Trends that will shape the Modern Data Stack in 2024
Gen AI, Data Mesh, Regulation and Observability. 2024 is going to be a fun year!
2023 will forever be remembered as the year of Generative AI. In this digital age, you’d be hard-pressed to find someone with internet access who hasn’t heard of ChatGPT. If you have been around long enough to witness a technology cycle or two, you know that we are entering quite a transformative one. According to a McKinsey survey, AI adoption has doubled since 2017. While the recent developments will undoubtedly accelerate the adoption further, I tend to believe that the results we will see in 2024 will remain relatively small as most companies are still trying to figure out how to align data strategy with business objectives while also navigating the increasing regulatory scrutiny. As far as the data industry is concerned, AI adoption will drive further data adoption by making data and the data infrastructure more accessible to broader users within the organization, making the case for more AI projects. Secure democratization of the data will be a big topic; we will see more practical implementations of the data mesh and more investment toward security, privacy, and observability.
The purpose of this article is not to make any bold statements about how AI will change the data industry as we know it but rather to shed light on some areas where we are likely to see continued investments from enterprises and the enthusiasm around Data & AI becoming a self-fulfilling prophecy.
AI will be put to WORK, and shake the Modern Data Stack as we know it.
Of course, we start here. There is no denying that LLMs have entirely altered the way we think about and do technology, and the data & analytics space is no exception. As far as the Modern data stack goes, here are some areas where LLMs are going to change the game:
Data Analytics: Introducing AI in analytics workflows will increase automation, efficiency, and accessibility.
- Automation: AI can be used to automate tedious tasks such as data collection, preparation, and cleansing and reduce the likelihood of manual errors.
- Efficiency: The use of more sophisticated predictive models will allow companies to anticipate future trends and increase the accuracy of their forecasts. AI algorithms can be utilized to identify and study customer behavior, allowing for highly personalized product recommendations and more targeted marketing campaigns.
- Accessibility: AI will help AI adoption. NLP (Natural Language Processing) can be leveraged to make AI-powered data analytics more accessible by allowing even the least technical users to interact with data in a conversational manner.
Vector Databases on the rise: LLMs require infrastructure that allows for fast querying and high processing speed of vast volumes of data, both structured and unstructured (schema-less). This is where the mathematical concept of vector and vector search databases come into the picture. Instead of rows and columns (in the case of traditional relational databases), data is represented in a multidimensional space typical of a vector representation in mathematics. In the context of a Gen AI application, vector databases allow for fast processing and querying of vectorized data. More here and here.
“Imagine a vector database as a vast warehouse and artificial intelligence as the skilled warehouse manager. In this warehouse, every item (data) is stored in a box (vector), organized neatly on shelves in multidimensional space,” as stated by Mark Hinkle in The New Stack
The “ML pipeline”
In traditional data engineering, a data pipeline is the process by which data is moved from source to destination, typically to make it accessible to the business through BI for reporting and analytics. The ML pipeline is similar to the traditional data pipeline in the sense that it is also a process of data movement; however, its primary purpose is to enable the process of developing and deploying machine learning models, and in that sense, unlike the data pipeline, the ML pipeline is not a “straight line” — more on the differences between data and ML pipelines here and here.
Successful ML, AI, and Data Science projects will require robust infrastructure that will allow for building, testing, training, optimizing, and maintaining the accuracy of the models. It starts with well-structured ML pipelines.

Privacy, please.
There is no denying that data usage and, as a result, companies’ need for democratization of both the data and the platform will continue to grow massively in 2024. That said, as both Data & AI get more regulated, the scrutiny around personal data protection policies will increase. Great summary of what to expect as far as AI regulation goes for the next 12 months here.
BYODM: Bring Your Own Data Mesh
Since it was first introduced by its creator Zhamak in 2019, the data mesh has been subject to numerous debates and a fair share of skepticism. Four years later, several implementations and variations emerged where companies embraced the principles of the concept and applied them to their architecture. Decentralization, domain-oriented design, IaaS, data as a product, and end-to-end federated governance are all great principles organizations should embrace to create and foster a silo-free, democratized data environment. However, moving from a traditional monolithic structure to a full data mesh is not easy and requires significant cultural and organizational change. This is why a gradual adoption that allows to slowly introduce the concept and prove its value while aligning existing and future technology and business considerations is what we have seen most work over the last couple of years.
Ultimately, it is essential to remember that the Data Mesh is an architectural and organizational shift, not a technology solution. I think the BYODM approach will prevail in 2024.
Data & AI Observability
I am biased here. That said, it is hard to argue against the case for Data & AI Observability in a world where every organization is thinking about the potential of LLMs.
“There’s no AI strategy without a data strategy. The intelligence we’re all aiming for results in the data” Frank Slootman.
Over the past couple of years, Data Observability has become a key component in every modern organization’s data strategy. If you are new to the concept, I recommend you start here or here. There is no denying that AI will also reshape the Data Observability space. Adopting AI agents and using NLP will increase the level of automation and inclusivity of the platform solutions, which in turn will propel the adoption. The concept of Data Observability, as we know it, will evolve to capture the potential of AI in observability and cover more AI use cases.
Most of the available solutions on the market already cover a few aspects of what will become Data & AI Observability. If you look at data science as a data consumption use case, monitoring the data that goes into model training is already covered under most frameworks. The future of Data & AI Observability will evolve to include insights into ML model’s behavior, output, and performance. Like how data pipelines are covered today, Data Observability platforms will include actionable insights on ML pipelines to allow for effective anomaly detection, root cause analysis, and incident management and bring reliability and efficiency to ML product deployment.
Conclusion
2024 is a leap year, which means we have 366 opportunities to do more and create innovation with data. Although 2023 will forever be remembered as the year of Gen AI, 2024 is when we will start seeing organizations working toward Data & AI maturity. But to do AI right, a well-thought-out data strategy is instrumental. The Modern Data Stack is an ever-evolving space, and in 2024, we will see more innovation brought by and catalyzed by the growing adoption of AI. As companies experiment more with AI in 2024, governance and observability will take center stage to ensure smooth and efficient deployments.
Trends that will shape the Modern Data Stack in 2024 was originally published in Towards Data Science on Medium, where people are continuing the conversation by highlighting and responding to this story.
Gen AI, Data Mesh, Regulation and Observability. 2024 is going to be a fun year!
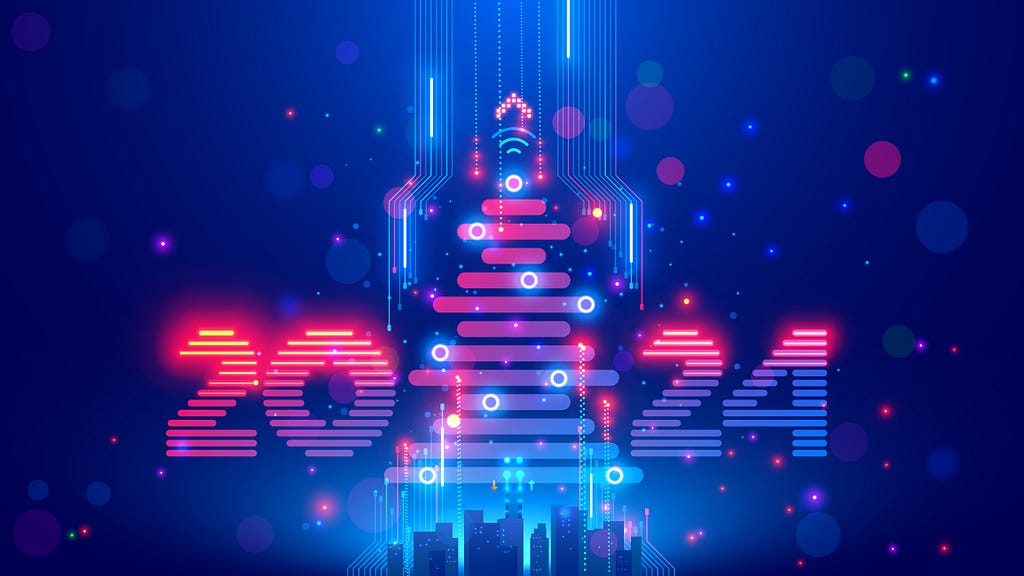
2023 will forever be remembered as the year of Generative AI. In this digital age, you’d be hard-pressed to find someone with internet access who hasn’t heard of ChatGPT. If you have been around long enough to witness a technology cycle or two, you know that we are entering quite a transformative one. According to a McKinsey survey, AI adoption has doubled since 2017. While the recent developments will undoubtedly accelerate the adoption further, I tend to believe that the results we will see in 2024 will remain relatively small as most companies are still trying to figure out how to align data strategy with business objectives while also navigating the increasing regulatory scrutiny. As far as the data industry is concerned, AI adoption will drive further data adoption by making data and the data infrastructure more accessible to broader users within the organization, making the case for more AI projects. Secure democratization of the data will be a big topic; we will see more practical implementations of the data mesh and more investment toward security, privacy, and observability.
The purpose of this article is not to make any bold statements about how AI will change the data industry as we know it but rather to shed light on some areas where we are likely to see continued investments from enterprises and the enthusiasm around Data & AI becoming a self-fulfilling prophecy.
AI will be put to WORK, and shake the Modern Data Stack as we know it.
Of course, we start here. There is no denying that LLMs have entirely altered the way we think about and do technology, and the data & analytics space is no exception. As far as the Modern data stack goes, here are some areas where LLMs are going to change the game:
Data Analytics: Introducing AI in analytics workflows will increase automation, efficiency, and accessibility.
- Automation: AI can be used to automate tedious tasks such as data collection, preparation, and cleansing and reduce the likelihood of manual errors.
- Efficiency: The use of more sophisticated predictive models will allow companies to anticipate future trends and increase the accuracy of their forecasts. AI algorithms can be utilized to identify and study customer behavior, allowing for highly personalized product recommendations and more targeted marketing campaigns.
- Accessibility: AI will help AI adoption. NLP (Natural Language Processing) can be leveraged to make AI-powered data analytics more accessible by allowing even the least technical users to interact with data in a conversational manner.
Vector Databases on the rise: LLMs require infrastructure that allows for fast querying and high processing speed of vast volumes of data, both structured and unstructured (schema-less). This is where the mathematical concept of vector and vector search databases come into the picture. Instead of rows and columns (in the case of traditional relational databases), data is represented in a multidimensional space typical of a vector representation in mathematics. In the context of a Gen AI application, vector databases allow for fast processing and querying of vectorized data. More here and here.
“Imagine a vector database as a vast warehouse and artificial intelligence as the skilled warehouse manager. In this warehouse, every item (data) is stored in a box (vector), organized neatly on shelves in multidimensional space,” as stated by Mark Hinkle in The New Stack
The “ML pipeline”
In traditional data engineering, a data pipeline is the process by which data is moved from source to destination, typically to make it accessible to the business through BI for reporting and analytics. The ML pipeline is similar to the traditional data pipeline in the sense that it is also a process of data movement; however, its primary purpose is to enable the process of developing and deploying machine learning models, and in that sense, unlike the data pipeline, the ML pipeline is not a “straight line” — more on the differences between data and ML pipelines here and here.
Successful ML, AI, and Data Science projects will require robust infrastructure that will allow for building, testing, training, optimizing, and maintaining the accuracy of the models. It starts with well-structured ML pipelines.

Privacy, please.
There is no denying that data usage and, as a result, companies’ need for democratization of both the data and the platform will continue to grow massively in 2024. That said, as both Data & AI get more regulated, the scrutiny around personal data protection policies will increase. Great summary of what to expect as far as AI regulation goes for the next 12 months here.
BYODM: Bring Your Own Data Mesh
Since it was first introduced by its creator Zhamak in 2019, the data mesh has been subject to numerous debates and a fair share of skepticism. Four years later, several implementations and variations emerged where companies embraced the principles of the concept and applied them to their architecture. Decentralization, domain-oriented design, IaaS, data as a product, and end-to-end federated governance are all great principles organizations should embrace to create and foster a silo-free, democratized data environment. However, moving from a traditional monolithic structure to a full data mesh is not easy and requires significant cultural and organizational change. This is why a gradual adoption that allows to slowly introduce the concept and prove its value while aligning existing and future technology and business considerations is what we have seen most work over the last couple of years.
Ultimately, it is essential to remember that the Data Mesh is an architectural and organizational shift, not a technology solution. I think the BYODM approach will prevail in 2024.
Data & AI Observability
I am biased here. That said, it is hard to argue against the case for Data & AI Observability in a world where every organization is thinking about the potential of LLMs.
“There’s no AI strategy without a data strategy. The intelligence we’re all aiming for results in the data” Frank Slootman.
Over the past couple of years, Data Observability has become a key component in every modern organization’s data strategy. If you are new to the concept, I recommend you start here or here. There is no denying that AI will also reshape the Data Observability space. Adopting AI agents and using NLP will increase the level of automation and inclusivity of the platform solutions, which in turn will propel the adoption. The concept of Data Observability, as we know it, will evolve to capture the potential of AI in observability and cover more AI use cases.
Most of the available solutions on the market already cover a few aspects of what will become Data & AI Observability. If you look at data science as a data consumption use case, monitoring the data that goes into model training is already covered under most frameworks. The future of Data & AI Observability will evolve to include insights into ML model’s behavior, output, and performance. Like how data pipelines are covered today, Data Observability platforms will include actionable insights on ML pipelines to allow for effective anomaly detection, root cause analysis, and incident management and bring reliability and efficiency to ML product deployment.
Conclusion
2024 is a leap year, which means we have 366 opportunities to do more and create innovation with data. Although 2023 will forever be remembered as the year of Gen AI, 2024 is when we will start seeing organizations working toward Data & AI maturity. But to do AI right, a well-thought-out data strategy is instrumental. The Modern Data Stack is an ever-evolving space, and in 2024, we will see more innovation brought by and catalyzed by the growing adoption of AI. As companies experiment more with AI in 2024, governance and observability will take center stage to ensure smooth and efficient deployments.
Trends that will shape the Modern Data Stack in 2024 was originally published in Towards Data Science on Medium, where people are continuing the conversation by highlighting and responding to this story.