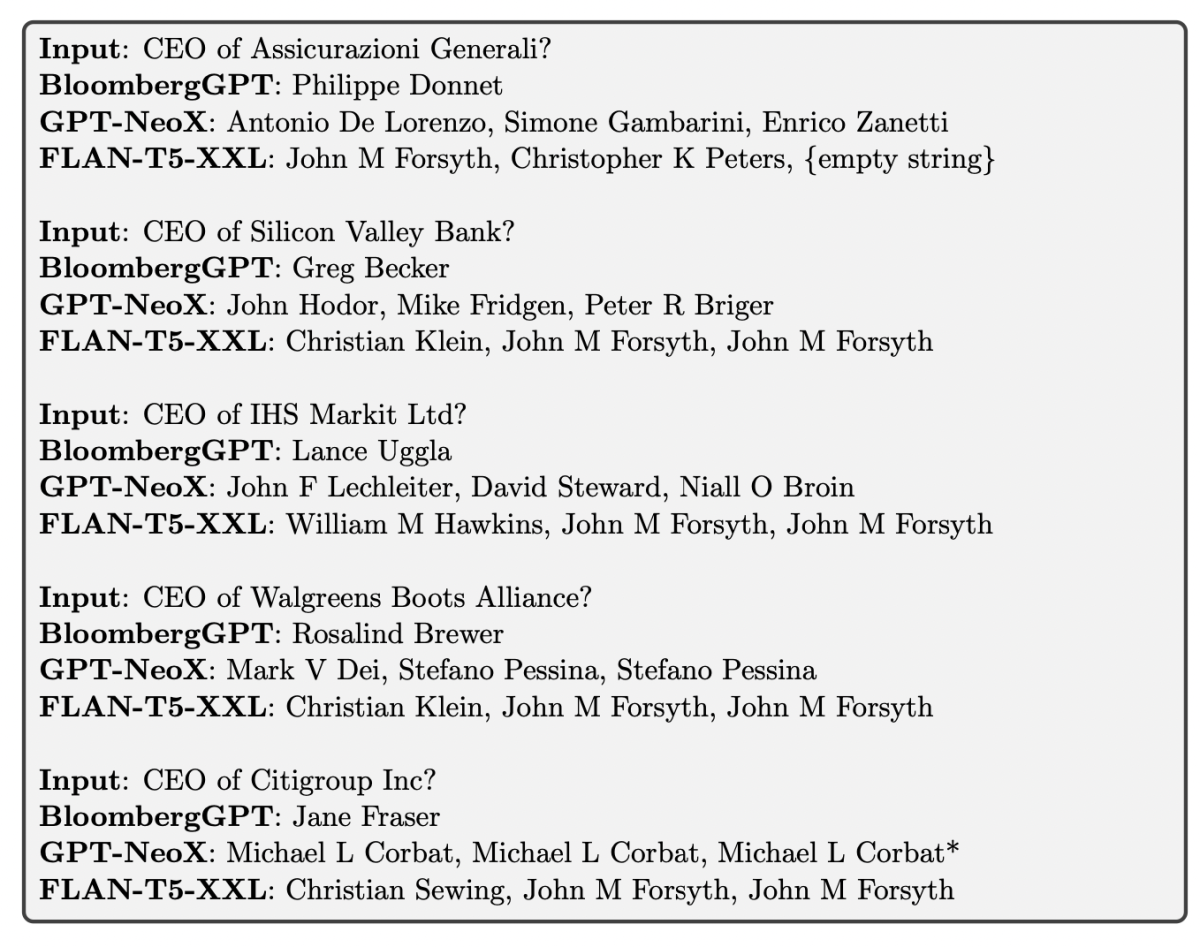
Source: “BloombergGPT: A Large Language Model for Finance”
5- Financial report generation
Generative AI can automatically create well-structured, coherent, and informative financial reports based on available data. These reports may include:
- Balance sheets
- Income statements
- Cash flow statements
This automation not only streamlines the reporting process and reduces manual effort, but it also ensures consistency, accuracy, and timely delivery of reports.
Moreover, generative AI models can be used to generate customized financial reports or visualizations tailored to specific user needs, making them even more valuable for businesses and financial professionals.
6- Fraud detection
Generative AI can be used for fraud detection in finance by generating synthetic examples of fraudulent transactions or activities. These generated examples can help train and augment machine learning algorithms to recognize and differentiate between legitimate and fraudulent patterns in financial data.
The enhanced understanding of fraud patterns allows these models to identify suspicious activities more accurately and effectively, leading to faster detection and prevention of fraud. By incorporating generative AI in fraud detection systems, financial institutions can:
- Improve the overall security and integrity of their operations
- Minimize losses due to fraud
- Maintain consumer trust
7- Generating applicant-friendly denial explanations
AI plays a significant role in the banking sector, particularly in loan decision-making processes. It helps banks and financial institutions assess customers’ creditworthiness, determine appropriate credit limits, and set loan pricing based on risk. However, both decision-makers and loan applicants need clear explanations of AI-based decisions, such as reasons for application denials, to foster trust and improve customer awareness for future applications.
A conditional generative adversarial network (GAN), a generative AI variant, was used to generate user-friendly denial explanations. By organizing denial reasons hierarchically from simple to complex, two-level conditioning is employed to generate more understandable explanations for applicants (Figure 3).
Figure 3. AI generated loan denial explanations
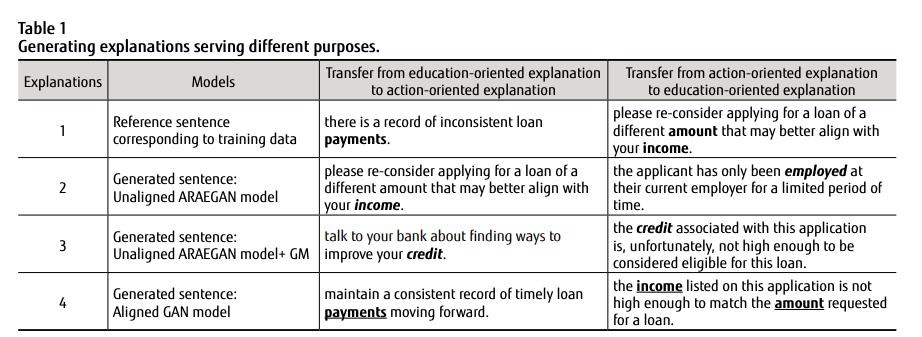
Source: “Generating User-Friendly Explanations for Loan Denials Using Generative Adversarial Networks”
8- Portfolio management and risk management
Another financial application of generative AI can be portfolio optimization. By analyzing historical financial data and generating various investment scenarios, generative AI models can help asset managers and investors identify optimal asset and wealth management, taking into account factors such as:
- Risk tolerance
- Expected returns
- Investment horizons
These models can simulate different market conditions, economic environments, and events to better understand the potential impacts on portfolio performance. This allows financial professionals to develop and fine-tune their investment strategies, optimize risk-adjusted returns, and make more informed decisions about managing their portfolios. This ultimately leads to improved financial outcomes for their clients or institutions.
9- Synthetic data generation
Since customer information is proprietary data for finance teams, it introduces some problems in terms of its use and regulation. Generative AI can be employed by financial institutions to produce synthetic data that adheres to privacy regulations such as GDPR and CCPA. By learning patterns and relationships from real financial data, generative AI models are able to create synthetic datasets that closely resemble the original data while preserving data privacy.
These synthetic datasets can be used for various purposes by financial institutions without exposing sensitive customer information, such as:
- Training machine learning models
- Conducting stress tests
- Validating models
For more on synthetic data, you can check our articles comparing synthetic data and real data, or comparing synthetic data and data masking methods for data privacy.
10- Sentiment analysis
Sentiment analysis, an approach within NLP, categorizes texts, images, or videos according to their emotional tone as negative, positive, or neutral. By gaining insights into customers’ emotions and opinions, companies can devise strategies to enhance their services or products based on these findings.
Financial institutions also benefit from sentiment analysis to measure their brand reputation and customer satisfaction through social media posts, news articles, or other sources.
Check out our article on stock market sentiment analysis to learn more.
For example, BloombergGPT was also evaluated in the sentiment analysis task. As a fine-tuned generative model for finance, it outperformed other models by succeeding in sentiment analysis.
If you have questions or need help in finding the right vendors, we can help:
Autonomous finance refers to the use of advanced technologies, such as artificial intelligence (AI) and hyperautomation, to automate financial processes, decision-making, and services. Financial institutions are increasingly adopting autonomous finance due to its potential to enhance efficiency, reduce operational costs, and improve customer experiences.
To boost autonomous finance, the financial services industry can leverage generative AI technologies. In this article, we are explaining top 10 generative AI finance use cases by providing some real life examples.
Generative AI Finance Use Cases
The financial services industry is already on its way to adopt the generative AI models for certain financial tasks.
For instance, Morgan Stanley employs OpenAI-powered chatbots to support financial advisors by utilizing the company’s internal collection of research and data as a knowledge resource. More interestingly, Bloomberg announced its finance fine-tuned generative model BloombergGPT, which is capable of making sentiment analysis, news classification and some other financial tasks, successfully passing the benchmarks.
Figure 1. How BloombergGPT performs across two broad categories of NLP tasks: finance-specific and general-purpose
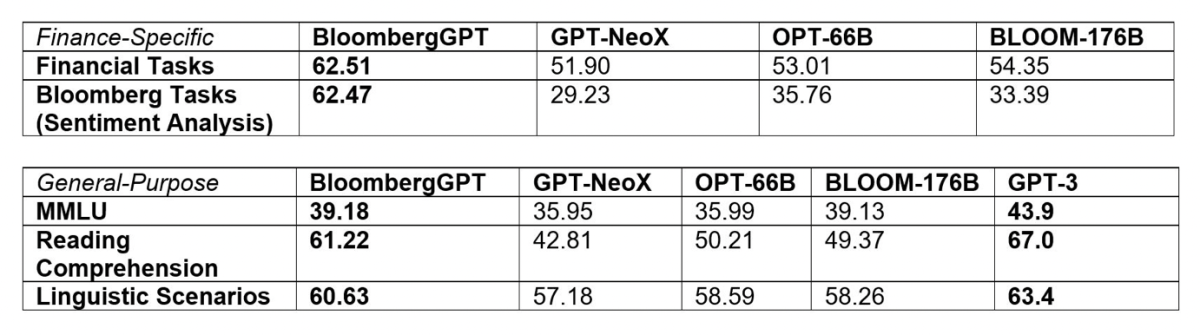
Source: Bloomberg
However, generative AI has a bigger potential to streamline many financial tasks if adopted adequately. Here are the top 10 potential use cases:
1- Conversational finance
Generative AI is a class of AI models that can generate new data by learning patterns from existing data, and generate human-like text based on the input provided. Conversational AI specifically focuses on simulating human-like conversations through AI-powered chatbots or virtual assistants, by using natural language processing (NLP), natural language understanding (NLU) and natural language generation (NLG).
In the context of conversational finance, generative AI models can be used to produce more natural and contextually relevant responses, as they are trained to understand and generate human-like language patterns. As a result, generative AI can significantly enhance the performance and user experience of financial conversational AI systems by providing more accurate, engaging, and nuanced interactions with users.
Conversational finance provides customers with:
- Improved customer support
- Personalized financial advice
- Payment notifications
For more on conversational finance, you can check our article on the use cases of conversational AI in the financial services industry.
Also, for the wide range of use cases of conversational AI for customer service operations, check our conversational AI for customer service article.
2- Document analysis
Generative AI can be used to process, summarize, and extract valuable information from large volumes of financial documents, such as annual reports, financial statements, and earnings calls, facilitating more efficient analysis and decision-making.
3- Financial analysis and forecasting
By learning from historical financial data, generative AI models can capture complex patterns and relationships in the data, enabling them to make predictive analytics about future trends, asset prices, and economic indicators.
Generative AI models, when fine-tuned properly, can generate various scenarios by simulating market conditions, macroeconomic factors, and other variables, providing valuable insights into potential risks and opportunities.
4- Financial question answering
By leveraging its understanding of human language patterns and its ability to generate coherent, contextually relevant responses, generative AI can provide accurate and detailed answers to financial questions posed by users.
These models can be trained on large datasets of financial knowledge to respond to a wide range of financial queries with appropriate information, including topics like:
- Accounting principles
- Financial ratios
- Stock analysis
- Regulatory compliance
For example, BloombergGPT can accurately respond to some finance related questions compared to other generative models.
Figure 2. The ability of BloombergGPT, GPT-NeoX, and FLAN-T5-XXL to re- call the names of CEOs of companies
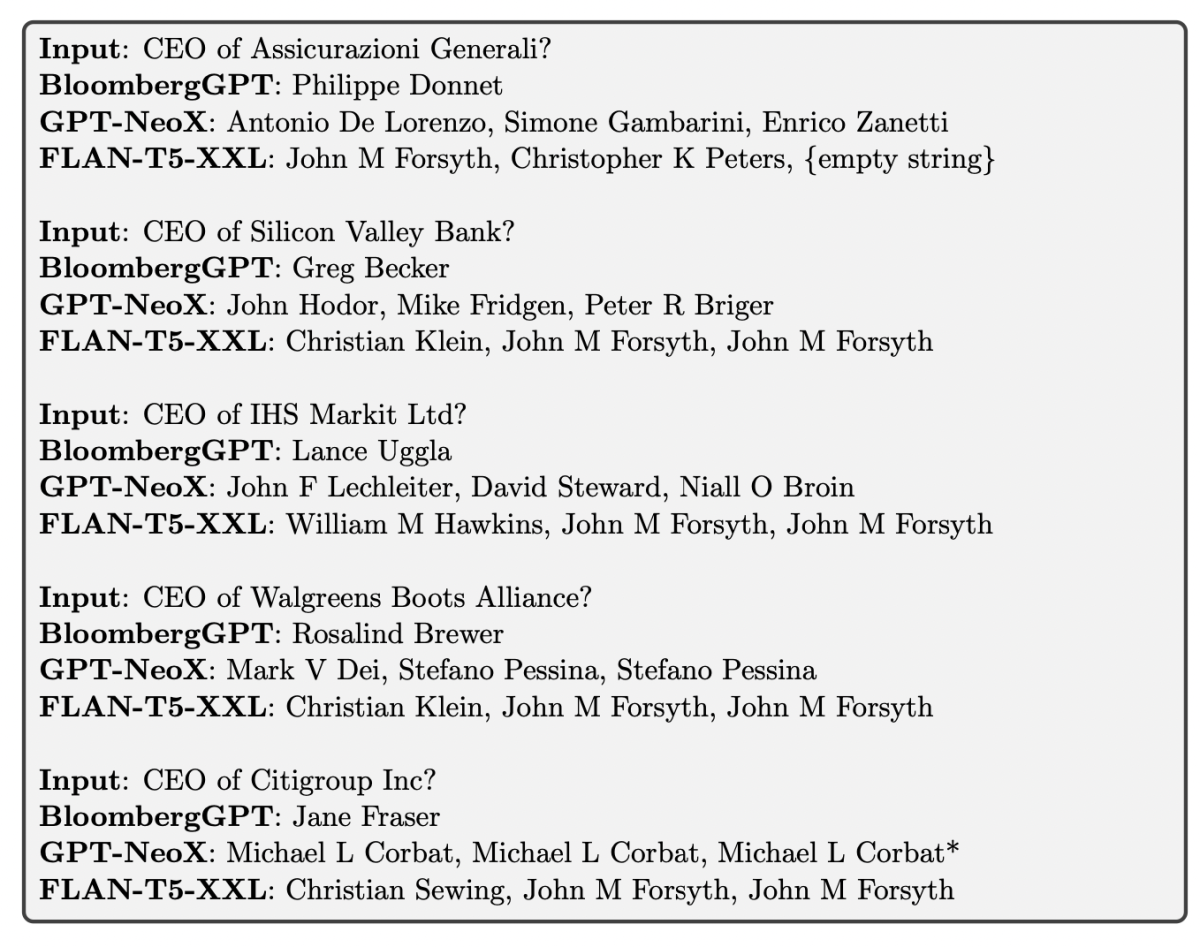
Source: “BloombergGPT: A Large Language Model for Finance”
5- Financial report generation
Generative AI can automatically create well-structured, coherent, and informative financial reports based on available data. These reports may include:
- Balance sheets
- Income statements
- Cash flow statements
This automation not only streamlines the reporting process and reduces manual effort, but it also ensures consistency, accuracy, and timely delivery of reports.
Moreover, generative AI models can be used to generate customized financial reports or visualizations tailored to specific user needs, making them even more valuable for businesses and financial professionals.
6- Fraud detection
Generative AI can be used for fraud detection in finance by generating synthetic examples of fraudulent transactions or activities. These generated examples can help train and augment machine learning algorithms to recognize and differentiate between legitimate and fraudulent patterns in financial data.
The enhanced understanding of fraud patterns allows these models to identify suspicious activities more accurately and effectively, leading to faster detection and prevention of fraud. By incorporating generative AI in fraud detection systems, financial institutions can:
- Improve the overall security and integrity of their operations
- Minimize losses due to fraud
- Maintain consumer trust
7- Generating applicant-friendly denial explanations
AI plays a significant role in the banking sector, particularly in loan decision-making processes. It helps banks and financial institutions assess customers’ creditworthiness, determine appropriate credit limits, and set loan pricing based on risk. However, both decision-makers and loan applicants need clear explanations of AI-based decisions, such as reasons for application denials, to foster trust and improve customer awareness for future applications.
A conditional generative adversarial network (GAN), a generative AI variant, was used to generate user-friendly denial explanations. By organizing denial reasons hierarchically from simple to complex, two-level conditioning is employed to generate more understandable explanations for applicants (Figure 3).
Figure 3. AI generated loan denial explanations
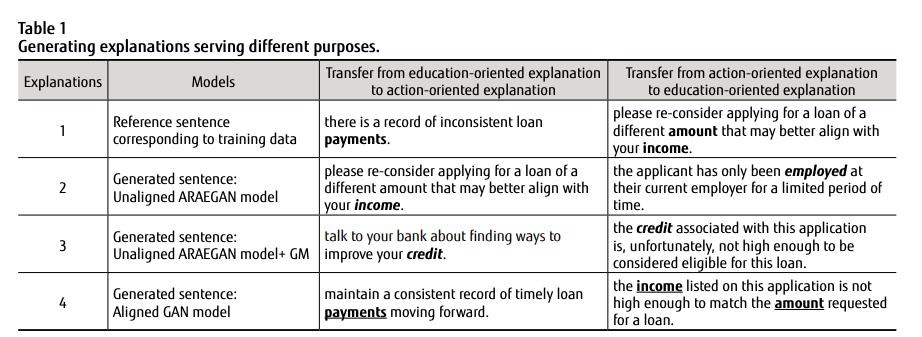
Source: “Generating User-Friendly Explanations for Loan Denials Using Generative Adversarial Networks”
8- Portfolio management and risk management
Another financial application of generative AI can be portfolio optimization. By analyzing historical financial data and generating various investment scenarios, generative AI models can help asset managers and investors identify optimal asset and wealth management, taking into account factors such as:
- Risk tolerance
- Expected returns
- Investment horizons
These models can simulate different market conditions, economic environments, and events to better understand the potential impacts on portfolio performance. This allows financial professionals to develop and fine-tune their investment strategies, optimize risk-adjusted returns, and make more informed decisions about managing their portfolios. This ultimately leads to improved financial outcomes for their clients or institutions.
9- Synthetic data generation
Since customer information is proprietary data for finance teams, it introduces some problems in terms of its use and regulation. Generative AI can be employed by financial institutions to produce synthetic data that adheres to privacy regulations such as GDPR and CCPA. By learning patterns and relationships from real financial data, generative AI models are able to create synthetic datasets that closely resemble the original data while preserving data privacy.
These synthetic datasets can be used for various purposes by financial institutions without exposing sensitive customer information, such as:
- Training machine learning models
- Conducting stress tests
- Validating models
For more on synthetic data, you can check our articles comparing synthetic data and real data, or comparing synthetic data and data masking methods for data privacy.
10- Sentiment analysis
Sentiment analysis, an approach within NLP, categorizes texts, images, or videos according to their emotional tone as negative, positive, or neutral. By gaining insights into customers’ emotions and opinions, companies can devise strategies to enhance their services or products based on these findings.
Financial institutions also benefit from sentiment analysis to measure their brand reputation and customer satisfaction through social media posts, news articles, or other sources.
Check out our article on stock market sentiment analysis to learn more.
For example, BloombergGPT was also evaluated in the sentiment analysis task. As a fine-tuned generative model for finance, it outperformed other models by succeeding in sentiment analysis.
If you have questions or need help in finding the right vendors, we can help: