Examining the Influence Between NLP and Other Fields of Study
A cautionary note on how NLP papers are drawing proportionally less from other fields over time
Natural Language Processing (NLP) is poised to substantially influence the world. However, significant progress comes hand-in-hand with substantial risks. Addressing them requires broad engagement with various fields of study. Join us on this empirical and visual quest (with data and visualizations) as we explore questions such as:
- Which fields of study are influencing NLP? And to what degree?
- Which fields of study is NLP influencing? And to what degree?
- How have these changed over time?
This blog post presents some of the key results from our EMNLP 2023 paper:
Jan Philip Wahle, Terry Ruas, Mohamed Abdalla, Bela Gipp, Saif M. Mohammad. We are Who We Cite: Bridges of Influence Between Natural Language Processing and Other Academic Fields (2023) Proceedings of the 2023 Conference on Empirical Methods in Natural Language Processing (EMNLP), Singapore. BibTeX
Blog post by Jan Philip Wahle and Saif M. Mohammad
Original Idea: Saif M. Mohammad
MOTIVATION
A fascinating aspect of science is how different fields of study interact and influence each other. Many significant advances have emerged from the synergistic interaction of multiple disciplines. For example, the conception of quantum mechanics is a theory that coalesced Planck’s idea of quantized energy levels, Einstein’s photoelectric effect, and Bohr’s atom model.
The degree to which the ideas and artifacts of a field of study are helpful to the world is a measure of its influence.
Developing a better sense of the influence of a field has several benefits, such as understanding what fosters greater innovation and what stifles it, what a field has success at understanding and what remains elusive, or who are the most prominent stakeholders benefiting and who are being left behind.
Mechanisms of field-to-field influence are complex, but one notable marker of scientific influence is citations. The extent to which a source field cites a target field is a rough indicator of the degree of influence of the target on the source. We note here, though, that not all citations are equal and subject to various biases. Nonetheless, meaningful inferences can be drawn at an aggregate level; for example, if the proportion of citations from field x to a target field y has markedly increased as compared to the proportion of citations from other fields to the target, then it is likely that the influence of x on y has grown.
WHY NLP?
While studying influence is useful for any field of study, we focus on Natural language Processing (NLP) research for one critical reason.
NLP is at an inflection point. Recent developments in large language models have captured the imagination of the scientific world, industry, and the general public.
Thus, NLP is poised to exert substantial influence despite significant risks. Further, language is social, and its applications have complex social implications. Therefore, responsible research and development need engagement with a wide swathe of literature (arguably, more so for NLP than other fields).
By tracing hundreds of thousands of citations, we systematically and quantitatively examine broad trends in the influence of various fields of study on NLP and NLP’s influence on them.
We use Semantic Scholar’s field of study attribute to categorize papers into 23 fields, such as math, medicine, or computer science. A paper can belong to one or many fields. For example, a paper that targets a medical application using computer algorithms might be in medicine and computer science. NLP itself is an interdisciplinary subfield of computer science, machine learning, and linguistics. We categorize a paper as NLP when it is in the ACL Anthology, which is arguably the largest repository of NLP literature (albeit not a complete set of all NLP papers).
Data Overview
- 209m papers and 2.5b citations from various fields (Semantic Scholar): For each citation, the field of study of the citing and cited paper.
- Semantic Scholar’s field of study attribute to categorize papers into 23 fields, such as math, medicine, or computer science.
- 77K NLP papers from 1965 to 2022 (ACL Anthology)
Q1. Who influences NLP? Who is influenced by NLP?
To understand this, we are particularly interested in two types of citations:
- Outgoing Citations: Which fields are cited by NLP papers?
- Incoming Citations: Which fields cite NLP papers?
Figure 1 visualizes the flow of citations between NLP and CS/non-CS papers.
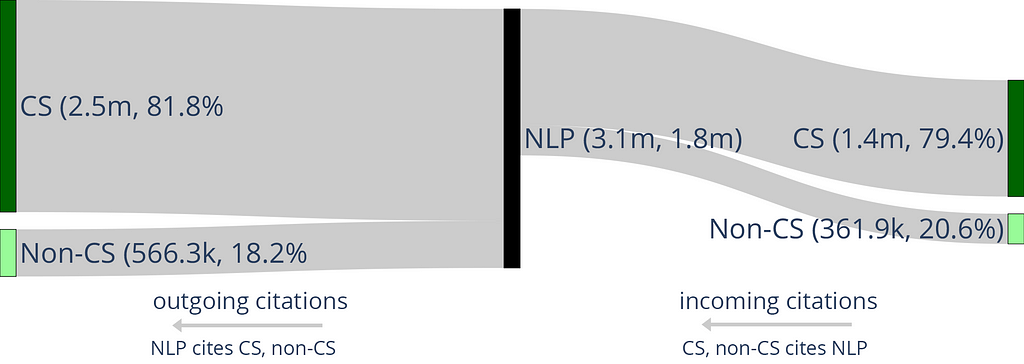
Of all citations, 79.4% received by NLP are from Computer Science (CS) papers. Similarly, more than 4 of 5 citations (81.8%) from NLP papers go to CS papers. But how has that changed over time? Has NLP always been citing as much CS?
Figure 2 (a) shows the percentage of citations from NLP to CS and non-CS papers over time; Figure 2 (b) shows the percentages to NLP from CS and non-CS papers over time.
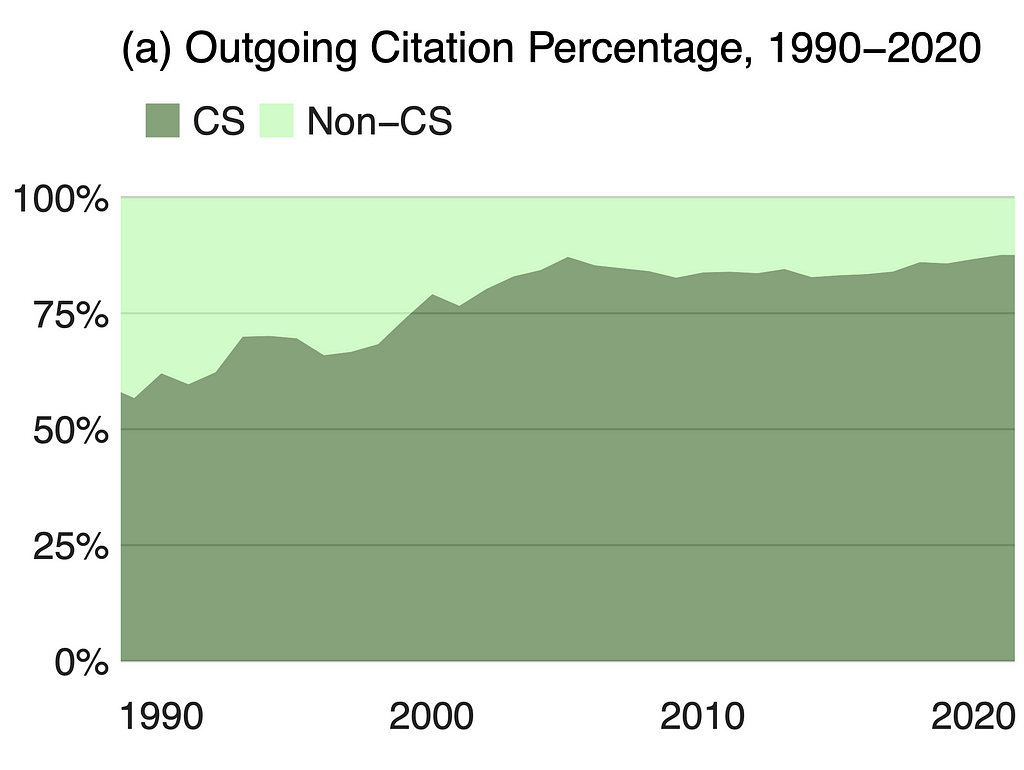
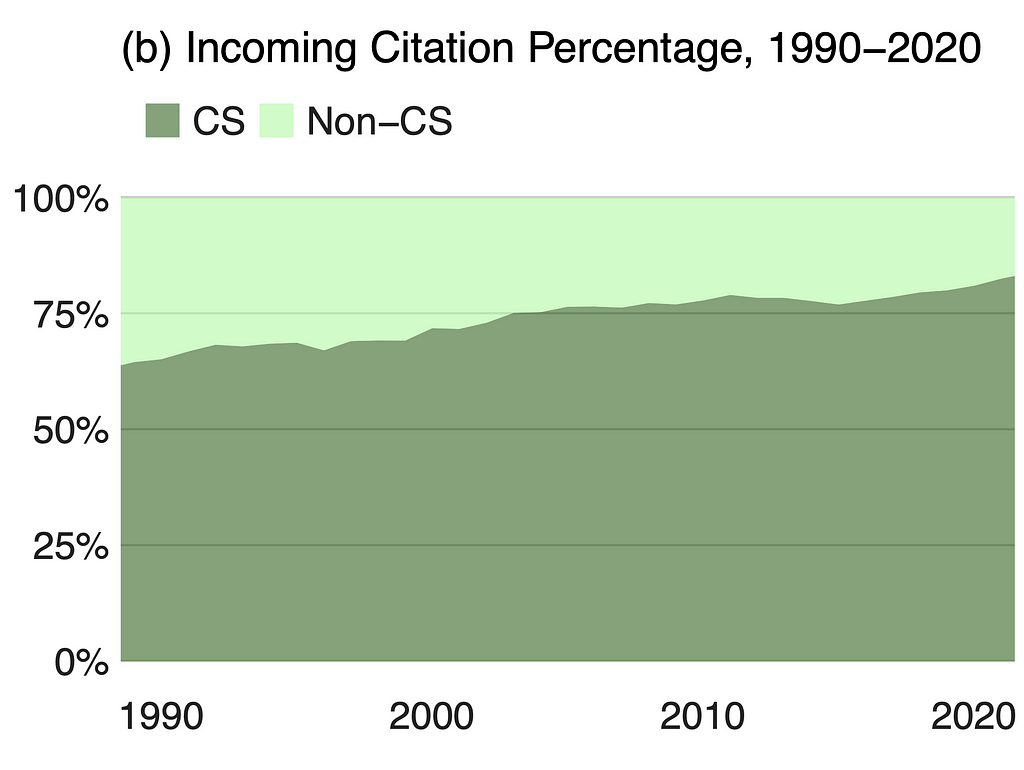
Observe that while in 1990, only about 54% of the outgoing citations were to CS, that number has steadily increased and reached 83% by 2020. This is a dramatic transformation and shows how CS-centric NLP has become over the years. The plot for incoming citations (Figure 2 (b)) shows that NLP receives most citations from CS, and that has also increased steadily from about 64% to about 81% in 2020.
Figure 3 shows a Sankey plot when we only consider the non-CS fields:
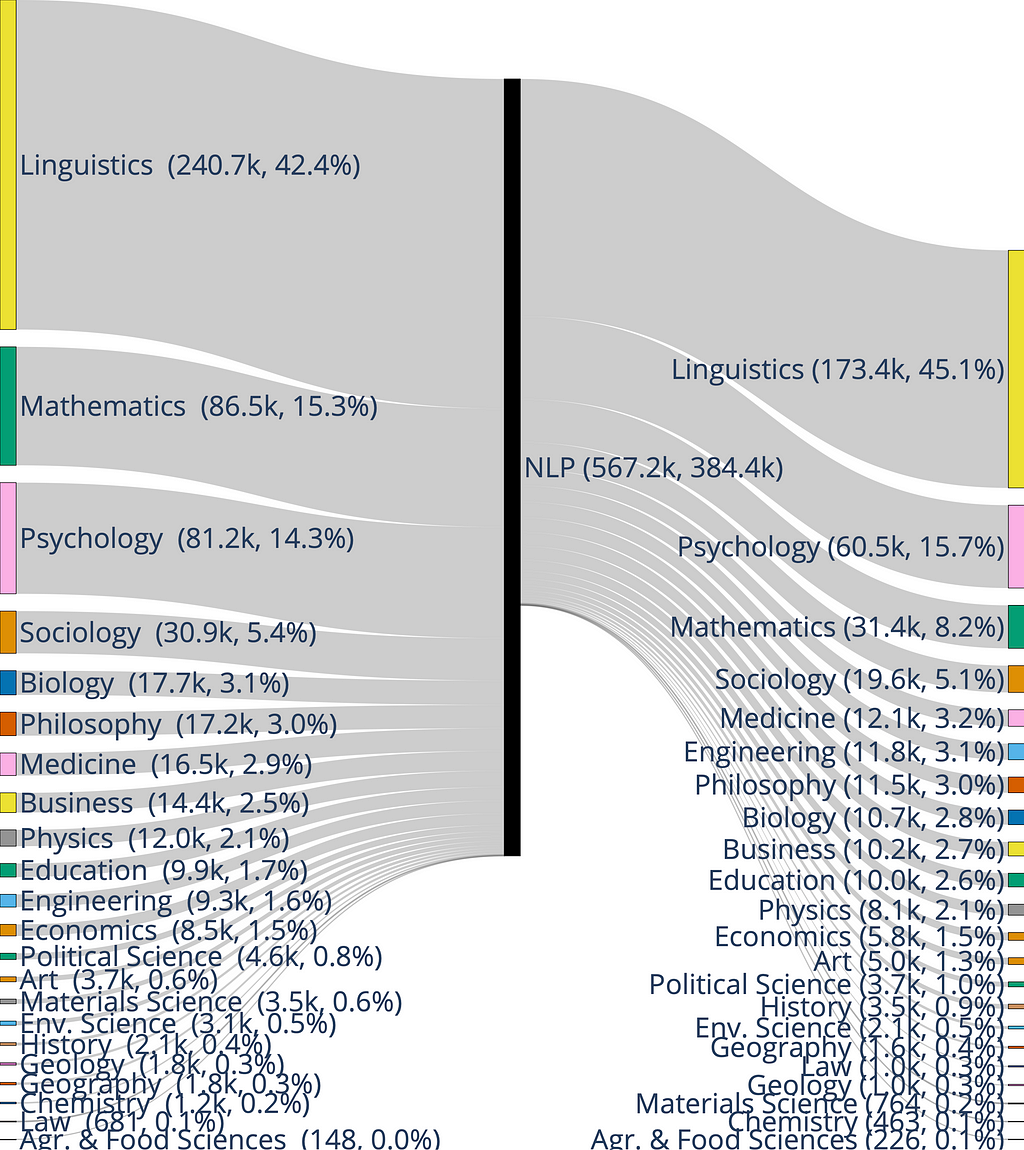
We see that linguistics, mathematics, psychology, and sociology are the most cited non-CS fields by NLP. They are also the non-CS fields that cite NLP the most, albeit the order for mathematics and psychology is swapped.
Linguistics received 42.4% of all citations from NLP to non-CS papers, and 45.1% from non-CS papers to NLP are from linguistics. NLP cites mathematics more than psychology, but more of NLP’s incites come from psychology than math.
We then wondered how these citation percentages changed over time. We suspected NLP cited linguistics more, but how much more? And what were the distributions for other fields in the past?
Figure 4 shows the citation share from NLP to non-CS papers (Figure 4(a)) and from non-CS papers to NLP (Figure 4(b)) over time.

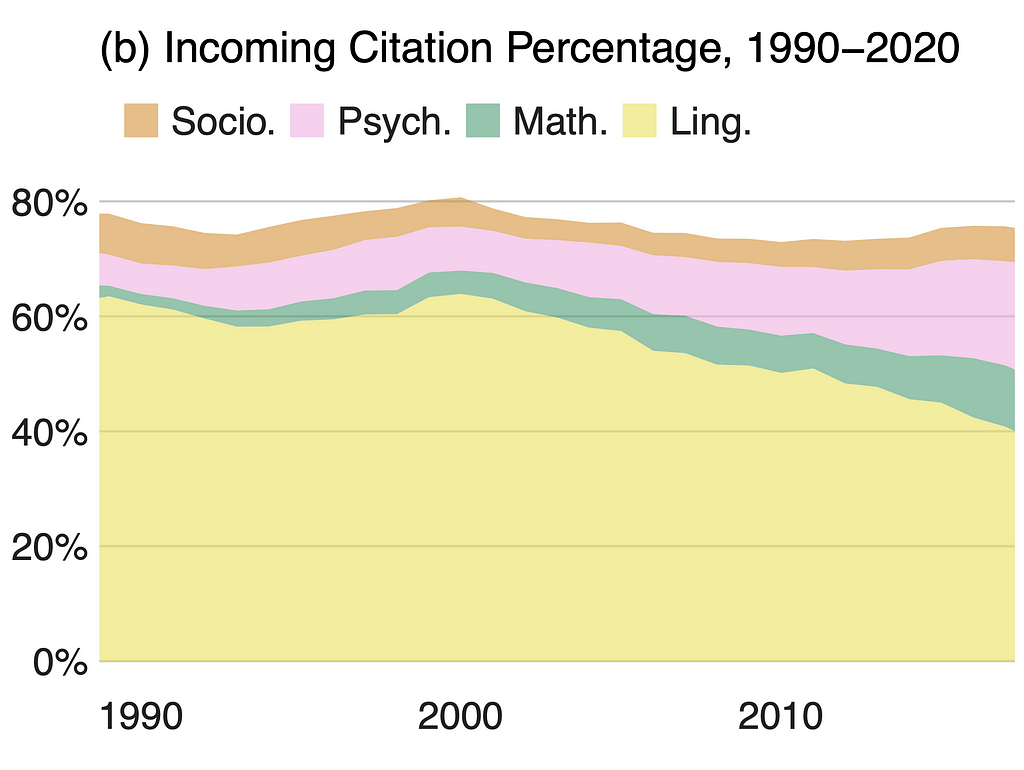
Observe that linguistics has experienced a marked (relative) decline in relevance for NLP from 2000 to 2020 (Figure 4). From 60.3% to 26.9% for outgoing citations (a) and 62.7% to 39.6% for incoming citations (b). This relative decline seems primarily because of an increase in the percentage of math citations by NLP and the percentages of citations by psychology and math to NLP.
To Summarize: Over time, both the in- and out-citations of CS have increased. These results also show a waning influence of linguistics and a marked rise in the influence of mathematics (probably due to the increasing dominance of mathematics-heavy deep learning and large language models) and psychology (probably due to increased use of psychological models of behavior, emotion, and well-being in NLP applications). The large increase in the influence of mathematics seems to have largely eaten into what used to be the influence of linguistics.
Q2. Which fields does NLP cite more than average?
As we know from earlier in this blog, 15.3% of NLP’s non-CS citations go to math, but how does that compare to other fields citing math? Are we citing math more often than the average paper from other fields?
To answer this question, we calculate the difference between NLP’s outgoing citation percentage to a field f and the macro average of the outgoing citation percentages from various fields to f. We name this metric Outgoing Relative Citational Prominence (ORCP). If NLP has an ORCP greater than 0 for f, then a greater percentage of NLP’s citations are to f than the average field. ORCP is calculated in the following way:
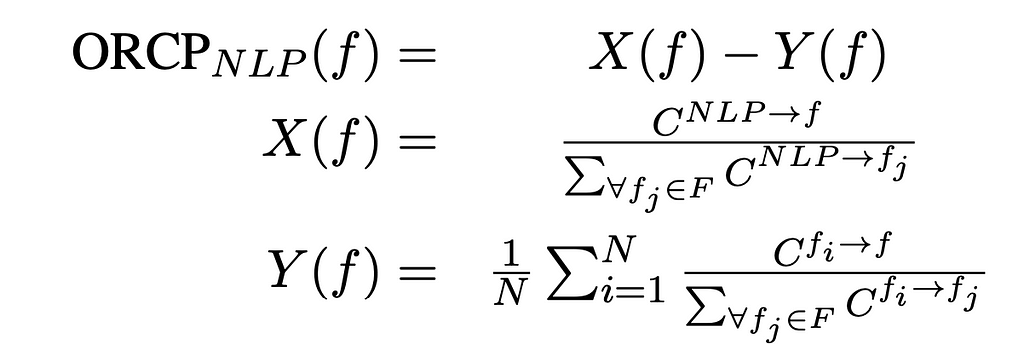
where F is the set of all fields, N is the number of all fields, and C is the number of citations from one field to another.
Figure 5 shows a plot of NLP’s ORCPs in various fields.

NLP cites CS markedly more than average (ORCP = 73.9%). This score means that NLP’s citation percentage to mathematics is 73.9 percentage points more than the average field’s citation percentage to math.
Quantifying how much more NLP cites CS over the average helps us understand how prominently we are drawing from ideas within CS. Even though linguistics is the primary source for theories of languages, NLP papers cite linguistics only 6.3 percentage points more than average (markedly lower than CS). Interestingly, even though psychology is the third most cited non-CS field by NLP (see Figure 3), it has an ORCP of −5.0, indicating that NLP cites psychology markedly less than how much the other fields cite psychology.
Q3. To what extent is NLP insular?
In this context, by insularity, we mean to what extent a field is drawing from its own literature as opposed to ideas from other fields. One way to estimate insularity is to compute how many citations of papers go to the same field as opposed to papers from other fields. For NLP and each of the 23 fields of study, we measure this percentage of intra-field citations, i.e., the number of citations from a field to itself over all outgoing citations.
Figure 6 shows the percentage of intra-field citations over time.
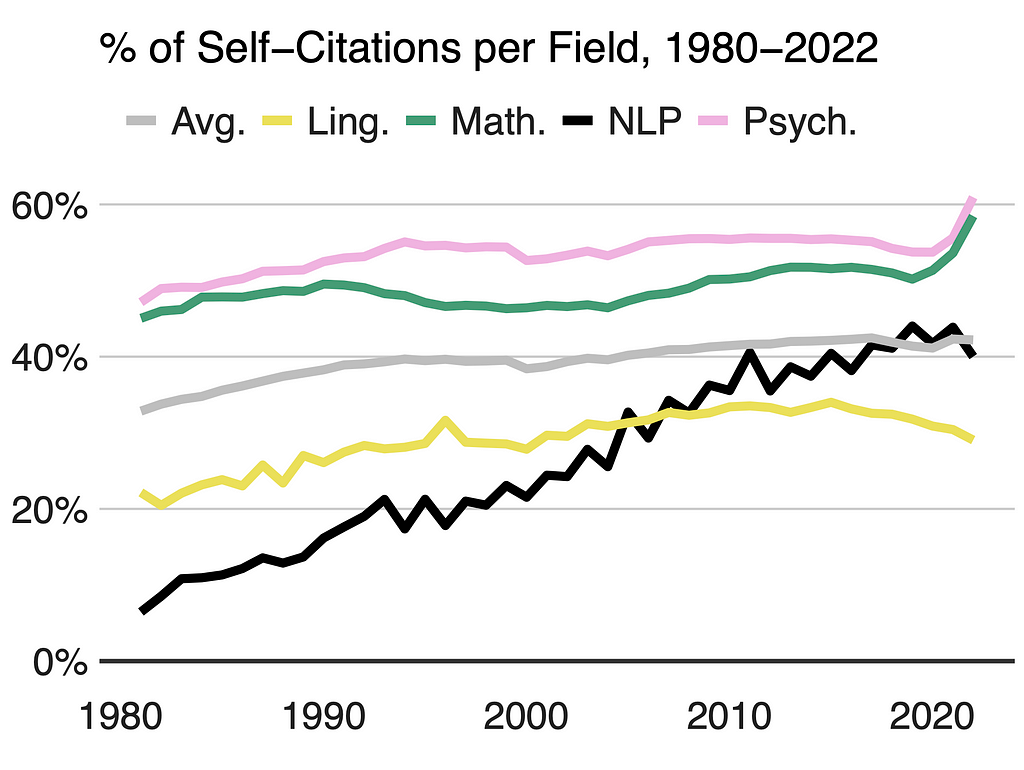
In 1980, only 5%, or every 20th citation from an NLP paper, was to another NLP paper (Figure 6). Since then, the proportion has increased substantially, to 20% in 2000 and 40% in 2020, an increase of ten percentage points per decade. In 2022, NLP reached the same intra-field citation percentage as the average intra-field citation percentage of all fields.
Compared to other fields, such as linguistics, NLP experienced particularly strong growth in intra-field citations after a lower score start. This is probably because NLP, as a field, is younger than others and was much smaller during the 1980s and 90s. Linguistics’s intra-citation percentage has increased slowly from 21% in 1980 to 33% in 2010 but decreased ever since. Interestingly, math and psychology had a mostly steady intra-field citation percentage over time but recently experienced a swift increase.
Given that a paper can also belong to one or more fields, we can also estimate how interdisciplinarity papers are by measuring the number of fields a paper belongs to and how that compares to other academic fields.
Figure 7 shows the average number of fields per paper over time.
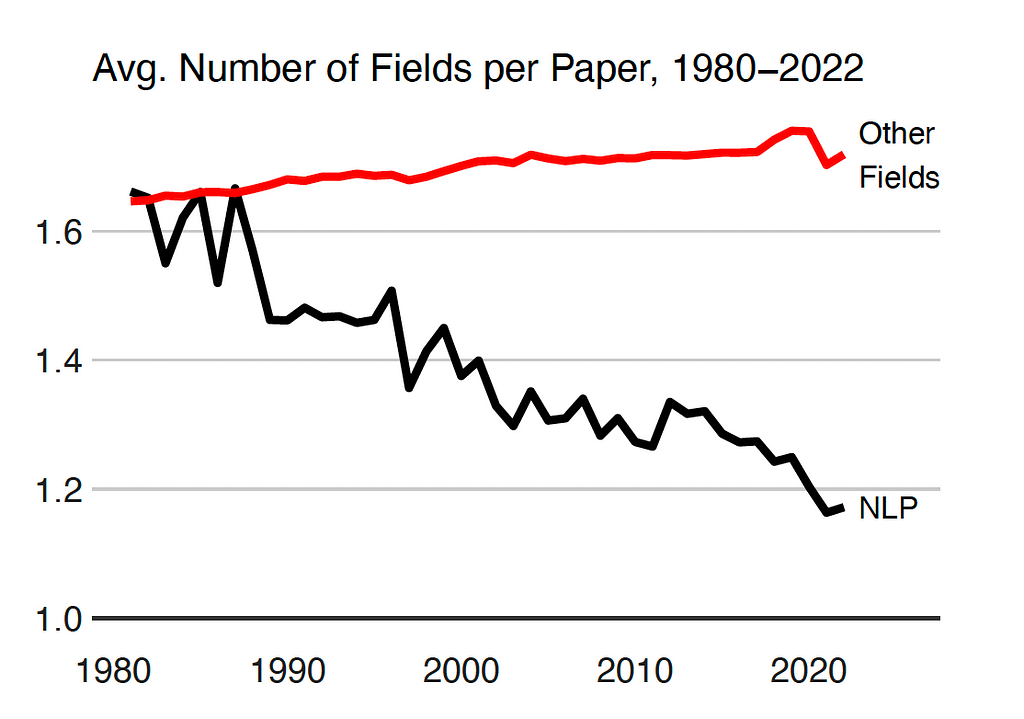
The average number of fields per NLP paper was comparable to other fields in 1980. However, the trends for NLP and other fields from 1980 to 2020 diverge sharply. While papers in other fields have become slightly more interdisciplinary, NLP papers have become less concerned with multiple fields.
Q4. Is there a bottom-line metric that captures the degree of diversity of outgoing citations? How did the diversity of outgoing citations to NLP papers (as captured by this metric) change over time? And the same questions for incoming citations?
To capture how diversely NLP is citing various fields in a single metric, we introduce the Citation Field Diversity Index (CFDI).
CFDI measures a paper’s diversity in citations to various fields. In simpler terms, a higher CFDI indicates that a paper cites papers from various fields. This metric offers insights into the spread of scholarly cross-field influence. The CFDI is defined using the Gini-Simpson index as follows:

here, xf is the number of papers in field f, and N is the total number of citations. Scores close to 1 indicate that the number of citations from the target field (in our case, NLP) to each of the 23 fields is roughly uniform. A score of 0 indicates that all the citations go to just one field. Incoming CFDI is calculated similarly, except by considering citations from other fields to the target field (NLP).
Figure 8 shows outgoing CFDI (a) and incoming CFDI (b) over time.
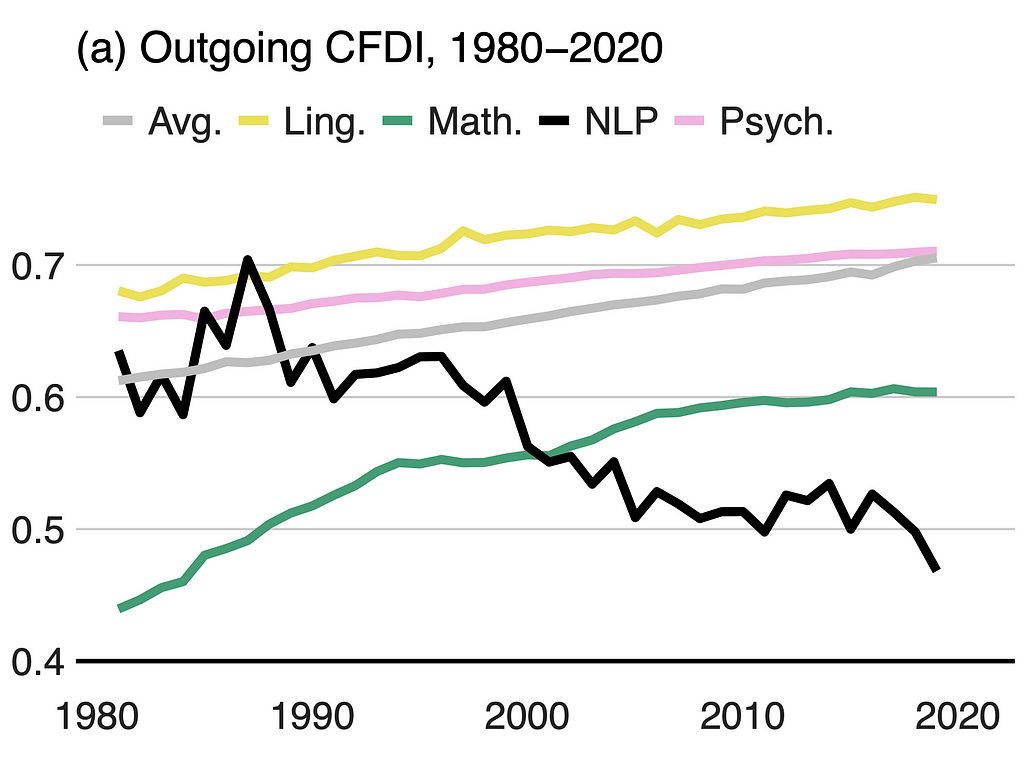
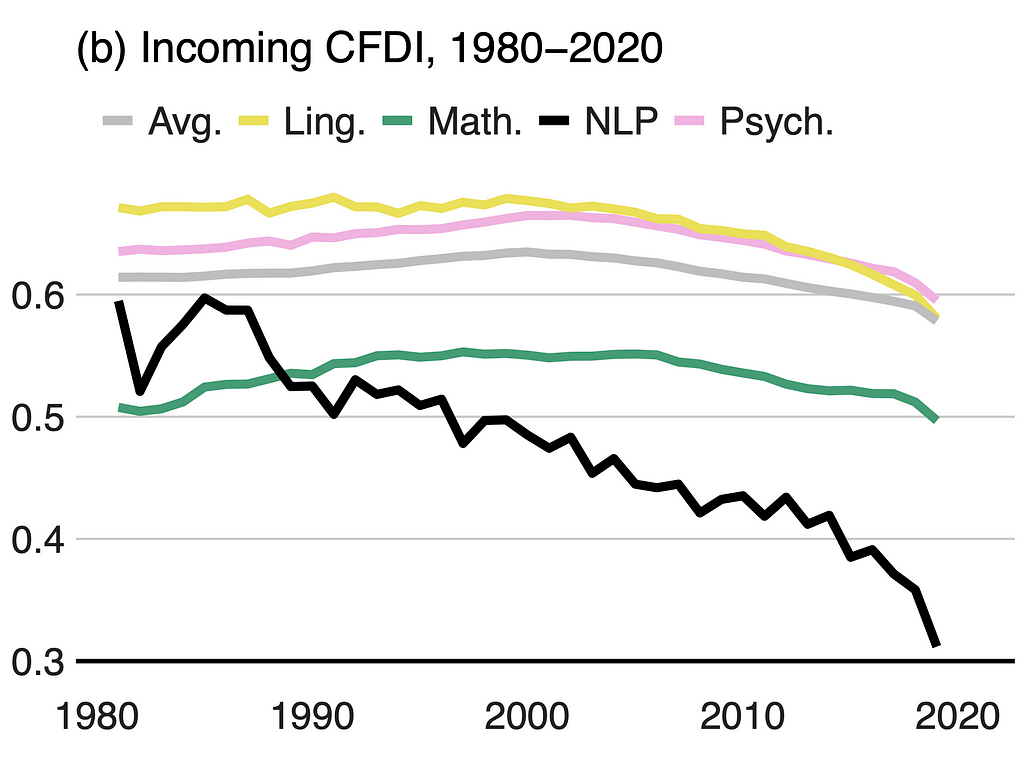
While the average outgoing CFDI has slowly increased with time, NLP has experienced a swift decline over the last four decades. The average outgoing CFDI has grown by 0.08, while NLP’s outgoing CFDI declined by about 30% (0.18) from 1980–2020. CFDI for linguistics and psychology follow a similar trend to the average, while math has experienced a large increase in outgoing CFDI of 0.16 over four decades.
Similar to outgoing CFDI, NLP papers also have a marked decline in incoming CFDI over time, indicating that incoming citations are coming mainly from one (or few fields) instead of many. Figure 5 (b) shows the plot. While the incoming CFDIs for other fields, and the average, remained steady from 1980 to 2010, NLP’s incoming CFDI has decreased from 0.59 to 0.42. In the 2010s, all fields declined in CFDI, but NLP had a particularly strong fall (0.42 to 0.31).
Key Takeaways
- NLP draws mainly from ideas within CS (>80%). After CS, NLP is most influenced by linguistics, mathematics, psychology, and sociology
- Over time, NLP’s citations to non-CS fields and its diversity in citing different fields decreased while other fields have remained steady
- Papers in NLP are getting less interdisciplinary, increasingly residing in only one field and citing their own field
DISCUSSION. A key point about scientific influence is that, as researchers, we are not just passive objects of influence. Whereas some influences are hard to ignore (say, because of substantial buy-in from one’s peers and the review process), others are a direct result of active engagement by the researcher with relevant literature (possibly from a different field).
We (can) choose whose literature to engage with and thus benefit from.
Similarly, while at times we may happen to influence other fields, we (can) choose to engage with other fields so that they are more aware of our work.
Such an engagement can be through cross-field exchanges at conferences, blog posts about one’s work for a target audience in another field, etc.
Over the last five years, NLP technologies have been widely deployed, impacting billions of people directly and indirectly. Numerous instances have also surfaced where it is clear that adequate thought was not given to developing those systems, leading to various adverse outcomes. It has also been well-established that a crucial ingredient in developing better systems is to engage with a wide range of literature and ideas, especially bringing in ideas from outside of CS (say, psychology, social science, linguistics, etc.)
Against this backdrop, the picture of NLP’s striking lack of engagement with the broader research literature — especially when marginalized communities continue to face substantial risks from its technologies — is an indictment of the field of NLP.
Not only are we not engaging with outside literature more than the average field or even just the same as other fields, but our engagement is, in fact, markedly less. A trend that is only getting worse.
THE GOOD NEWS is that this can change. It is a myth to believe that citation patterns are “meant to happen” or that individual researchers and teams do not have a choice in what they cite. We can actively choose what literature we engage with. We can work harder to tie our work with ideas in linguistics, psychology, social science, and beyond. Using language and computation, we can work more on problems that matter to other fields. By keeping an eye on work in other fields, we can bring their ideas to new compelling forms in NLP.
As researchers, mentors, reviewers, and committee members, we should ask ourselves, how are we:
influencing the broader exchange of ideas across fields
and consciously acting against:
focusing excessively on CS works at the expense of relevant work from other fields, such as psychology, sociology, and linguistics.
Epilogue
Online Tool to Calculate Cross-Field Influence
To facilitate reflection on the papers we cite from various fields, we created an online public tool to compute the amount of cross-field citations for individual papers and even sets of papers (e.g., author profiles, proceedings). Insert a Semantic Scholar or ACL Anthology URL, and the tool computes various metrics such as the most cited fields, the citation field diversity, and so on. Or upload a PDF draft, and the bibliography will be parsed and linked to their corresponding fields.
You will be able to answer questions such as:
- Which fields influence me (as an author) the most?
- What is the field diversity of my submission draft?
- What are the most relevant fields to this particular proceeding?
By answering these questions, you may find yourself reflecting further:
- “Are there ideas rooted in other fields that can be used innovatively in my research?”
- “Can I expand my literature search to include works from other fields?”
For a quick introduction to the tool, see this short video.
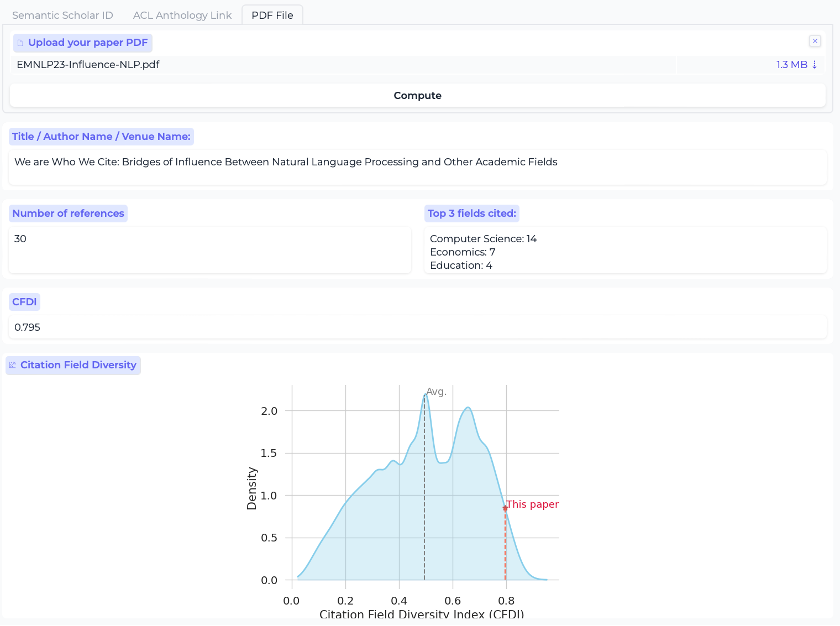
Acknowledgments
This work was partially supported by the DAAD (German Academic Exchange Service) under grant no. 9187215, the Lower Saxony Ministry of Science and Culture, and the VW Foundation. Many thanks to Roland Kuhn, Andreas Stephan, Annika Schulte-Hürmann, and Tara Small for their thoughtful discussions.
Unless otherwise noted, all images are by the author.
Related Works of Interest
- Geographic Citation Gaps in NLP (EMNLP, 2022)
- D3: A Massive Dataset of Scholarly Metadata for Analyzing the State of Computer Science Research (LREC, 2022)
- Forgotten Knowledge: Examining the Citational Amnesia in NLP (ACL, 2023)
- The Elephant in the Room: Analyzing the Presence of Big Tech in NLP Research (ACL, 2023)
- We are Who We Cite: Bridges of Influence Between Natural Language
Processing and Other Academic Fields (EMNLP, 2023)
Jan Philip Wahle
Research Staff, University of Göttingen, Germany
Visiting Researcher, National Research Council, Canada
Twitter: @jpwahle
Webpage: https://jpwahle.com/
Saif M. Mohammad
Senior Research Scientist, National Research Council Canada
Twitter: @saifmmohammad
Webpage: http://saifmohammad.com/
Examining the Influence Between NLP and Other Fields of Study was originally published in Towards Data Science on Medium, where people are continuing the conversation by highlighting and responding to this story.
A cautionary note on how NLP papers are drawing proportionally less from other fields over time
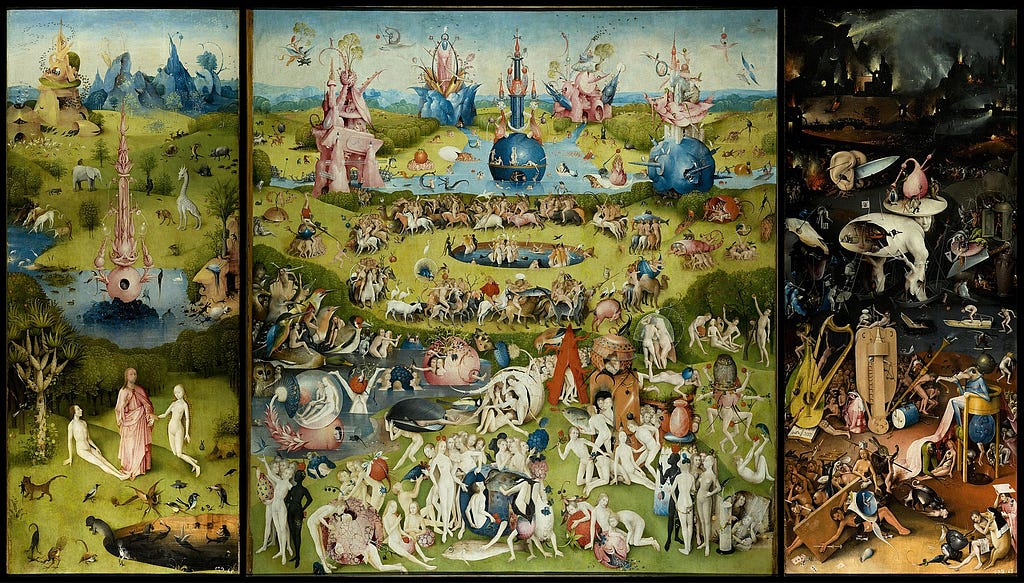
Natural Language Processing (NLP) is poised to substantially influence the world. However, significant progress comes hand-in-hand with substantial risks. Addressing them requires broad engagement with various fields of study. Join us on this empirical and visual quest (with data and visualizations) as we explore questions such as:
- Which fields of study are influencing NLP? And to what degree?
- Which fields of study is NLP influencing? And to what degree?
- How have these changed over time?
This blog post presents some of the key results from our EMNLP 2023 paper:
Jan Philip Wahle, Terry Ruas, Mohamed Abdalla, Bela Gipp, Saif M. Mohammad. We are Who We Cite: Bridges of Influence Between Natural Language Processing and Other Academic Fields (2023) Proceedings of the 2023 Conference on Empirical Methods in Natural Language Processing (EMNLP), Singapore. BibTeX
Blog post by Jan Philip Wahle and Saif M. Mohammad
Original Idea: Saif M. Mohammad
MOTIVATION
A fascinating aspect of science is how different fields of study interact and influence each other. Many significant advances have emerged from the synergistic interaction of multiple disciplines. For example, the conception of quantum mechanics is a theory that coalesced Planck’s idea of quantized energy levels, Einstein’s photoelectric effect, and Bohr’s atom model.
The degree to which the ideas and artifacts of a field of study are helpful to the world is a measure of its influence.
Developing a better sense of the influence of a field has several benefits, such as understanding what fosters greater innovation and what stifles it, what a field has success at understanding and what remains elusive, or who are the most prominent stakeholders benefiting and who are being left behind.
Mechanisms of field-to-field influence are complex, but one notable marker of scientific influence is citations. The extent to which a source field cites a target field is a rough indicator of the degree of influence of the target on the source. We note here, though, that not all citations are equal and subject to various biases. Nonetheless, meaningful inferences can be drawn at an aggregate level; for example, if the proportion of citations from field x to a target field y has markedly increased as compared to the proportion of citations from other fields to the target, then it is likely that the influence of x on y has grown.
WHY NLP?
While studying influence is useful for any field of study, we focus on Natural language Processing (NLP) research for one critical reason.
NLP is at an inflection point. Recent developments in large language models have captured the imagination of the scientific world, industry, and the general public.
Thus, NLP is poised to exert substantial influence despite significant risks. Further, language is social, and its applications have complex social implications. Therefore, responsible research and development need engagement with a wide swathe of literature (arguably, more so for NLP than other fields).
By tracing hundreds of thousands of citations, we systematically and quantitatively examine broad trends in the influence of various fields of study on NLP and NLP’s influence on them.
We use Semantic Scholar’s field of study attribute to categorize papers into 23 fields, such as math, medicine, or computer science. A paper can belong to one or many fields. For example, a paper that targets a medical application using computer algorithms might be in medicine and computer science. NLP itself is an interdisciplinary subfield of computer science, machine learning, and linguistics. We categorize a paper as NLP when it is in the ACL Anthology, which is arguably the largest repository of NLP literature (albeit not a complete set of all NLP papers).
Data Overview
- 209m papers and 2.5b citations from various fields (Semantic Scholar): For each citation, the field of study of the citing and cited paper.
- Semantic Scholar’s field of study attribute to categorize papers into 23 fields, such as math, medicine, or computer science.
- 77K NLP papers from 1965 to 2022 (ACL Anthology)
Q1. Who influences NLP? Who is influenced by NLP?
To understand this, we are particularly interested in two types of citations:
- Outgoing Citations: Which fields are cited by NLP papers?
- Incoming Citations: Which fields cite NLP papers?
Figure 1 visualizes the flow of citations between NLP and CS/non-CS papers.
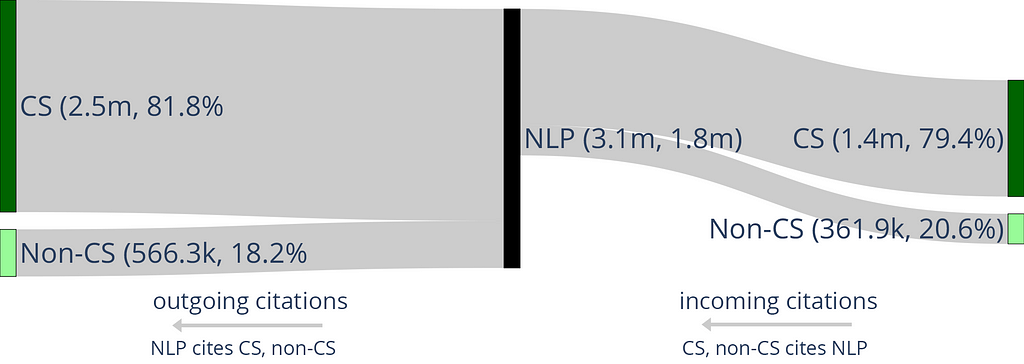
Of all citations, 79.4% received by NLP are from Computer Science (CS) papers. Similarly, more than 4 of 5 citations (81.8%) from NLP papers go to CS papers. But how has that changed over time? Has NLP always been citing as much CS?
Figure 2 (a) shows the percentage of citations from NLP to CS and non-CS papers over time; Figure 2 (b) shows the percentages to NLP from CS and non-CS papers over time.
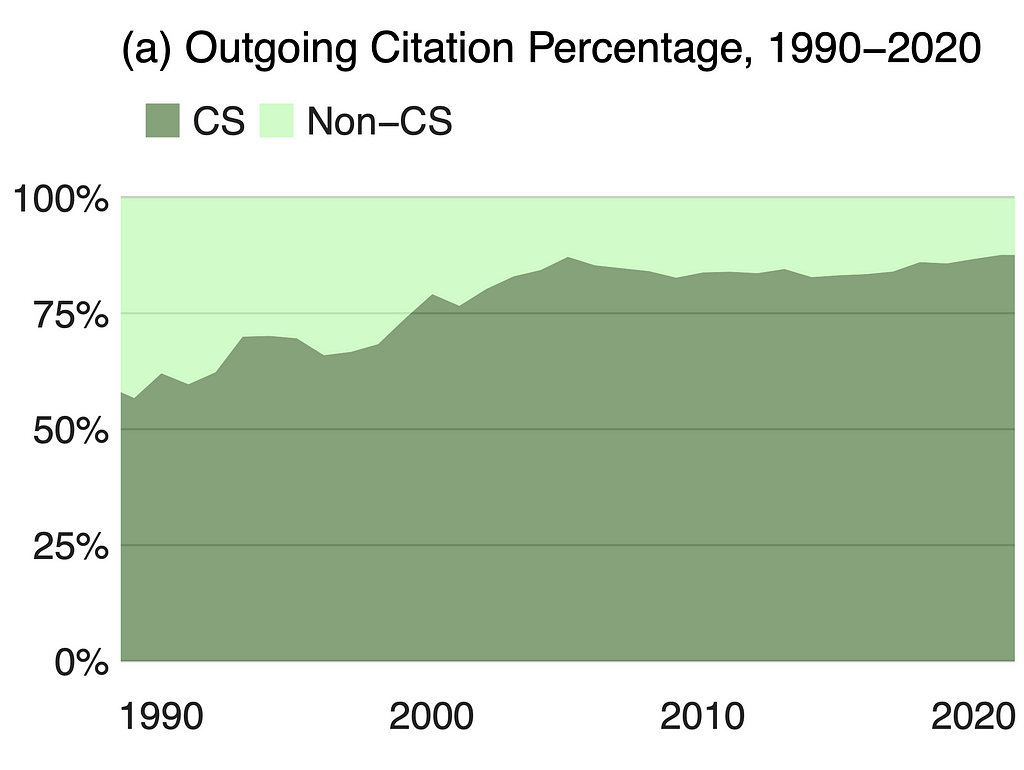
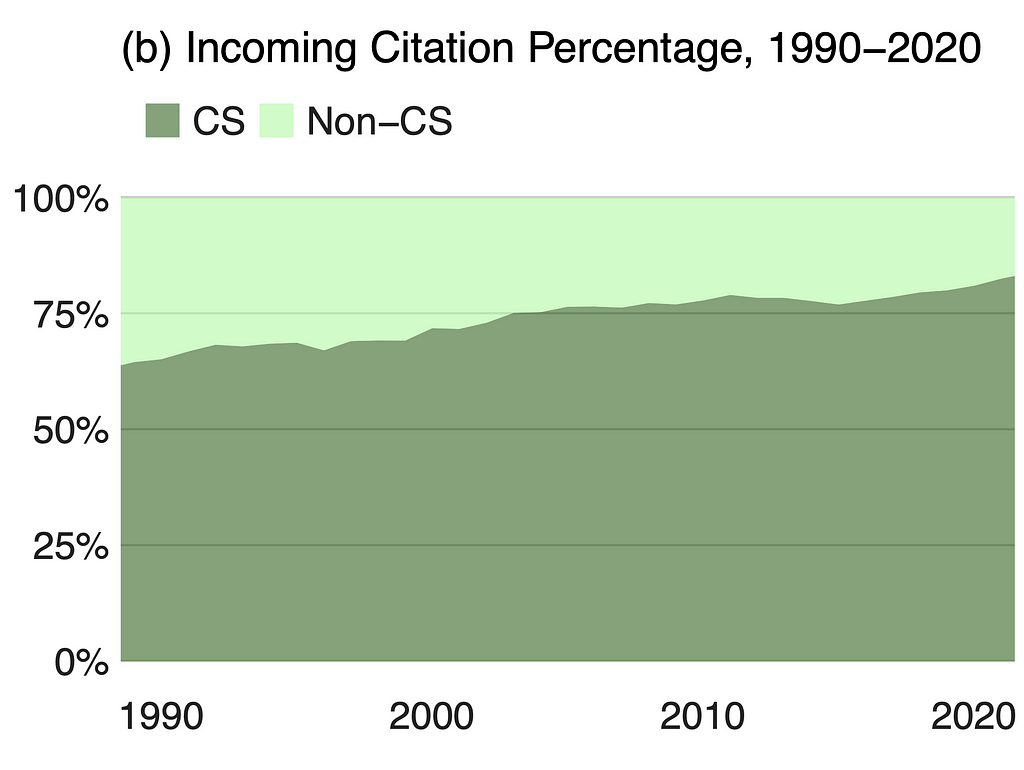
Observe that while in 1990, only about 54% of the outgoing citations were to CS, that number has steadily increased and reached 83% by 2020. This is a dramatic transformation and shows how CS-centric NLP has become over the years. The plot for incoming citations (Figure 2 (b)) shows that NLP receives most citations from CS, and that has also increased steadily from about 64% to about 81% in 2020.
Figure 3 shows a Sankey plot when we only consider the non-CS fields:
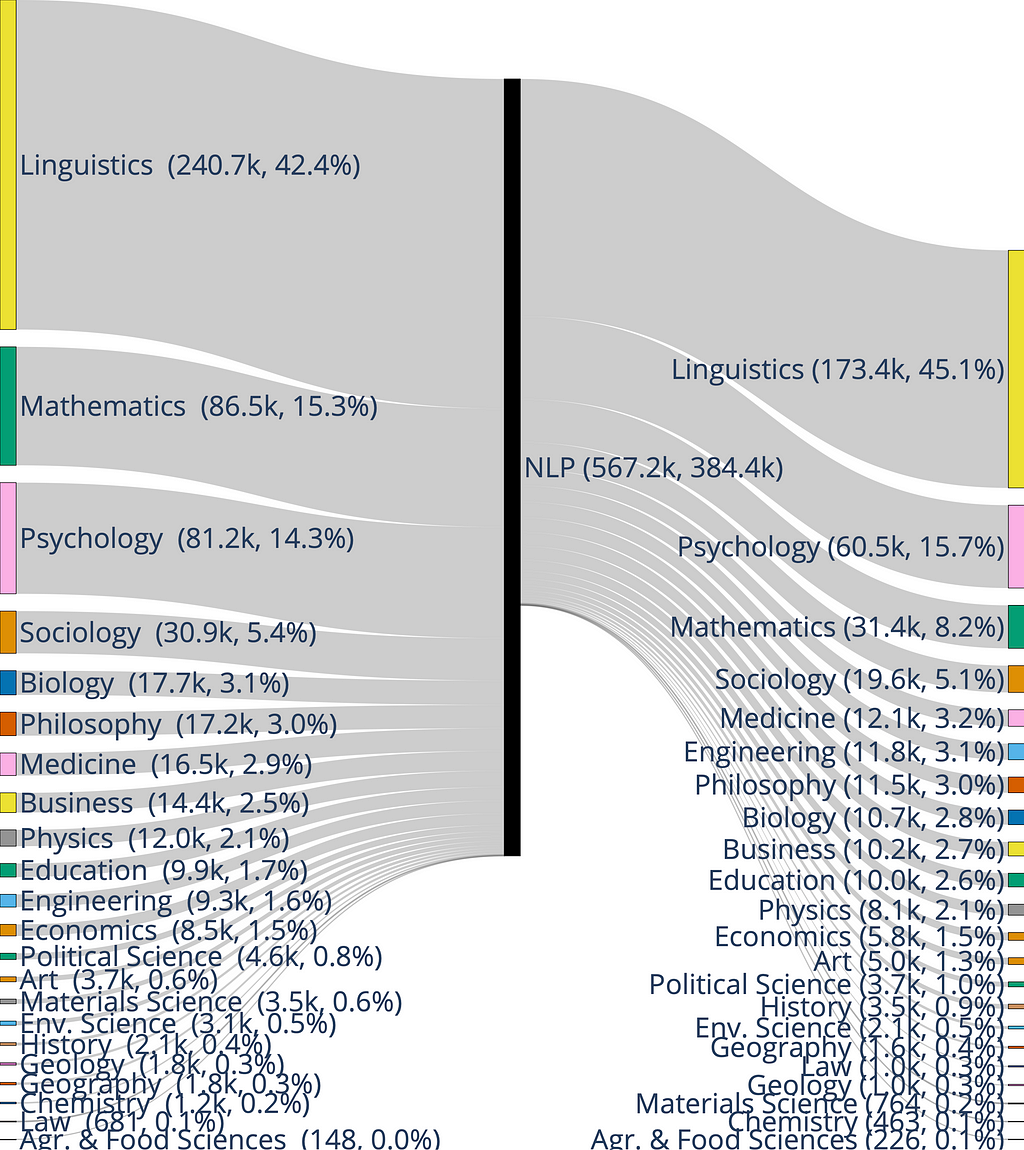
We see that linguistics, mathematics, psychology, and sociology are the most cited non-CS fields by NLP. They are also the non-CS fields that cite NLP the most, albeit the order for mathematics and psychology is swapped.
Linguistics received 42.4% of all citations from NLP to non-CS papers, and 45.1% from non-CS papers to NLP are from linguistics. NLP cites mathematics more than psychology, but more of NLP’s incites come from psychology than math.
We then wondered how these citation percentages changed over time. We suspected NLP cited linguistics more, but how much more? And what were the distributions for other fields in the past?
Figure 4 shows the citation share from NLP to non-CS papers (Figure 4(a)) and from non-CS papers to NLP (Figure 4(b)) over time.

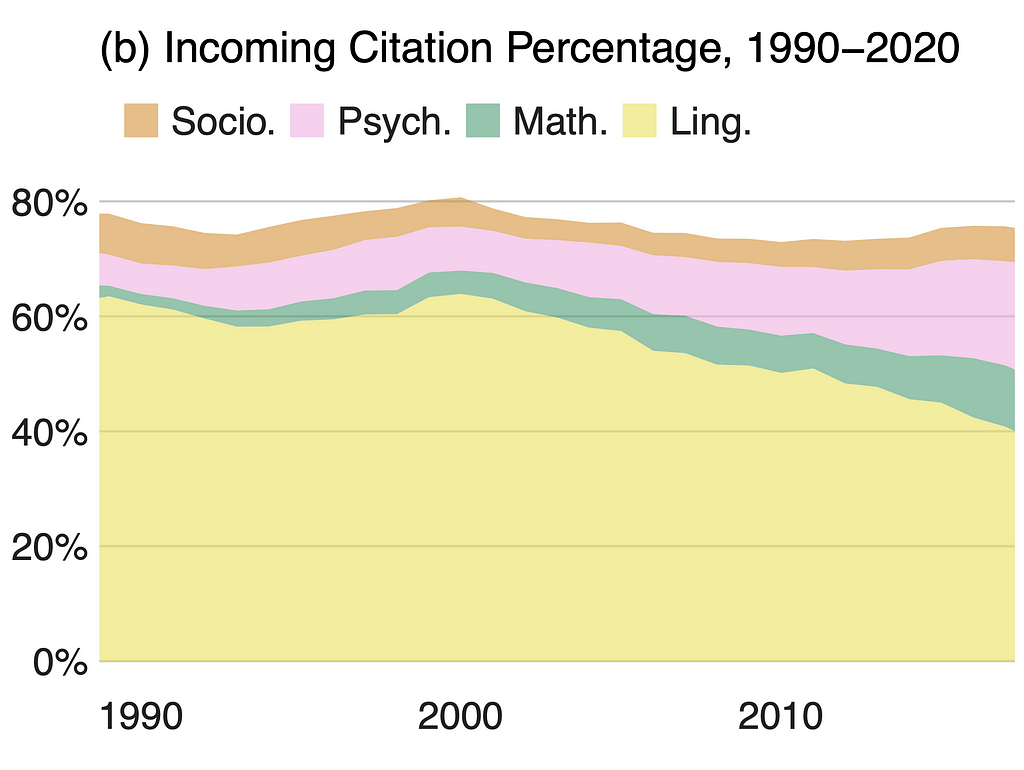
Observe that linguistics has experienced a marked (relative) decline in relevance for NLP from 2000 to 2020 (Figure 4). From 60.3% to 26.9% for outgoing citations (a) and 62.7% to 39.6% for incoming citations (b). This relative decline seems primarily because of an increase in the percentage of math citations by NLP and the percentages of citations by psychology and math to NLP.
To Summarize: Over time, both the in- and out-citations of CS have increased. These results also show a waning influence of linguistics and a marked rise in the influence of mathematics (probably due to the increasing dominance of mathematics-heavy deep learning and large language models) and psychology (probably due to increased use of psychological models of behavior, emotion, and well-being in NLP applications). The large increase in the influence of mathematics seems to have largely eaten into what used to be the influence of linguistics.
Q2. Which fields does NLP cite more than average?
As we know from earlier in this blog, 15.3% of NLP’s non-CS citations go to math, but how does that compare to other fields citing math? Are we citing math more often than the average paper from other fields?
To answer this question, we calculate the difference between NLP’s outgoing citation percentage to a field f and the macro average of the outgoing citation percentages from various fields to f. We name this metric Outgoing Relative Citational Prominence (ORCP). If NLP has an ORCP greater than 0 for f, then a greater percentage of NLP’s citations are to f than the average field. ORCP is calculated in the following way:
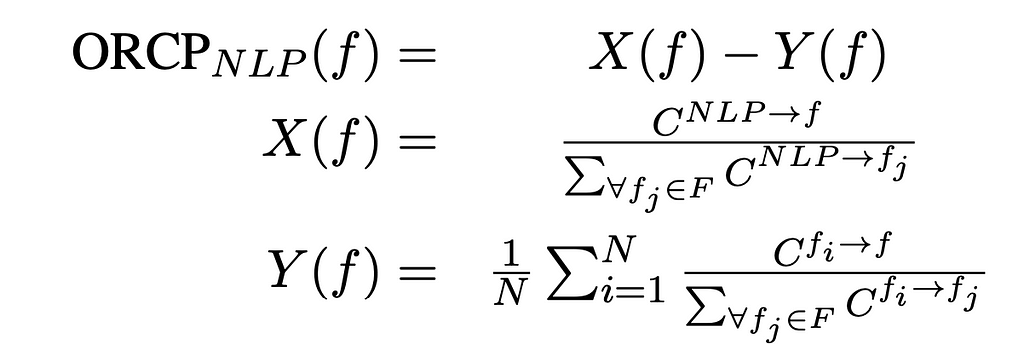
where F is the set of all fields, N is the number of all fields, and C is the number of citations from one field to another.
Figure 5 shows a plot of NLP’s ORCPs in various fields.

NLP cites CS markedly more than average (ORCP = 73.9%). This score means that NLP’s citation percentage to mathematics is 73.9 percentage points more than the average field’s citation percentage to math.
Quantifying how much more NLP cites CS over the average helps us understand how prominently we are drawing from ideas within CS. Even though linguistics is the primary source for theories of languages, NLP papers cite linguistics only 6.3 percentage points more than average (markedly lower than CS). Interestingly, even though psychology is the third most cited non-CS field by NLP (see Figure 3), it has an ORCP of −5.0, indicating that NLP cites psychology markedly less than how much the other fields cite psychology.
Q3. To what extent is NLP insular?
In this context, by insularity, we mean to what extent a field is drawing from its own literature as opposed to ideas from other fields. One way to estimate insularity is to compute how many citations of papers go to the same field as opposed to papers from other fields. For NLP and each of the 23 fields of study, we measure this percentage of intra-field citations, i.e., the number of citations from a field to itself over all outgoing citations.
Figure 6 shows the percentage of intra-field citations over time.
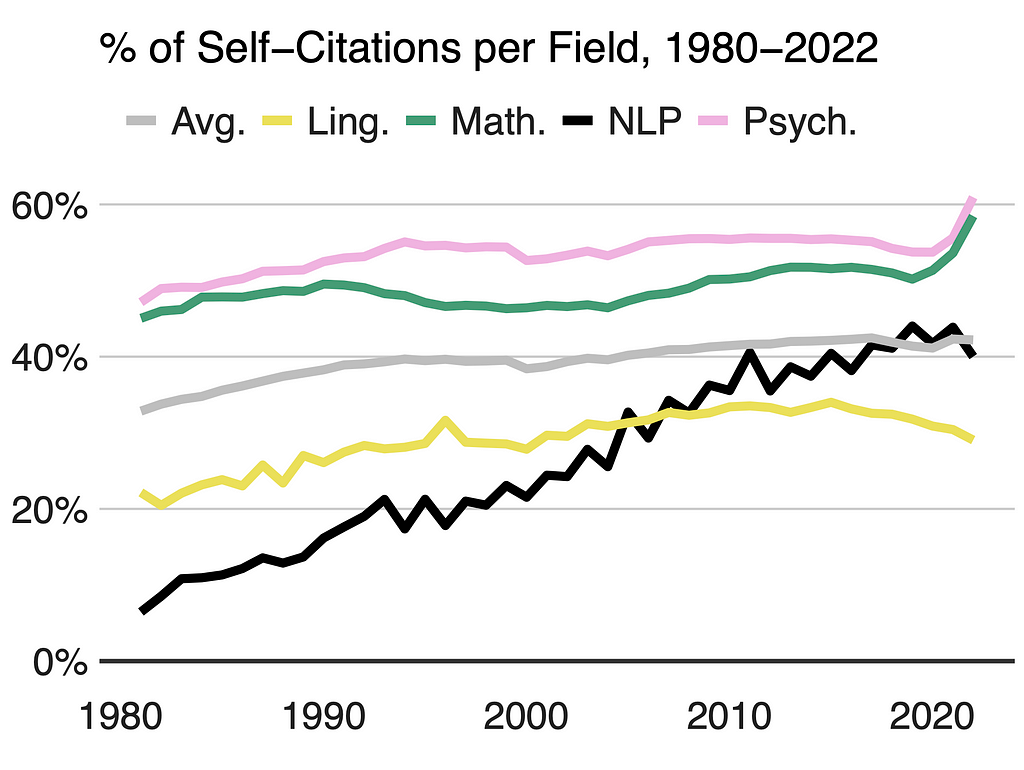
In 1980, only 5%, or every 20th citation from an NLP paper, was to another NLP paper (Figure 6). Since then, the proportion has increased substantially, to 20% in 2000 and 40% in 2020, an increase of ten percentage points per decade. In 2022, NLP reached the same intra-field citation percentage as the average intra-field citation percentage of all fields.
Compared to other fields, such as linguistics, NLP experienced particularly strong growth in intra-field citations after a lower score start. This is probably because NLP, as a field, is younger than others and was much smaller during the 1980s and 90s. Linguistics’s intra-citation percentage has increased slowly from 21% in 1980 to 33% in 2010 but decreased ever since. Interestingly, math and psychology had a mostly steady intra-field citation percentage over time but recently experienced a swift increase.
Given that a paper can also belong to one or more fields, we can also estimate how interdisciplinarity papers are by measuring the number of fields a paper belongs to and how that compares to other academic fields.
Figure 7 shows the average number of fields per paper over time.
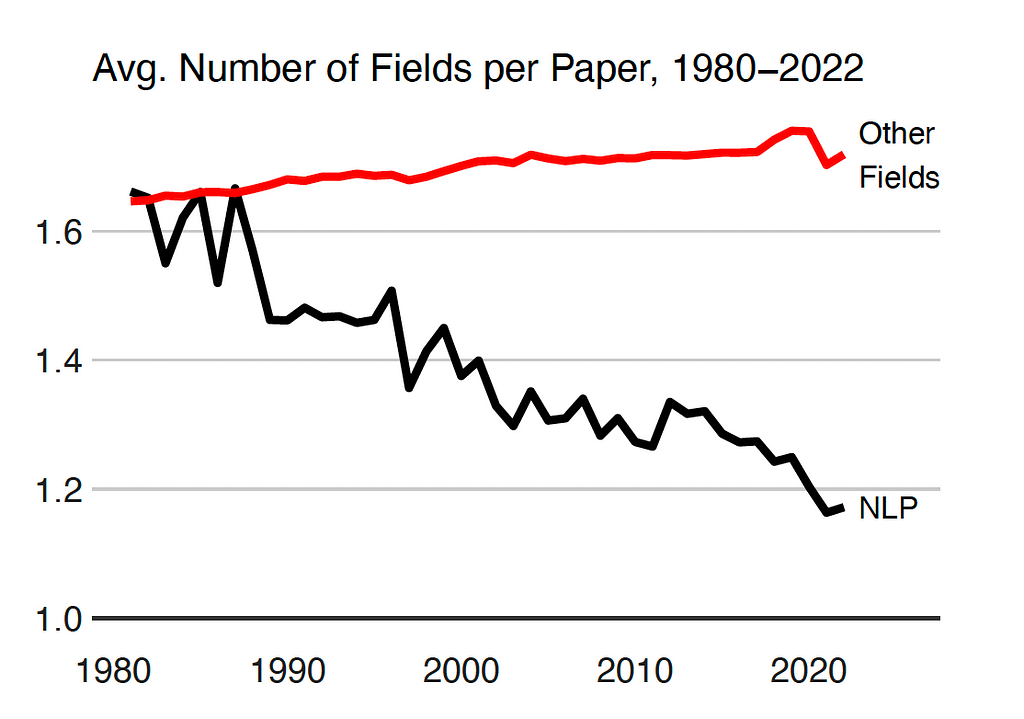
The average number of fields per NLP paper was comparable to other fields in 1980. However, the trends for NLP and other fields from 1980 to 2020 diverge sharply. While papers in other fields have become slightly more interdisciplinary, NLP papers have become less concerned with multiple fields.
Q4. Is there a bottom-line metric that captures the degree of diversity of outgoing citations? How did the diversity of outgoing citations to NLP papers (as captured by this metric) change over time? And the same questions for incoming citations?
To capture how diversely NLP is citing various fields in a single metric, we introduce the Citation Field Diversity Index (CFDI).
CFDI measures a paper’s diversity in citations to various fields. In simpler terms, a higher CFDI indicates that a paper cites papers from various fields. This metric offers insights into the spread of scholarly cross-field influence. The CFDI is defined using the Gini-Simpson index as follows:

here, xf is the number of papers in field f, and N is the total number of citations. Scores close to 1 indicate that the number of citations from the target field (in our case, NLP) to each of the 23 fields is roughly uniform. A score of 0 indicates that all the citations go to just one field. Incoming CFDI is calculated similarly, except by considering citations from other fields to the target field (NLP).
Figure 8 shows outgoing CFDI (a) and incoming CFDI (b) over time.
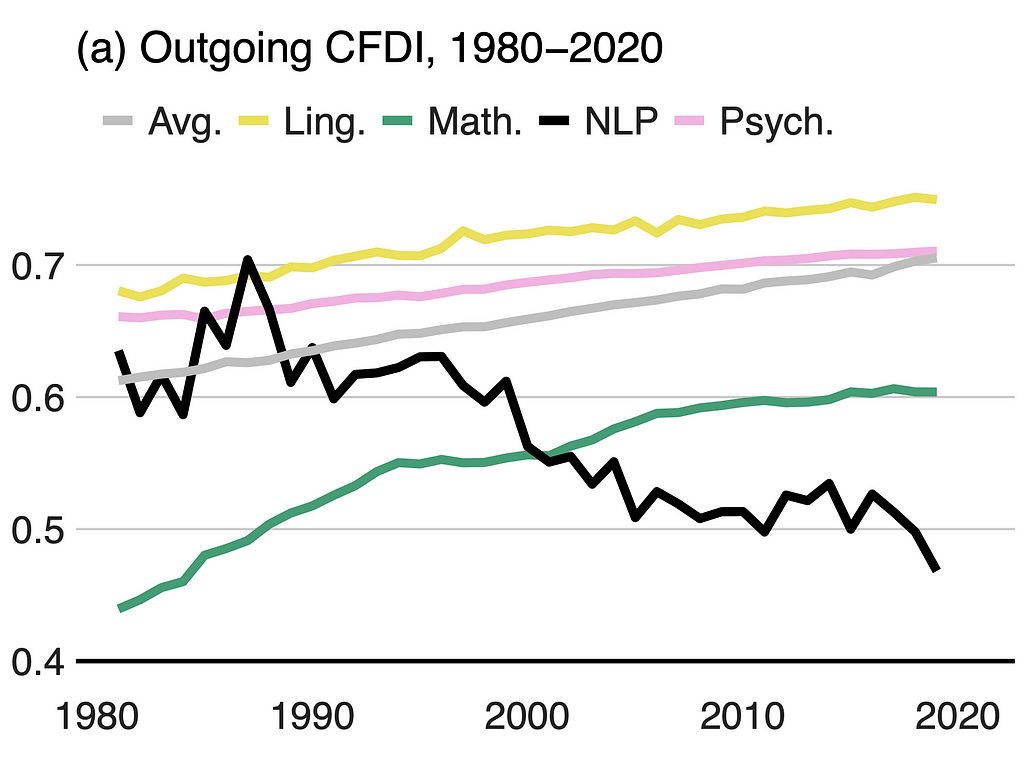
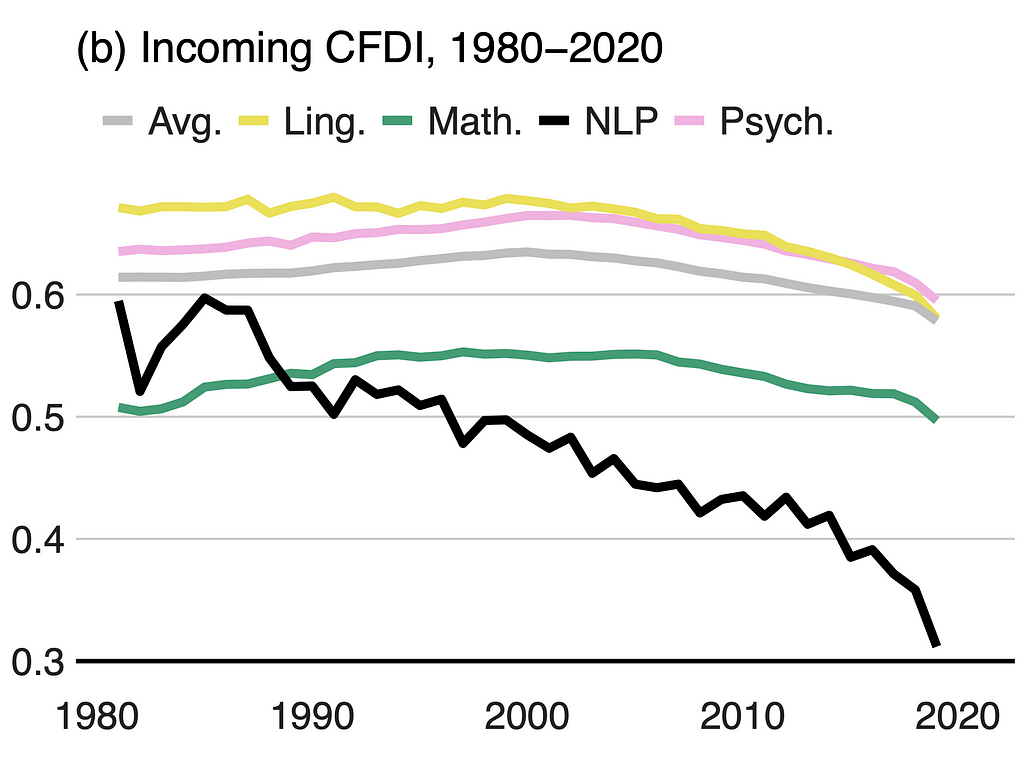
While the average outgoing CFDI has slowly increased with time, NLP has experienced a swift decline over the last four decades. The average outgoing CFDI has grown by 0.08, while NLP’s outgoing CFDI declined by about 30% (0.18) from 1980–2020. CFDI for linguistics and psychology follow a similar trend to the average, while math has experienced a large increase in outgoing CFDI of 0.16 over four decades.
Similar to outgoing CFDI, NLP papers also have a marked decline in incoming CFDI over time, indicating that incoming citations are coming mainly from one (or few fields) instead of many. Figure 5 (b) shows the plot. While the incoming CFDIs for other fields, and the average, remained steady from 1980 to 2010, NLP’s incoming CFDI has decreased from 0.59 to 0.42. In the 2010s, all fields declined in CFDI, but NLP had a particularly strong fall (0.42 to 0.31).
Key Takeaways
- NLP draws mainly from ideas within CS (>80%). After CS, NLP is most influenced by linguistics, mathematics, psychology, and sociology
- Over time, NLP’s citations to non-CS fields and its diversity in citing different fields decreased while other fields have remained steady
- Papers in NLP are getting less interdisciplinary, increasingly residing in only one field and citing their own field
DISCUSSION. A key point about scientific influence is that, as researchers, we are not just passive objects of influence. Whereas some influences are hard to ignore (say, because of substantial buy-in from one’s peers and the review process), others are a direct result of active engagement by the researcher with relevant literature (possibly from a different field).
We (can) choose whose literature to engage with and thus benefit from.
Similarly, while at times we may happen to influence other fields, we (can) choose to engage with other fields so that they are more aware of our work.
Such an engagement can be through cross-field exchanges at conferences, blog posts about one’s work for a target audience in another field, etc.
Over the last five years, NLP technologies have been widely deployed, impacting billions of people directly and indirectly. Numerous instances have also surfaced where it is clear that adequate thought was not given to developing those systems, leading to various adverse outcomes. It has also been well-established that a crucial ingredient in developing better systems is to engage with a wide range of literature and ideas, especially bringing in ideas from outside of CS (say, psychology, social science, linguistics, etc.)
Against this backdrop, the picture of NLP’s striking lack of engagement with the broader research literature — especially when marginalized communities continue to face substantial risks from its technologies — is an indictment of the field of NLP.
Not only are we not engaging with outside literature more than the average field or even just the same as other fields, but our engagement is, in fact, markedly less. A trend that is only getting worse.
THE GOOD NEWS is that this can change. It is a myth to believe that citation patterns are “meant to happen” or that individual researchers and teams do not have a choice in what they cite. We can actively choose what literature we engage with. We can work harder to tie our work with ideas in linguistics, psychology, social science, and beyond. Using language and computation, we can work more on problems that matter to other fields. By keeping an eye on work in other fields, we can bring their ideas to new compelling forms in NLP.
As researchers, mentors, reviewers, and committee members, we should ask ourselves, how are we:
influencing the broader exchange of ideas across fields
and consciously acting against:
focusing excessively on CS works at the expense of relevant work from other fields, such as psychology, sociology, and linguistics.
Epilogue
Online Tool to Calculate Cross-Field Influence
To facilitate reflection on the papers we cite from various fields, we created an online public tool to compute the amount of cross-field citations for individual papers and even sets of papers (e.g., author profiles, proceedings). Insert a Semantic Scholar or ACL Anthology URL, and the tool computes various metrics such as the most cited fields, the citation field diversity, and so on. Or upload a PDF draft, and the bibliography will be parsed and linked to their corresponding fields.
You will be able to answer questions such as:
- Which fields influence me (as an author) the most?
- What is the field diversity of my submission draft?
- What are the most relevant fields to this particular proceeding?
By answering these questions, you may find yourself reflecting further:
- “Are there ideas rooted in other fields that can be used innovatively in my research?”
- “Can I expand my literature search to include works from other fields?”
For a quick introduction to the tool, see this short video.
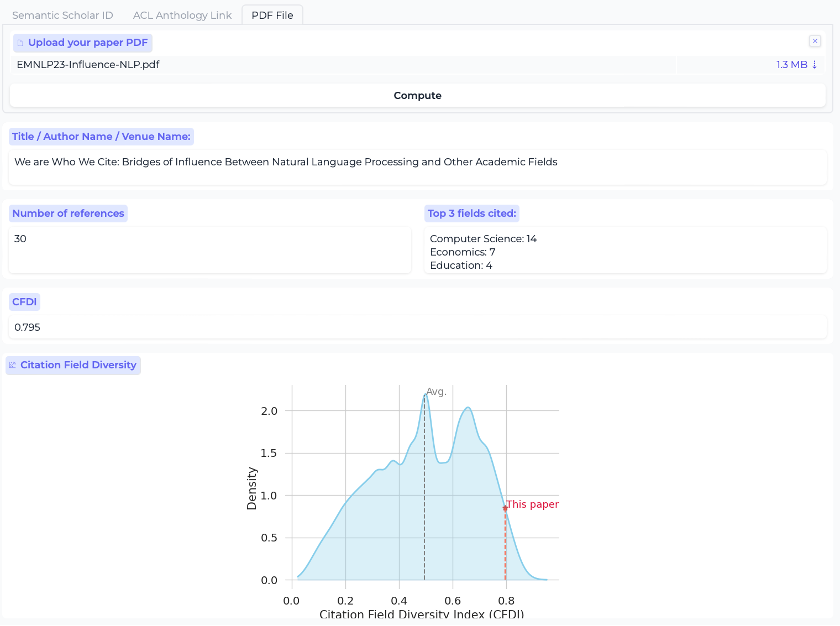
Acknowledgments
This work was partially supported by the DAAD (German Academic Exchange Service) under grant no. 9187215, the Lower Saxony Ministry of Science and Culture, and the VW Foundation. Many thanks to Roland Kuhn, Andreas Stephan, Annika Schulte-Hürmann, and Tara Small for their thoughtful discussions.
Unless otherwise noted, all images are by the author.
Related Works of Interest
- Geographic Citation Gaps in NLP (EMNLP, 2022)
- D3: A Massive Dataset of Scholarly Metadata for Analyzing the State of Computer Science Research (LREC, 2022)
- Forgotten Knowledge: Examining the Citational Amnesia in NLP (ACL, 2023)
- The Elephant in the Room: Analyzing the Presence of Big Tech in NLP Research (ACL, 2023)
- We are Who We Cite: Bridges of Influence Between Natural Language
Processing and Other Academic Fields (EMNLP, 2023)
Jan Philip Wahle
Research Staff, University of Göttingen, Germany
Visiting Researcher, National Research Council, Canada
Twitter: @jpwahle
Webpage: https://jpwahle.com/
Saif M. Mohammad
Senior Research Scientist, National Research Council Canada
Twitter: @saifmmohammad
Webpage: http://saifmohammad.com/
Examining the Influence Between NLP and Other Fields of Study was originally published in Towards Data Science on Medium, where people are continuing the conversation by highlighting and responding to this story.