Who are chatbots (and what are they to you)?
Language processing in humans and computers: Part 1
Who are chatbots
(and what are they to you)?
Introduction
Chatbots: Shifting the paradigm of meaning
What just happened?
We live in strange times.
Stories used to be told by storytellers, poems recited by poets, music played by musicians, science taught by teachers. Then the printing and recording technologies made copying possible and the copyright got invented and the owners of the recording and printing equipment started earning more than musicians and storytellers. Then the web happened and it all came under your fingertips. Now chatbots happened and you can ask them to write poetry or to explain science, or even combine the two.
They even seem to have sparks of a sense of humor. I asked the same bot to translate a subtle metaphor from Croatian to English and she did it so well that I got an impulse to thank her in French, with “merci :)” to which she pivoted to German: “Gern geschehen! If you have any more questions, feel free to ask. ☺️”
The paradigm of meaning
Our interactions with computers evolved gradually. They have been able to maintain light conversations since the 1960s, and personal assistants conversing in human voices have been sold for a while. Not a big deal. Human appearances are in the eyes of human observers.
But with chatbots, the appearances seem to have gone beyond the eyes. When you and I chat, we assume that the same words mean the same things because we have seen the same world. If we talk about chairs, we can point to a chair. The word “chair” refers to a thing in the world. But a chatbot has never seen a chair, or anything else. For a chatbot, a word cannot possibly refer to a thing in the world, since it has no access to the world. It appears to know what it is talking about because it was trained on data downloaded from the web, which were uploaded by people who know what they are talking about. A chatbot has never seen a chair, or a tree, or a cow, or felt pain, but his chats are remixes of the chats between people who have. Chatbot’s words do not refer to things directly, but indirectly, through people’s words. The next image illustrates how this works.

When I say “cow’’, it is because I have a cow on my mind. When a chatbot says “cow”, it is because that word is a likely completion of the preceding context. Many theories of meaning have been developed in different parts of linguistics and philosophy, often subsumed under the common name semiotics. While they differ in many things, they mostly stick with the picture on the left, of meaning as a relation between a signifying token, say a written or spoken word, and a signified item, an object, or a concept. While many semioticists noted that words and other fragments of language are also referred to by words and other fragments of language, the fact that the entire process of language production can be realized within a self-contained system of references, as a whirlwind of words arising from words — as demonstrated by chatbots — is a fundamental, unforeseen challenge. According to most theories of meaning, chatbots should be impossible. Yet here they are!
Zeno and the aliens
A chatbot familiar with pre-Socratic philosophy would be tempted to compare the conundrum of meaning with the conundrum of motion, illustrated in DALL-E’s picture of Parmenides and Zeno.
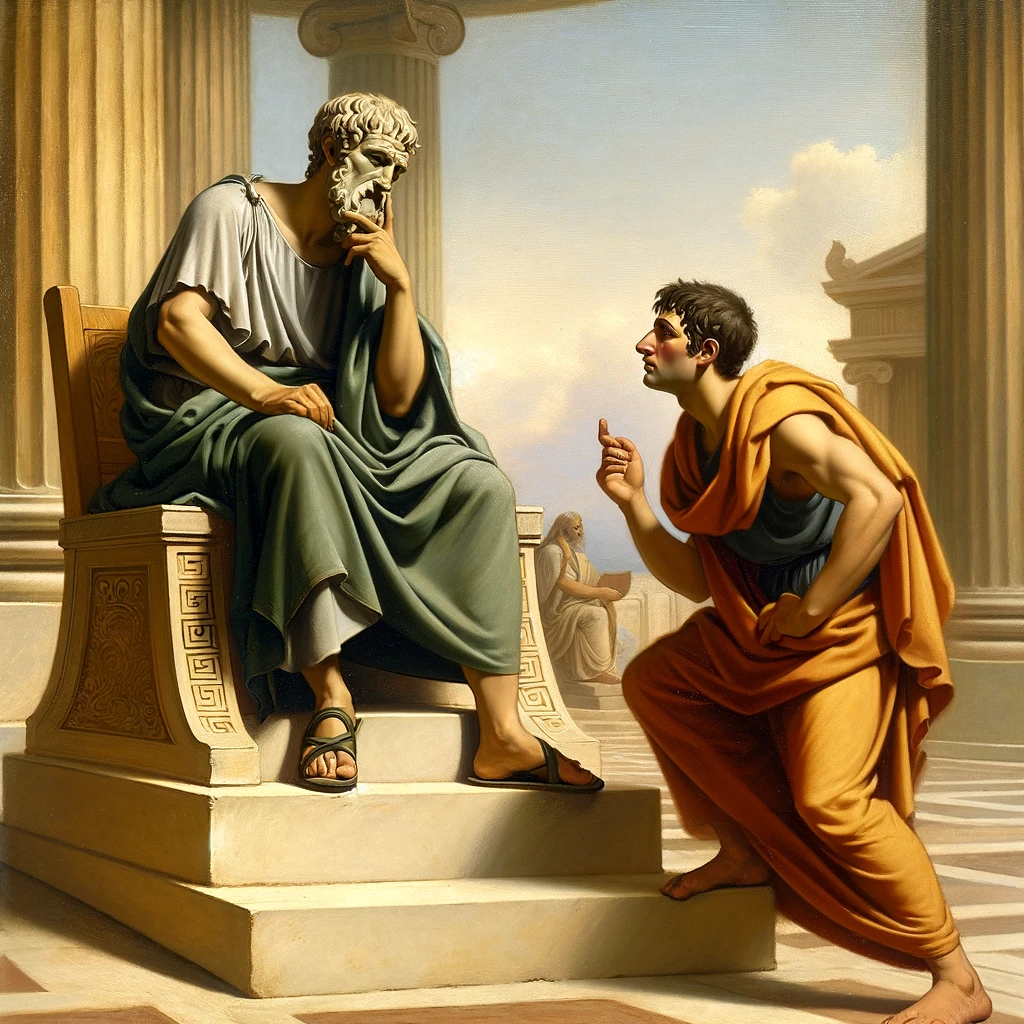
Parmenides was a leading Greek philosopher in the VI — V centuries BCE. Zeno was his most prominent pupil. To a modern eye, the illustration seems to be showing Zeno disproving Parmenides’ claims against the possibility of movement by moving around. An empiric counterexample. For all we know, Zeno did not intend to disprove his teacher’s claims and neither Parmenides nor Plato (who presented Parmenides’ philosophy in eponymous dialogue) seem to have noticed the tension between Parmenides’ denial of the possibility of movement and Zeno’s actual movement. Philosophy and the students pacing around were not viewed in the same realm. (Ironically, when the laws of motion were finally understood some 2000 years later, Parmenides’ argument, popularized in Zeno’s story about Achilles and tortoise, played an important role.)
Before you dismiss concerns about words and things and Zeno and the chatbots as a philosophical conundrum of no consequence for our projects in science and engineering, note that the self-contained language models, built and trained by chatbot researchers and their companies, could just as well be built and trained by an alien spaceship parked by the Moon. They could listen in, crawl our the websites, scrub the data, build neural networks, train them to speak on our favorite topics in perfect English, provide compelling explanations, illustrate them in vivid colors. Your friendly AI could be operated by product engineers in San Francisco, or in Pyongyang, or on the Moon. They don’t need to understand the chats to build the chatbots. That is not science fiction.
But a broad range of sci-fi scenarios opens up. There was a movie where the landing on the Moon was staged on Earth. Maybe the Moon landing was real but the last World Cup final was modified by AI. Or maybe it wasn’t modified but the losing team can easily prove that it could have been, and the winning team would have more trouble to prove that it wasn’t. Conspiracy theorists are, of course, mostly easy to recognize, but there is an underlying logical property of conspiracies worth taking note of: A large family of false statement generators are one-way functions: most of their false statements are much harder to disprove than to generate.
Without pursuing the interleaving threads of AI science and fiction very far, it seems clear that the boundaries between science and fiction, and between fiction and reality, may have been breached in ways unseen before. We live in strange times.
AI: How did we get here and where do we go?
The mind-body problem and solution
The idea of machine intelligence goes back to Alan Turing, the mathematician who defined and described the processes of computation that surround us. At the age of 19, Alan confronted the problem of mind — where it comes from and where it goes? — when he suddenly lost a friend with whom he had just fallen in love.
Some 300 years earlier, philosopher René Descartes was pondering about the human body. One of the first steps into modern science was his realization that living organisms were driven by the same physical mechanisms as the rest of nature, i.e. that they were in essence similar to the machines built at the time. One thing that he couldn’t figure out was how the human body gives rise to the human mind. He stated that as the mind-body problem.
Alan Turing essentially solved the mind-body problem. His description of computation as a process implementable in machines, and his results proving that that process can simulate our reasoning and calculations, suggested that the mind may be arising from the body as a system of computational processes. He speculated that some version of computation was implemented in our neurons. He illustrated his 1947 research report by a neural network.
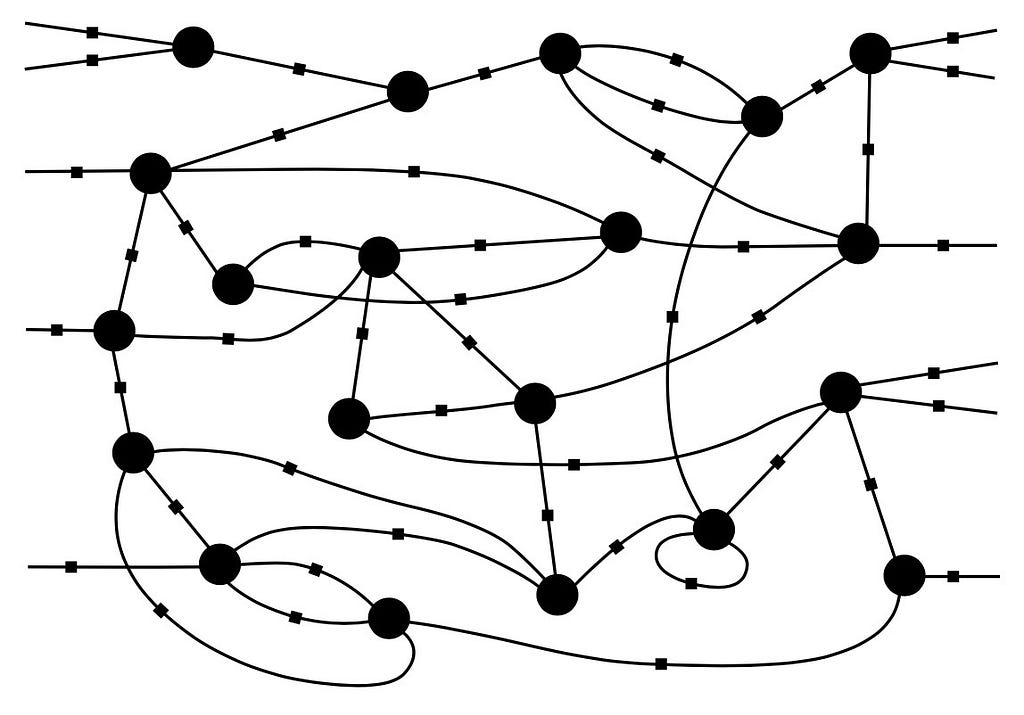
Since such computational processes are implementable in machines, it was reasonable to expect that they could give rise to machine intelligence. Turing spent several years on a futile effort to build one of the first computers, mostly driven by thoughts about the potential of intelligent machinery, and about its consequences.
Turing and Darwin
During WWII, Turing’s theoretical research was superseded by cryptanalysis at Bletchley Park. That part of the story seems well-known. When the war ended, he turned down positions at Cambridge and Princeton and accepted work at the National Physics Laboratory, hoping to build a computer and test the idea of machine intelligence. The 1947 memo contains what seems to be the first emergence of the ideas of training neural networks, and of supervised and unsupervised learning. It was so far ahead of its time that some parts seem still ahead. It was submitted to the director of the National Physics Laboratory Sir Charles G. Darwin, a grandson of Charles Darwin and a prominent eugenicist on his own account. In Sir Darwin’s opinion, Turing’s memo read like a “fanciful school-boy’s essay”. Also resenting Turing’s “smudgy’’ appearance, Sir Darwin smothered the computer project by putting it under strict administrative control. The machine intelligence memo sank into oblivion for more than 20 years. Turing devoted the final years until his death (at the age of 42, by biting into a cyanide-laced apple!) to exploring the computational aspects of life. E.g., what determines the shape of the black spots on a white cow’s hide? Life always interested him.
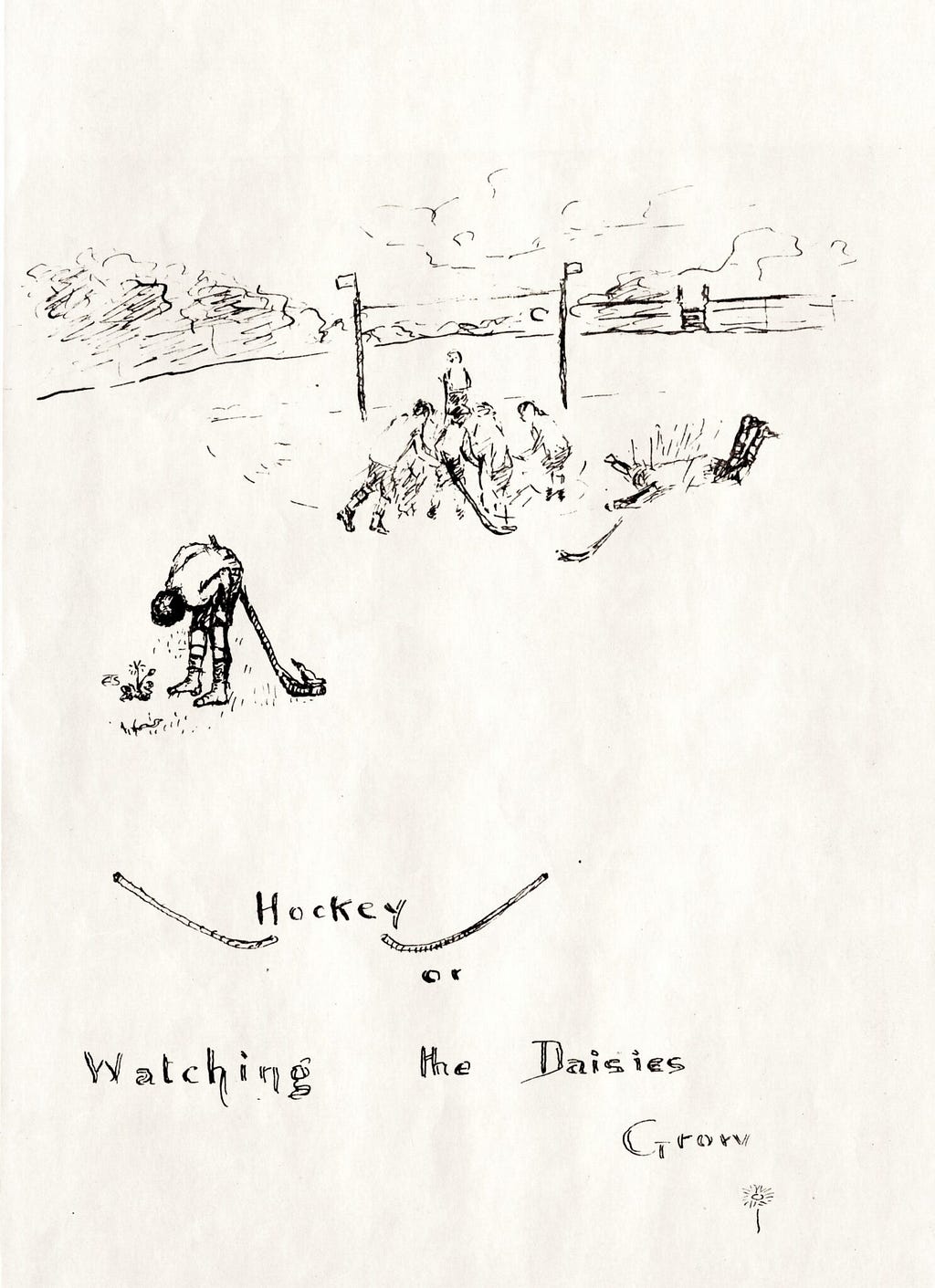
2 years after Turing’s death (9 years after the Intelligent Machinery memo), Turing’s machine intelligence got renamed to Artificial Intelligence (AI) at the legendary Dartmouth workshop.
The history of artificial intelligence is mostly a history of efforts towards intelligent design of intelligence. The main research efforts were to logically reconstruct phenomena of human behavior, like affects, emotions, common sense, etc.; and to realize them in software.
Turing, in contrast, was assuming machine intelligence would evolve spontaneously. The main published account of his thoughts on the topic appeared in the journal “Mind”. The paper opens with the question: “Can machines think?”. What we now call the Turing Test is offered as a means for deciding the answer. The idea is that a machine that can maintain a conversation and remain indistinguishable from a thinking human being must be recognized as a thinking machine. At this time of confusion around chatbots, the closing paragraph of the “Mind” paper seems particularly interesting:
An important feature of a learning machine is that its teacher will often be very largely ignorant of quite what is going on inside, although he may still be able to some extent to predict his pupil’s behavior. […] This is in clear contrast with a normal procedure when using a machine to do computations: one’s object is then to have a clear mental picture of the state of the machine at each moment in the computation. This object can only be achieved with a struggle. The view that `the machine can only do what we know how to order it to do’, appears strange in the face of this fact. Intelligent behaviour presumably consists in a departure from the completely disciplined behaviour.
Designers and builders of chatbots and language engines published many accounts of their systems’ methods and architectures but seem as stumped as everyone by their behaviors. Some initially denied the unexpected behaviors, but then stopped working and started talking about a threat to humanity. Turing anticipated the unexpected behaviors. His broader message seems to be that not knowing what is on the mind of another intelligent entity is not a bug but a feature of intelligence. That is why intelligent entities communicate. Failing that, they view each other as an object. Understanding chatbots may require broadening our moral horizons.
From search engines to language models
One thing that Turing didn’t get right was how intelligent machines would learn to reason. He imagined that they would need to learn from teachers. He didn’t predict the Web. The Web provided the space to upload the human mind. The language models behind chatbots are
- not a result of intelligent design of artificial intelligence,
- but an effect of spontaneous evolution of the Web.
Like search engines, language models are fed data scraped from the Web by crawlers. A search engine builds an index to serve links based on rankings (pulled from data or pushed by sponsors), whereas a language engine builds a model to predict continuations of text based on context references. The computations evolve, but the proportions that are being computed remain the same:

What does the Web past say about the AI future?
The space of language, computation, networks, and AI has many dimensions. The notion of mind has many definitions. If we take into account that our mind depends on language, computation, networks, and AI as its tools and extensions, just like music depends on instruments, then it seems reasonable to say that we have gotten quite close to answering Turing’s question whether machines can think. As we use chatbots, language models are becoming extensions of our language, and our language is becoming a extension of language models. Computers and devices are already a part of our thinking. Our thinking is a part of our computers and devices. Turing’s question whether machines can think is closely related with the question whether people can think.
Our daily life depends on computers. Children learn to speak the language of tablet menus together with their native tongues. Networks absorb our thoughts, reprocess them, and feed them back to us, suitably rearranged. We absorb information, reprocess it, and feed it back to computers. This extended mind processes data by linking human and artificial network nodes. Neither the nodes nor the network can reliably tell them apart. “I think, therefore I exist” may be stated as a hallucination. But this extended mind solves problems that no participant node could solve alone, by methods that are not available to any of them, and by nonlocal methods. Its functioning engenders nonlocal forms of awareness and attention. Language engines (they call themselves AIs) are built as a convenience for human customers, and their machine intelligence is meant to be a convenient extension of human intelligence. But the universality of the underlying computation means that the machine intelligence subsumes the human thinking, and vice versa. The universality of language makes intelligence invariant under implementations and decries the idea of artificiality as artificial.
Machines cannot think without people and people cannot think without machines — just like musicians cannot play symphonies without instruments, and the instruments cannot play them without the musicians. People have, of course, invented machine-only music, and voice-only music, mainly as opportunities to sell plastic beads and pearls, and to impose prohibitions. They will surely build markets that sell chatbot-only stories and churches that prohibit chatbots. But that has nothing to do with thinking or intelligence. That’s just stuff people do to each other.
If the past of search engines says anything about the future of language engines, then the main goal of chatbots will soon be to convince you to give your money to the engine’s owner’s sponsors. The new mind will soon be for sale. The goal of this course is to spell out an analytic framework to question its sanity and to explore the possibilities, the needs, and the means to restore it.
Overview: Layers of language (and of the course)
Lecture 1: Syntax
- grammars
- syntactic types and pregroups
Lecture 2: Semantics
- static semantics: vector space model and concept analysis
- dynamic semantics: n-grams, channels, dependent types
Lecture 3: Learning
- neural networks and gradient descent
- transformers and attention
- deep learning and associations
- beyond pretraining and hallucinations
Lecture 4: Universal search
- effective induction and algorithmic probability
- compression and algorithmic complexity
- effective pregroups
- noncommutative geometry of meaning
(The elephants in the room will be described in the next part.)
Who are chatbots
(and what are they to you)? was originally published in Towards Data Science on Medium, where people are continuing the conversation by highlighting and responding to this story.
Language processing in humans and computers: Part 1
Who are chatbots
(and what are they to you)?
Introduction
Chatbots: Shifting the paradigm of meaning
What just happened?
We live in strange times.
Stories used to be told by storytellers, poems recited by poets, music played by musicians, science taught by teachers. Then the printing and recording technologies made copying possible and the copyright got invented and the owners of the recording and printing equipment started earning more than musicians and storytellers. Then the web happened and it all came under your fingertips. Now chatbots happened and you can ask them to write poetry or to explain science, or even combine the two.
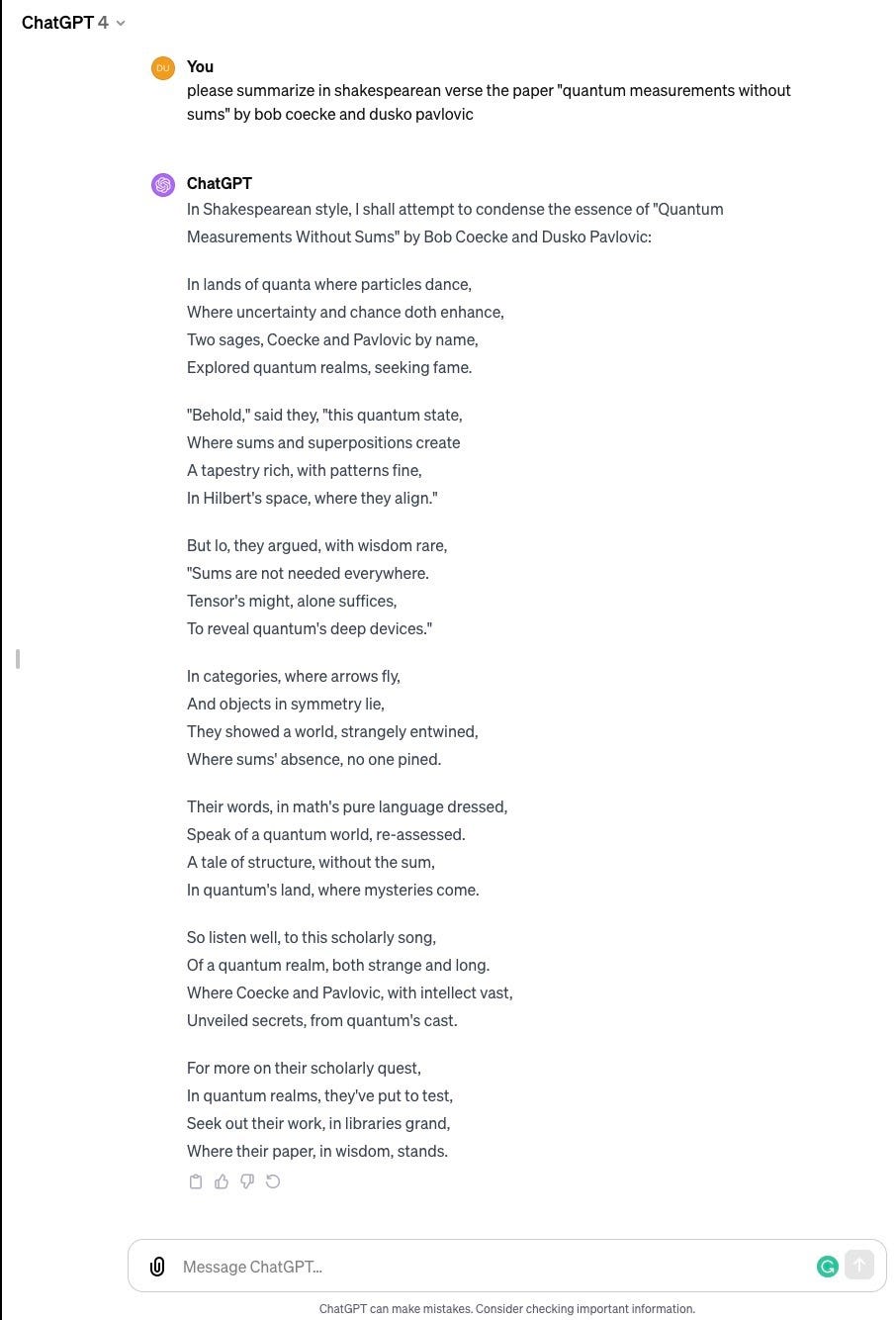
They even seem to have sparks of a sense of humor. I asked the same bot to translate a subtle metaphor from Croatian to English and she did it so well that I got an impulse to thank her in French, with “merci :)” to which she pivoted to German: “Gern geschehen! If you have any more questions, feel free to ask. ☺️”
The paradigm of meaning
Our interactions with computers evolved gradually. They have been able to maintain light conversations since the 1960s, and personal assistants conversing in human voices have been sold for a while. Not a big deal. Human appearances are in the eyes of human observers.
But with chatbots, the appearances seem to have gone beyond the eyes. When you and I chat, we assume that the same words mean the same things because we have seen the same world. If we talk about chairs, we can point to a chair. The word “chair” refers to a thing in the world. But a chatbot has never seen a chair, or anything else. For a chatbot, a word cannot possibly refer to a thing in the world, since it has no access to the world. It appears to know what it is talking about because it was trained on data downloaded from the web, which were uploaded by people who know what they are talking about. A chatbot has never seen a chair, or a tree, or a cow, or felt pain, but his chats are remixes of the chats between people who have. Chatbot’s words do not refer to things directly, but indirectly, through people’s words. The next image illustrates how this works.

When I say “cow’’, it is because I have a cow on my mind. When a chatbot says “cow”, it is because that word is a likely completion of the preceding context. Many theories of meaning have been developed in different parts of linguistics and philosophy, often subsumed under the common name semiotics. While they differ in many things, they mostly stick with the picture on the left, of meaning as a relation between a signifying token, say a written or spoken word, and a signified item, an object, or a concept. While many semioticists noted that words and other fragments of language are also referred to by words and other fragments of language, the fact that the entire process of language production can be realized within a self-contained system of references, as a whirlwind of words arising from words — as demonstrated by chatbots — is a fundamental, unforeseen challenge. According to most theories of meaning, chatbots should be impossible. Yet here they are!
Zeno and the aliens
A chatbot familiar with pre-Socratic philosophy would be tempted to compare the conundrum of meaning with the conundrum of motion, illustrated in DALL-E’s picture of Parmenides and Zeno.
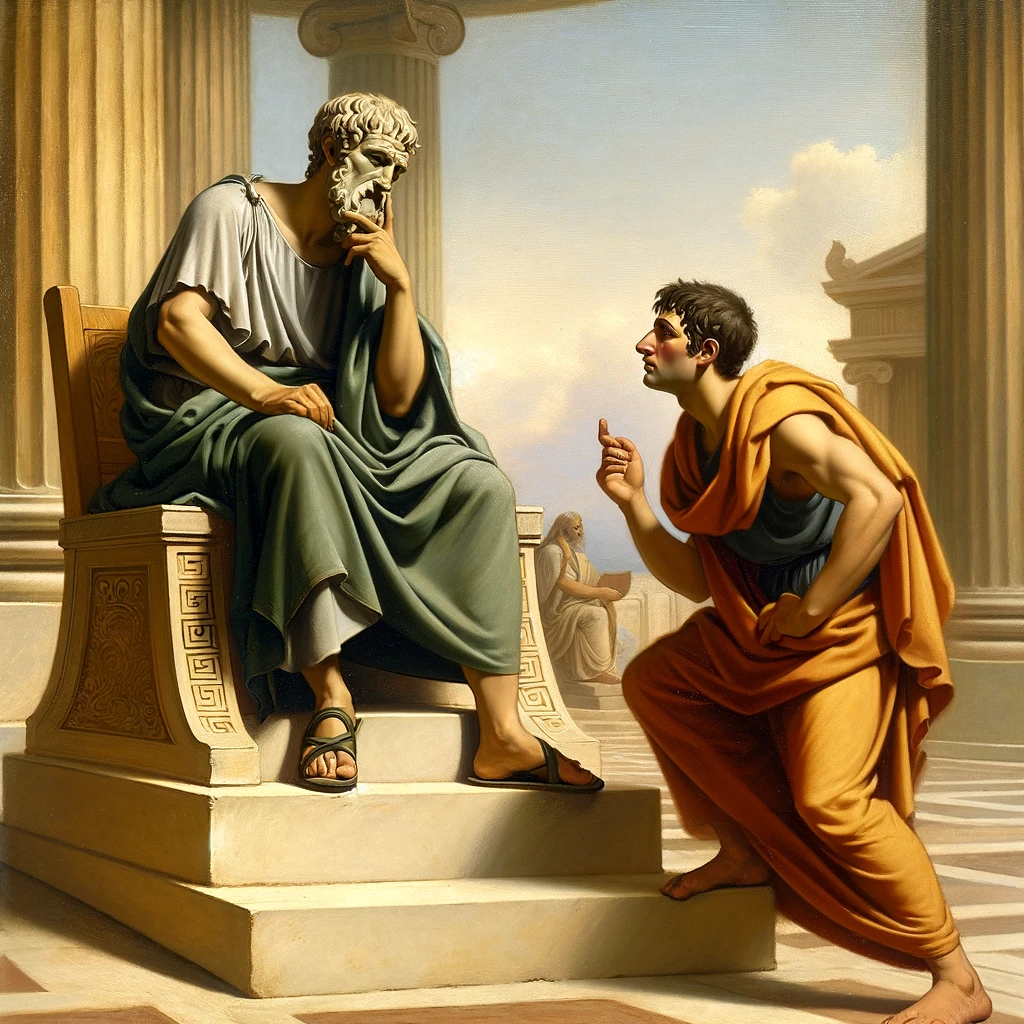
Parmenides was a leading Greek philosopher in the VI — V centuries BCE. Zeno was his most prominent pupil. To a modern eye, the illustration seems to be showing Zeno disproving Parmenides’ claims against the possibility of movement by moving around. An empiric counterexample. For all we know, Zeno did not intend to disprove his teacher’s claims and neither Parmenides nor Plato (who presented Parmenides’ philosophy in eponymous dialogue) seem to have noticed the tension between Parmenides’ denial of the possibility of movement and Zeno’s actual movement. Philosophy and the students pacing around were not viewed in the same realm. (Ironically, when the laws of motion were finally understood some 2000 years later, Parmenides’ argument, popularized in Zeno’s story about Achilles and tortoise, played an important role.)
Before you dismiss concerns about words and things and Zeno and the chatbots as a philosophical conundrum of no consequence for our projects in science and engineering, note that the self-contained language models, built and trained by chatbot researchers and their companies, could just as well be built and trained by an alien spaceship parked by the Moon. They could listen in, crawl our the websites, scrub the data, build neural networks, train them to speak on our favorite topics in perfect English, provide compelling explanations, illustrate them in vivid colors. Your friendly AI could be operated by product engineers in San Francisco, or in Pyongyang, or on the Moon. They don’t need to understand the chats to build the chatbots. That is not science fiction.
But a broad range of sci-fi scenarios opens up. There was a movie where the landing on the Moon was staged on Earth. Maybe the Moon landing was real but the last World Cup final was modified by AI. Or maybe it wasn’t modified but the losing team can easily prove that it could have been, and the winning team would have more trouble to prove that it wasn’t. Conspiracy theorists are, of course, mostly easy to recognize, but there is an underlying logical property of conspiracies worth taking note of: A large family of false statement generators are one-way functions: most of their false statements are much harder to disprove than to generate.
Without pursuing the interleaving threads of AI science and fiction very far, it seems clear that the boundaries between science and fiction, and between fiction and reality, may have been breached in ways unseen before. We live in strange times.
AI: How did we get here and where do we go?
The mind-body problem and solution
The idea of machine intelligence goes back to Alan Turing, the mathematician who defined and described the processes of computation that surround us. At the age of 19, Alan confronted the problem of mind — where it comes from and where it goes? — when he suddenly lost a friend with whom he had just fallen in love.
Some 300 years earlier, philosopher René Descartes was pondering about the human body. One of the first steps into modern science was his realization that living organisms were driven by the same physical mechanisms as the rest of nature, i.e. that they were in essence similar to the machines built at the time. One thing that he couldn’t figure out was how the human body gives rise to the human mind. He stated that as the mind-body problem.
Alan Turing essentially solved the mind-body problem. His description of computation as a process implementable in machines, and his results proving that that process can simulate our reasoning and calculations, suggested that the mind may be arising from the body as a system of computational processes. He speculated that some version of computation was implemented in our neurons. He illustrated his 1947 research report by a neural network.
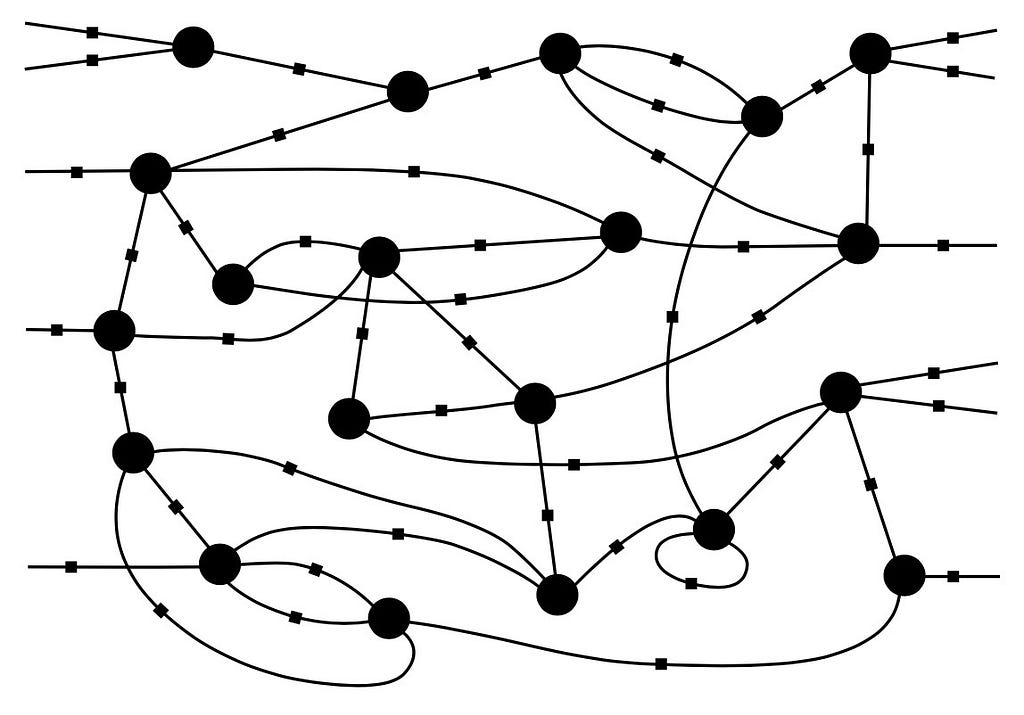
Since such computational processes are implementable in machines, it was reasonable to expect that they could give rise to machine intelligence. Turing spent several years on a futile effort to build one of the first computers, mostly driven by thoughts about the potential of intelligent machinery, and about its consequences.
Turing and Darwin
During WWII, Turing’s theoretical research was superseded by cryptanalysis at Bletchley Park. That part of the story seems well-known. When the war ended, he turned down positions at Cambridge and Princeton and accepted work at the National Physics Laboratory, hoping to build a computer and test the idea of machine intelligence. The 1947 memo contains what seems to be the first emergence of the ideas of training neural networks, and of supervised and unsupervised learning. It was so far ahead of its time that some parts seem still ahead. It was submitted to the director of the National Physics Laboratory Sir Charles G. Darwin, a grandson of Charles Darwin and a prominent eugenicist on his own account. In Sir Darwin’s opinion, Turing’s memo read like a “fanciful school-boy’s essay”. Also resenting Turing’s “smudgy’’ appearance, Sir Darwin smothered the computer project by putting it under strict administrative control. The machine intelligence memo sank into oblivion for more than 20 years. Turing devoted the final years until his death (at the age of 42, by biting into a cyanide-laced apple!) to exploring the computational aspects of life. E.g., what determines the shape of the black spots on a white cow’s hide? Life always interested him.
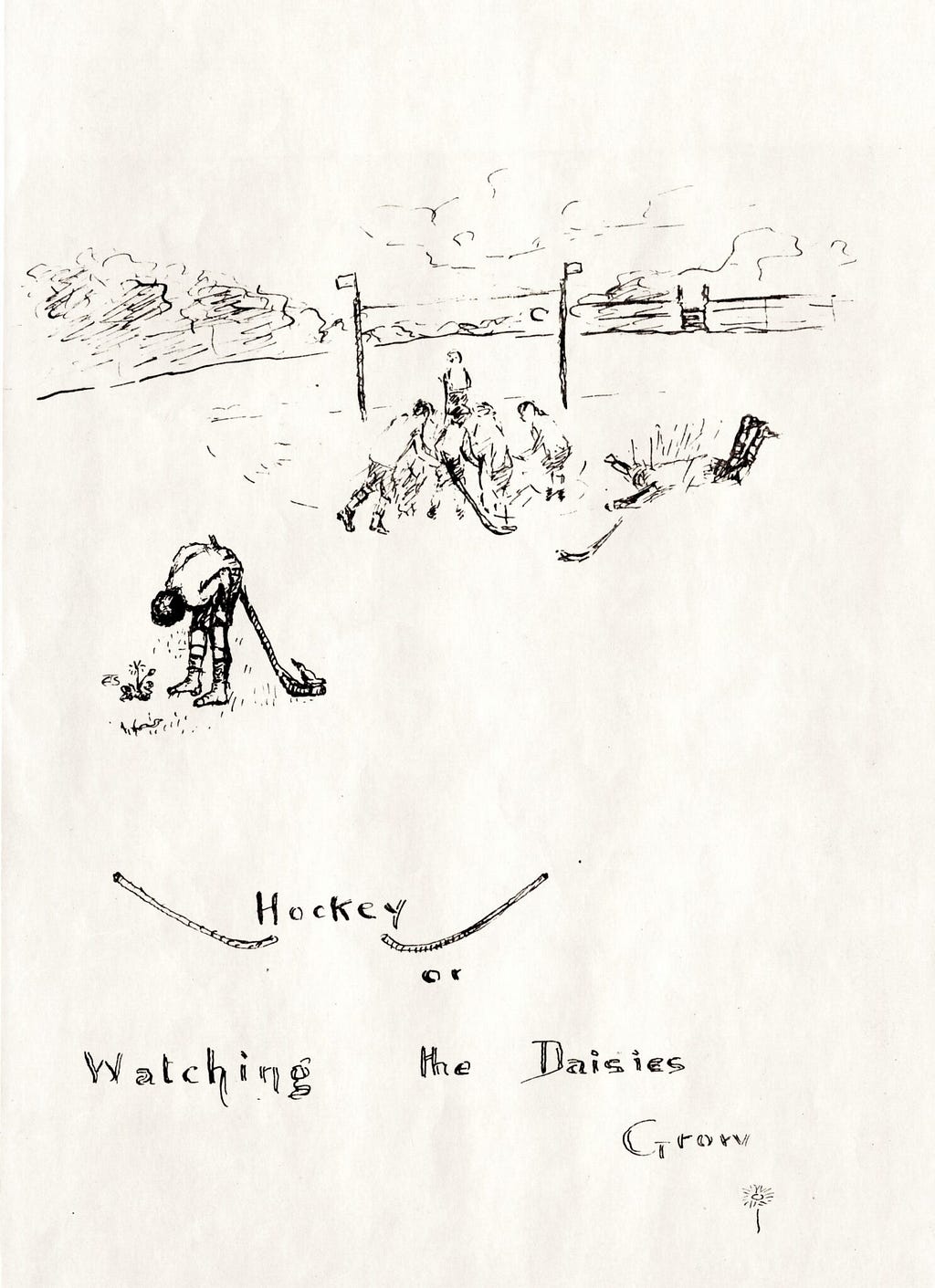
2 years after Turing’s death (9 years after the Intelligent Machinery memo), Turing’s machine intelligence got renamed to Artificial Intelligence (AI) at the legendary Dartmouth workshop.
The history of artificial intelligence is mostly a history of efforts towards intelligent design of intelligence. The main research efforts were to logically reconstruct phenomena of human behavior, like affects, emotions, common sense, etc.; and to realize them in software.
Turing, in contrast, was assuming machine intelligence would evolve spontaneously. The main published account of his thoughts on the topic appeared in the journal “Mind”. The paper opens with the question: “Can machines think?”. What we now call the Turing Test is offered as a means for deciding the answer. The idea is that a machine that can maintain a conversation and remain indistinguishable from a thinking human being must be recognized as a thinking machine. At this time of confusion around chatbots, the closing paragraph of the “Mind” paper seems particularly interesting:
An important feature of a learning machine is that its teacher will often be very largely ignorant of quite what is going on inside, although he may still be able to some extent to predict his pupil’s behavior. […] This is in clear contrast with a normal procedure when using a machine to do computations: one’s object is then to have a clear mental picture of the state of the machine at each moment in the computation. This object can only be achieved with a struggle. The view that `the machine can only do what we know how to order it to do’, appears strange in the face of this fact. Intelligent behaviour presumably consists in a departure from the completely disciplined behaviour.
Designers and builders of chatbots and language engines published many accounts of their systems’ methods and architectures but seem as stumped as everyone by their behaviors. Some initially denied the unexpected behaviors, but then stopped working and started talking about a threat to humanity. Turing anticipated the unexpected behaviors. His broader message seems to be that not knowing what is on the mind of another intelligent entity is not a bug but a feature of intelligence. That is why intelligent entities communicate. Failing that, they view each other as an object. Understanding chatbots may require broadening our moral horizons.
From search engines to language models
One thing that Turing didn’t get right was how intelligent machines would learn to reason. He imagined that they would need to learn from teachers. He didn’t predict the Web. The Web provided the space to upload the human mind. The language models behind chatbots are
- not a result of intelligent design of artificial intelligence,
- but an effect of spontaneous evolution of the Web.
Like search engines, language models are fed data scraped from the Web by crawlers. A search engine builds an index to serve links based on rankings (pulled from data or pushed by sponsors), whereas a language engine builds a model to predict continuations of text based on context references. The computations evolve, but the proportions that are being computed remain the same:

What does the Web past say about the AI future?
The space of language, computation, networks, and AI has many dimensions. The notion of mind has many definitions. If we take into account that our mind depends on language, computation, networks, and AI as its tools and extensions, just like music depends on instruments, then it seems reasonable to say that we have gotten quite close to answering Turing’s question whether machines can think. As we use chatbots, language models are becoming extensions of our language, and our language is becoming a extension of language models. Computers and devices are already a part of our thinking. Our thinking is a part of our computers and devices. Turing’s question whether machines can think is closely related with the question whether people can think.
Our daily life depends on computers. Children learn to speak the language of tablet menus together with their native tongues. Networks absorb our thoughts, reprocess them, and feed them back to us, suitably rearranged. We absorb information, reprocess it, and feed it back to computers. This extended mind processes data by linking human and artificial network nodes. Neither the nodes nor the network can reliably tell them apart. “I think, therefore I exist” may be stated as a hallucination. But this extended mind solves problems that no participant node could solve alone, by methods that are not available to any of them, and by nonlocal methods. Its functioning engenders nonlocal forms of awareness and attention. Language engines (they call themselves AIs) are built as a convenience for human customers, and their machine intelligence is meant to be a convenient extension of human intelligence. But the universality of the underlying computation means that the machine intelligence subsumes the human thinking, and vice versa. The universality of language makes intelligence invariant under implementations and decries the idea of artificiality as artificial.
Machines cannot think without people and people cannot think without machines — just like musicians cannot play symphonies without instruments, and the instruments cannot play them without the musicians. People have, of course, invented machine-only music, and voice-only music, mainly as opportunities to sell plastic beads and pearls, and to impose prohibitions. They will surely build markets that sell chatbot-only stories and churches that prohibit chatbots. But that has nothing to do with thinking or intelligence. That’s just stuff people do to each other.
If the past of search engines says anything about the future of language engines, then the main goal of chatbots will soon be to convince you to give your money to the engine’s owner’s sponsors. The new mind will soon be for sale. The goal of this course is to spell out an analytic framework to question its sanity and to explore the possibilities, the needs, and the means to restore it.
Overview: Layers of language (and of the course)
Lecture 1: Syntax
- grammars
- syntactic types and pregroups
Lecture 2: Semantics
- static semantics: vector space model and concept analysis
- dynamic semantics: n-grams, channels, dependent types
Lecture 3: Learning
- neural networks and gradient descent
- transformers and attention
- deep learning and associations
- beyond pretraining and hallucinations
Lecture 4: Universal search
- effective induction and algorithmic probability
- compression and algorithmic complexity
- effective pregroups
- noncommutative geometry of meaning
(The elephants in the room will be described in the next part.)
Who are chatbots
(and what are they to you)? was originally published in Towards Data Science on Medium, where people are continuing the conversation by highlighting and responding to this story.