Twelve HR-Ready Roles to Help Build Healthy Data Science and AI Teams | by Jason Tamara Widjaja | Jun, 2022
Data Science Dysfunctions
Put those data science Venn diagrams away. Yes all thirteen of them.
The ongoing great resignation that started in 2021, while gathering steam and backed by credible data, is nothing new in data science.
Today is not about running explainability on why people are quitting. (We did this and bravely published our thoughts on how we think this can be done with empathy and governance). Rather today I want to focus on one key driver of dysfunctional data science teams — management mistyping what data scientists should and should not do.
This particular dysfunction leads to multiple failure modes, including:
- Data scientists and analysts being pressed into building full stack applications instead of working with software engineering (in fact multiple roles in software engineering).
- Data scientists taking responsibility for data management, reporting and building dashboards (instead of working with professional data engineering and business intelligence teams).
- AI researchers spending increasing chunks of time deploying and monitoring models once projects are complete (this is closer to the work of machine learning engineers, and MLOps teams rather than researchers).
- Data science being conflated with artificial intelligence (AI methods can be used for insights, AI itself is a different discipline and has a different focus).
This is not to say that you need to hire four teams to model a table from a relational database. All data teams start somewhere, and in smaller companies multitasking is an absolute necessity.
Nor is this saying that data scientists are incapable of doing these tasks. A growth mindset is great, and all roles benefit from understanding the work of adjacent teams. But just because some data scientists can do a task does not mean it should be a part of core data science responsibilities as a general rule.
Data scientists often stretch and play multiple roles, doing whatever is necessary to hold things together. But this is a failure mode in itself — because it ironically causes management to think things are working well so all they need is… more data scientists. Thus perpetuating the dysfunction.
So, how do we avoid this?
Better, precise job titles.
This aligns candidate expectations, saves hiring manager time, and might even save your company being the subject of data science job bait and switch threads.
But before we get too far ahead, we need to talk about the data science Venn diagram.
Beyond Data Science Venn Diagrams
When someone mentions the data science Venn Diagram, a reasonable question would be “which one”?
At last count there are at least 13 data science Venn diagrams which are variations of Drew Conway’s original. (A big thank you to KDNuggets for bringing the list together.)
I have much respect to Drew Conway for sharing his ideas — it is easy to point out how something is not precisely right for me today while forgetting the state of the world before the idea was shared. His initial ideas are a great landmark to take reference from, and still applies today.
But the field has matured, and the problem we need to tackle is not just understanding the role of the data scientist, but giving equal attention to the rest of the data roles that complement data science. And appreciating that these are established and equal professions on their own.
Data Science Career Pathways from the World’s most AI-ready city
A few years ago I banded with my fellow team leads in data and AI to try tackling this problem. Serendipitously, what started as a small team effort ended up pulling us into a national role definition exercise in Singapore where many of us were based. Our work eventually expanded into defining twelve data, analytics, data science and AI roles.
These are published in the framework below.
Importantly, the target was equipping companies to build data and AI teams. And as such these roles are HR ready, and you will find the full package of:
- Role descriptions,
- Taxonomies of technical skills and soft skills,
- Calibrated levels of each skill for each role, and
- Definitions for each level of skill.
In the same period I was encouraged to hear that Singapore’s growth trajectory as an AI hub was supported by winning three awards by the Oliver Wyman Forum, Oxford Insights and the International Development Research Centre, and the IMD that currently put it as the world’s most AI-ready city. (As a data scientist I am perennially curious about the inner workings of rankings, so I dug a bit deeper and found through Stanford HAI’s global AI vibrancy tool that Singapore’s success was likely calculated on a per-capita basis.)
Regardless of awards, the framework is practically useful and now represents one of the most up to date sources for career pathways for data and AI professionals.
A Skills Framework for Data and AI Careers

There are Twelve Distinct Roles in Data and AI
These distinct roles are listed below. They are split into five role ‘families’, and the y axis roughly denotes seniority within the family:
- Data Analyst/Associate Data Engineer
- Business Intelligence Manager
- Business Intelligence Director
- Data Engineer
- Senior Data Engineer
- Data Architect
- Artificial Intelligence/Machine Learning Engineer
- Senior Artificial Intelligence/Machine Learning Engineer
- Data Scientist/Artificial Intelligence Scientist
- Artificial Intelligence Applied Researcher
- Head of Data Science and Artificial Intelligence
- Chief Data Officer/Chief Artificial Intelligence Officer
Rather than repeat the content on the site, a more useful framing might be to take a lens of someone new to data and AI careers.
We wanted to help new entrants to data, analytics and AI answer the question: which data role would I best thrive in?
And to answer this question, I propose four specific cuts of the data:

Business Intelligence is an established field with its own career path.
Business intelligence (“BI”) is an established field with many overlaps with data science. It includes identifying business needs, preparing and analysing data and presenting insights. However, these alone does not mean it should be subsumed into data science.
BI requires a mix of skills that are simultaneously close to the customer, close to the data, and close to the product or platform of choice. These skills include data analytics, data engineering, data governance, data visualization and stakeholder engagement – with full list accessible via the skills framework site here.
Equally important is what is missing — while advancement in BI mean that many modern BI tools now offer some predictive capability, responsibility for modelling is not on their required skill set.
A particularly interesting trait is also how every BI team we polled built their practice around a small number of commercial BI tools.
BI is its own distinct career pathway and it may come as a surprise to some data scientists that even now BI professionals and jobs often outnumber data science ones.
The question that provides the most information on a job is often about whether you produce insights or products.
If I was interviewing for a job and could only ask one question to get information on a data science role, I would not ask whether the job requires deep learning, operates in a devops environment, or requires knowledge of causality.
The single question that provides me the most information of your data science role is: “is your output primarily insights or products?”
Or asked in a slightly different way, is the output of your role primarily consumed by humans, or by machines?
To the extent the answer is ‘insights’, you drift towards the right of the framework , and will likely be more ‘scientist’ than ‘engineer’. This is where you double down on understanding your business problem domain, require careful inference skills, and channel communication skills to help your stakeholders to do the same. You would also benefit from sitting as close as you can to the decision makers you are trying to support.
Conversely to the extent the answer is ‘products’, you drift towards the left and towards the ‘engineer’ persona. You might be ‘closer to IT’, benefit greatly from an understanding of application development and integration, and will likely operate in agile product or delivery teams.
There are specific roles where PhDs are mandatory. But they are relatively few and far between.
The third question is one that often comes up when I speak to graduates – whether one requires a PhD to be a data scientist. In recent years, this has shifted slightly to ‘would having a PhD help my career’.
While the specifics of your situation is the most important, it is worth mentioning that there is a specific set of roles that benefit greatly from a PhD. These are often in machine learning or a specific subfield of artificial intelligence (such as image processing or NLP).
These fall under the AI Applied Researcher role, and are often marked by the R&D nature of their work, publishing papers as part of their work scope, and ability to produce patentable AI solutions.
Entry level applicants should be aware that analyst roles have multiple meanings.
If you are just switching into data and your offer letter says ‘data analyst’, that can be an unhelpful term(!).
This is because the ‘data analyst’ role is used multiple different job families, and your experience will be significantly different depending on which family the role sits in.
One interpretation of a data analyst is the role that analyses data for insights, and the other is the role that analyses data for engineering.
To further complicate things, both use the term modelling. But when a data scientist mentions modelling, it often implies fitting curves to distributions and building representations of the data for a predictive or inference task. But when a data engineer mentions modelling, this use is associated to data structures and schemas to store, manage and perform operations on data.
Whichever variation you find yourself in, know who you are and I trust you will become a model employee (sorry).
Closing thoughts: Building Your Best Team!
Going from data scientist to manager, director and beyond requires new roadmaps for the new personas and roles in your expanded teams. We did this so you don’t have to, so feel free to leverage and adapt this framework to your needs. And please do not hesitate to reach out if you have questions, feedback, or just need a sounding board in building out your team.
Now if you are a manager and you just hired an econometrician to ‘build your data fabric so you can do predictive analytics on top’, or hired a ‘BI developer’ to optimize your marketing spend because ‘you want the results on a dashboard’, you may want read this article again. But go take a walk in a random forest first.
Data Science Dysfunctions
Put those data science Venn diagrams away. Yes all thirteen of them.
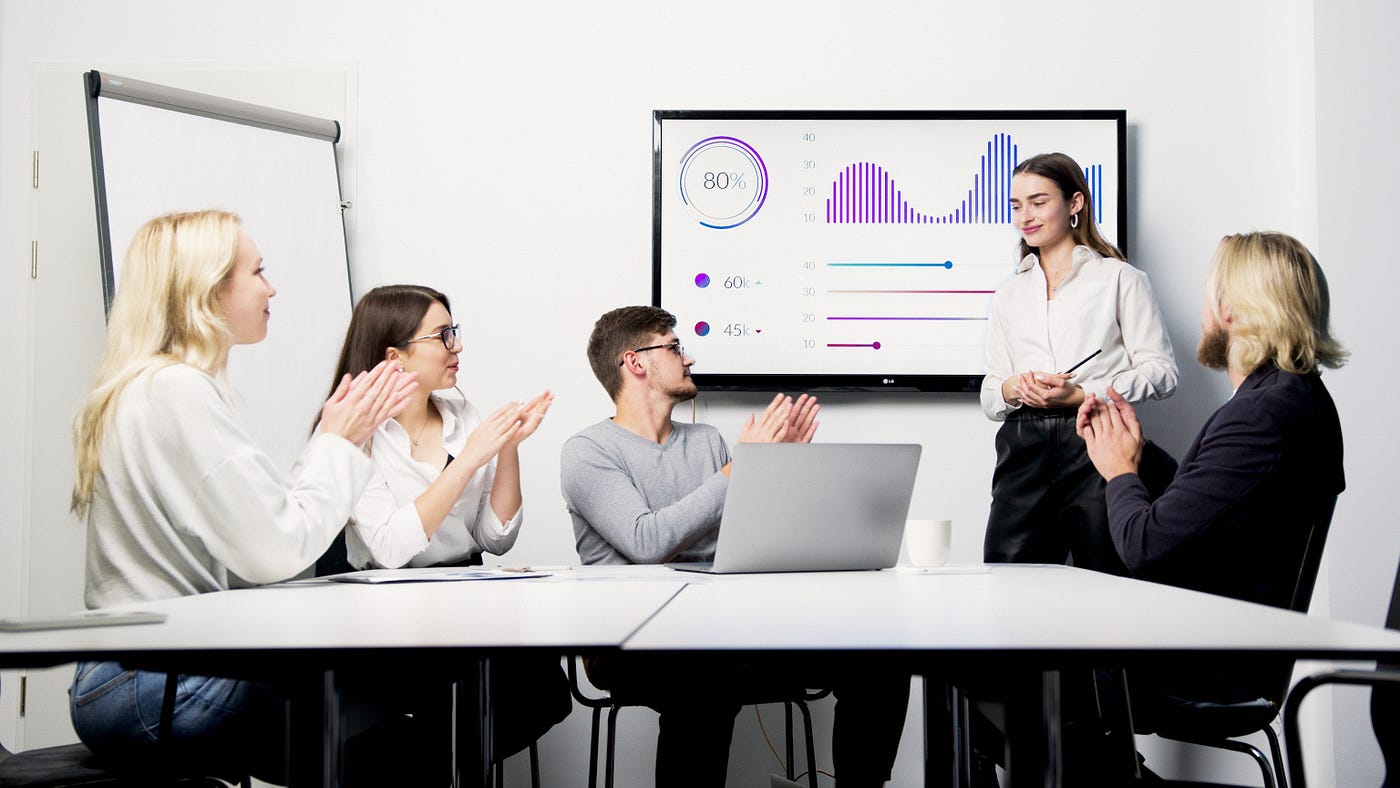
The ongoing great resignation that started in 2021, while gathering steam and backed by credible data, is nothing new in data science.
Today is not about running explainability on why people are quitting. (We did this and bravely published our thoughts on how we think this can be done with empathy and governance). Rather today I want to focus on one key driver of dysfunctional data science teams — management mistyping what data scientists should and should not do.
This particular dysfunction leads to multiple failure modes, including:
- Data scientists and analysts being pressed into building full stack applications instead of working with software engineering (in fact multiple roles in software engineering).
- Data scientists taking responsibility for data management, reporting and building dashboards (instead of working with professional data engineering and business intelligence teams).
- AI researchers spending increasing chunks of time deploying and monitoring models once projects are complete (this is closer to the work of machine learning engineers, and MLOps teams rather than researchers).
- Data science being conflated with artificial intelligence (AI methods can be used for insights, AI itself is a different discipline and has a different focus).
This is not to say that you need to hire four teams to model a table from a relational database. All data teams start somewhere, and in smaller companies multitasking is an absolute necessity.
Nor is this saying that data scientists are incapable of doing these tasks. A growth mindset is great, and all roles benefit from understanding the work of adjacent teams. But just because some data scientists can do a task does not mean it should be a part of core data science responsibilities as a general rule.
Data scientists often stretch and play multiple roles, doing whatever is necessary to hold things together. But this is a failure mode in itself — because it ironically causes management to think things are working well so all they need is… more data scientists. Thus perpetuating the dysfunction.
So, how do we avoid this?
Better, precise job titles.
This aligns candidate expectations, saves hiring manager time, and might even save your company being the subject of data science job bait and switch threads.
But before we get too far ahead, we need to talk about the data science Venn diagram.
Beyond Data Science Venn Diagrams
When someone mentions the data science Venn Diagram, a reasonable question would be “which one”?
At last count there are at least 13 data science Venn diagrams which are variations of Drew Conway’s original. (A big thank you to KDNuggets for bringing the list together.)
I have much respect to Drew Conway for sharing his ideas — it is easy to point out how something is not precisely right for me today while forgetting the state of the world before the idea was shared. His initial ideas are a great landmark to take reference from, and still applies today.
But the field has matured, and the problem we need to tackle is not just understanding the role of the data scientist, but giving equal attention to the rest of the data roles that complement data science. And appreciating that these are established and equal professions on their own.
Data Science Career Pathways from the World’s most AI-ready city
A few years ago I banded with my fellow team leads in data and AI to try tackling this problem. Serendipitously, what started as a small team effort ended up pulling us into a national role definition exercise in Singapore where many of us were based. Our work eventually expanded into defining twelve data, analytics, data science and AI roles.
These are published in the framework below.
Importantly, the target was equipping companies to build data and AI teams. And as such these roles are HR ready, and you will find the full package of:
- Role descriptions,
- Taxonomies of technical skills and soft skills,
- Calibrated levels of each skill for each role, and
- Definitions for each level of skill.
In the same period I was encouraged to hear that Singapore’s growth trajectory as an AI hub was supported by winning three awards by the Oliver Wyman Forum, Oxford Insights and the International Development Research Centre, and the IMD that currently put it as the world’s most AI-ready city. (As a data scientist I am perennially curious about the inner workings of rankings, so I dug a bit deeper and found through Stanford HAI’s global AI vibrancy tool that Singapore’s success was likely calculated on a per-capita basis.)
Regardless of awards, the framework is practically useful and now represents one of the most up to date sources for career pathways for data and AI professionals.
A Skills Framework for Data and AI Careers

There are Twelve Distinct Roles in Data and AI
These distinct roles are listed below. They are split into five role ‘families’, and the y axis roughly denotes seniority within the family:
- Data Analyst/Associate Data Engineer
- Business Intelligence Manager
- Business Intelligence Director
- Data Engineer
- Senior Data Engineer
- Data Architect
- Artificial Intelligence/Machine Learning Engineer
- Senior Artificial Intelligence/Machine Learning Engineer
- Data Scientist/Artificial Intelligence Scientist
- Artificial Intelligence Applied Researcher
- Head of Data Science and Artificial Intelligence
- Chief Data Officer/Chief Artificial Intelligence Officer
Rather than repeat the content on the site, a more useful framing might be to take a lens of someone new to data and AI careers.
We wanted to help new entrants to data, analytics and AI answer the question: which data role would I best thrive in?
And to answer this question, I propose four specific cuts of the data:

Business Intelligence is an established field with its own career path.
Business intelligence (“BI”) is an established field with many overlaps with data science. It includes identifying business needs, preparing and analysing data and presenting insights. However, these alone does not mean it should be subsumed into data science.
BI requires a mix of skills that are simultaneously close to the customer, close to the data, and close to the product or platform of choice. These skills include data analytics, data engineering, data governance, data visualization and stakeholder engagement – with full list accessible via the skills framework site here.
Equally important is what is missing — while advancement in BI mean that many modern BI tools now offer some predictive capability, responsibility for modelling is not on their required skill set.
A particularly interesting trait is also how every BI team we polled built their practice around a small number of commercial BI tools.
BI is its own distinct career pathway and it may come as a surprise to some data scientists that even now BI professionals and jobs often outnumber data science ones.
The question that provides the most information on a job is often about whether you produce insights or products.
If I was interviewing for a job and could only ask one question to get information on a data science role, I would not ask whether the job requires deep learning, operates in a devops environment, or requires knowledge of causality.
The single question that provides me the most information of your data science role is: “is your output primarily insights or products?”
Or asked in a slightly different way, is the output of your role primarily consumed by humans, or by machines?
To the extent the answer is ‘insights’, you drift towards the right of the framework , and will likely be more ‘scientist’ than ‘engineer’. This is where you double down on understanding your business problem domain, require careful inference skills, and channel communication skills to help your stakeholders to do the same. You would also benefit from sitting as close as you can to the decision makers you are trying to support.
Conversely to the extent the answer is ‘products’, you drift towards the left and towards the ‘engineer’ persona. You might be ‘closer to IT’, benefit greatly from an understanding of application development and integration, and will likely operate in agile product or delivery teams.
There are specific roles where PhDs are mandatory. But they are relatively few and far between.
The third question is one that often comes up when I speak to graduates – whether one requires a PhD to be a data scientist. In recent years, this has shifted slightly to ‘would having a PhD help my career’.
While the specifics of your situation is the most important, it is worth mentioning that there is a specific set of roles that benefit greatly from a PhD. These are often in machine learning or a specific subfield of artificial intelligence (such as image processing or NLP).
These fall under the AI Applied Researcher role, and are often marked by the R&D nature of their work, publishing papers as part of their work scope, and ability to produce patentable AI solutions.
Entry level applicants should be aware that analyst roles have multiple meanings.
If you are just switching into data and your offer letter says ‘data analyst’, that can be an unhelpful term(!).
This is because the ‘data analyst’ role is used multiple different job families, and your experience will be significantly different depending on which family the role sits in.
One interpretation of a data analyst is the role that analyses data for insights, and the other is the role that analyses data for engineering.
To further complicate things, both use the term modelling. But when a data scientist mentions modelling, it often implies fitting curves to distributions and building representations of the data for a predictive or inference task. But when a data engineer mentions modelling, this use is associated to data structures and schemas to store, manage and perform operations on data.
Whichever variation you find yourself in, know who you are and I trust you will become a model employee (sorry).
Closing thoughts: Building Your Best Team!
Going from data scientist to manager, director and beyond requires new roadmaps for the new personas and roles in your expanded teams. We did this so you don’t have to, so feel free to leverage and adapt this framework to your needs. And please do not hesitate to reach out if you have questions, feedback, or just need a sounding board in building out your team.
Now if you are a manager and you just hired an econometrician to ‘build your data fabric so you can do predictive analytics on top’, or hired a ‘BI developer’ to optimize your marketing spend because ‘you want the results on a dashboard’, you may want read this article again. But go take a walk in a random forest first.